Re-imaginig the Networks: Detecting Local Communities in Networks by Approximating Derivatives in Graph Space.
ASONAM '17: Advances in Social Networks Analysis and Mining 2017 Sydney Australia July, 2017(2017)
摘要
Finding communities in networks has become very important because various social and physical complex systems are represented as networks. Because of their ability to address large networks, local community detection methods that start from a seed node have become the focus of many research endeavours in recent times. In this paper, a derivative-based local community detection method, inspired by active contours, is proposed for finding a community surrounding an initial seed. The method is based on the concepts of curvature and gradient of the current communitys boundary. Curvature and gradient comprise the velocity function used to determine whether the boundary should move to include a candidate node in its vicinity. Unlike Euclidean space, networks have non-uniform space with fluctuations of dimensionality, given by the fluctuation in degrees of nodes, which complicates the calculation of derivatives used to define the curvature and gradient of boundary nodes. In this research, with some intuitive imagination, a framework for approximating derivatives in network space is proposed. Benchmarking this community detection method against three contemporary methods shows that it is capable of building communities with equal or better conductance.
更多查看译文
关键词
graph space,seed node,nonuniform space,boundary nodes,network space,derivative-based local community detection
AI 理解论文
溯源树
样例
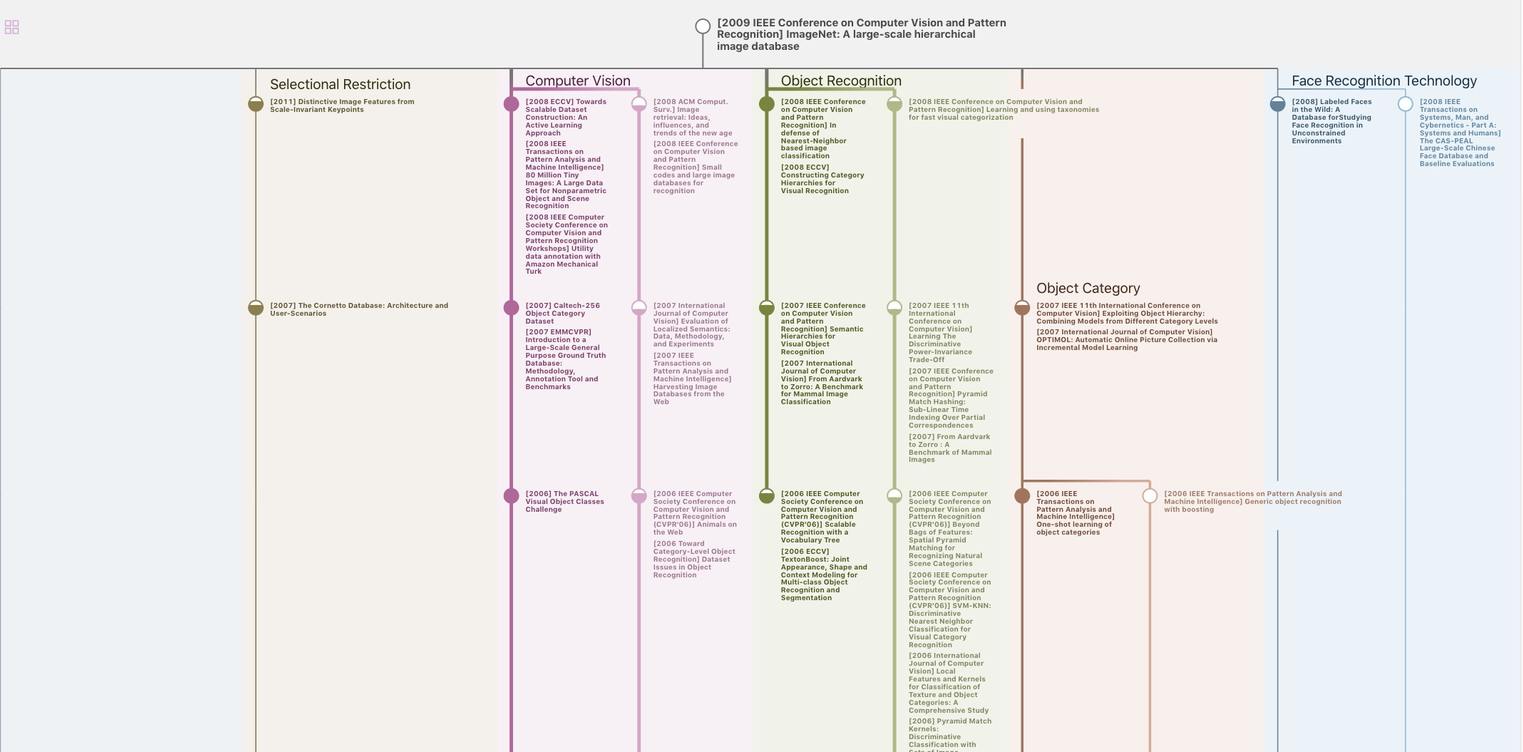
生成溯源树,研究论文发展脉络
Chat Paper
正在生成论文摘要