Gnmf Revisited: Joint Robust K-Nn Graph And Reconstruction-Based Graph Regularization For Image Clustering
ARTIFICIAL NEURAL NETWORKS AND MACHINE LEARNING, PT II(2017)
摘要
Clustering has long been a popular topic in machine learning and is the basic task of many vision applications. Graph regularized NMF (GNMF) and its variants as extensions of NMF decompose the whole dataset as the product of two low-rank matrices which respectively indicate centroids of clusters and cluster memberships for each sample. Although they utilize graph structure to reveal the geometrical structure within datasets, these methods completely ignore the robustness of graph structure. To address the issue above, this paper jointly incorporates a novel Robust Graph and Reconstruction-based Graph regularization into NMF (RG(2)NMF) to promote the gain in clustering performance. Particularly, RG(2)NMF stabilizes the objective of GNMF through the reconstruction regularization, and meanwhile exploits a learning procedure to derive the robust graph. Experiments of image clustering on two popular datasets illustrate the effectiveness of RG(2)NMF compared with the baseline methods in quantities.
更多查看译文
关键词
k-NN graph, Graph regularization, Nonnegative matrix factorization, Clustering
AI 理解论文
溯源树
样例
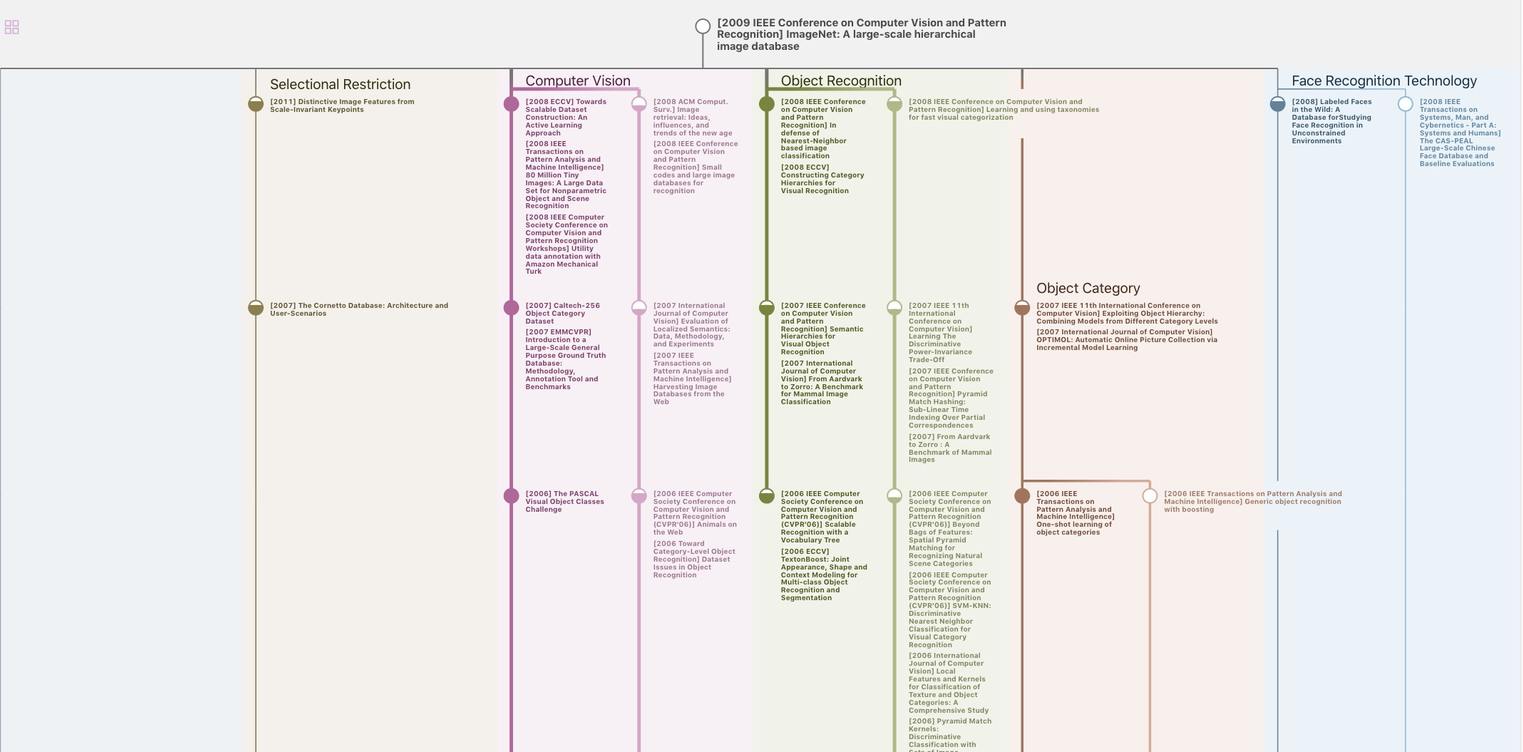
生成溯源树,研究论文发展脉络
Chat Paper
正在生成论文摘要