Sleep Apnea Event Detection From Nasal Airflow Using Convolutional Neural Networks
NEURAL INFORMATION PROCESSING, ICONIP 2017, PT V(2017)
摘要
Obstructive sleep apnea-hypopnea syndrome is a respiratory disorder characterized by abnormal breathing patterns during sleep. It causes problems during sleep, including loud snoring and frequent awaking. This study proposes a new approach for the detection of apnea-hypopnea events from the raw signal data of nasal airflow using convolutional neural networks. Convolutional neural networks are a prominent type of deep neural networks known for their ability to automatically learn features from high dimensional data without manual feature engineering. We demonstrate the applicability of this technique on a dataset of 24,480 samples (30 s long) extracted from nasal flow signals of 100 subjects in the MESA sleep study. The performance of the convolutional neural network model is compared with another approach that uses a support vector machine model with statistical features generated from the flow signal. Our results show that the convolutional neural network outperformed the support vector machine approach, achieving accuracy and F1-score of 75%.
更多查看译文
关键词
Sleep apnea detection, Convolutional neural networks, Support vector machines
AI 理解论文
溯源树
样例
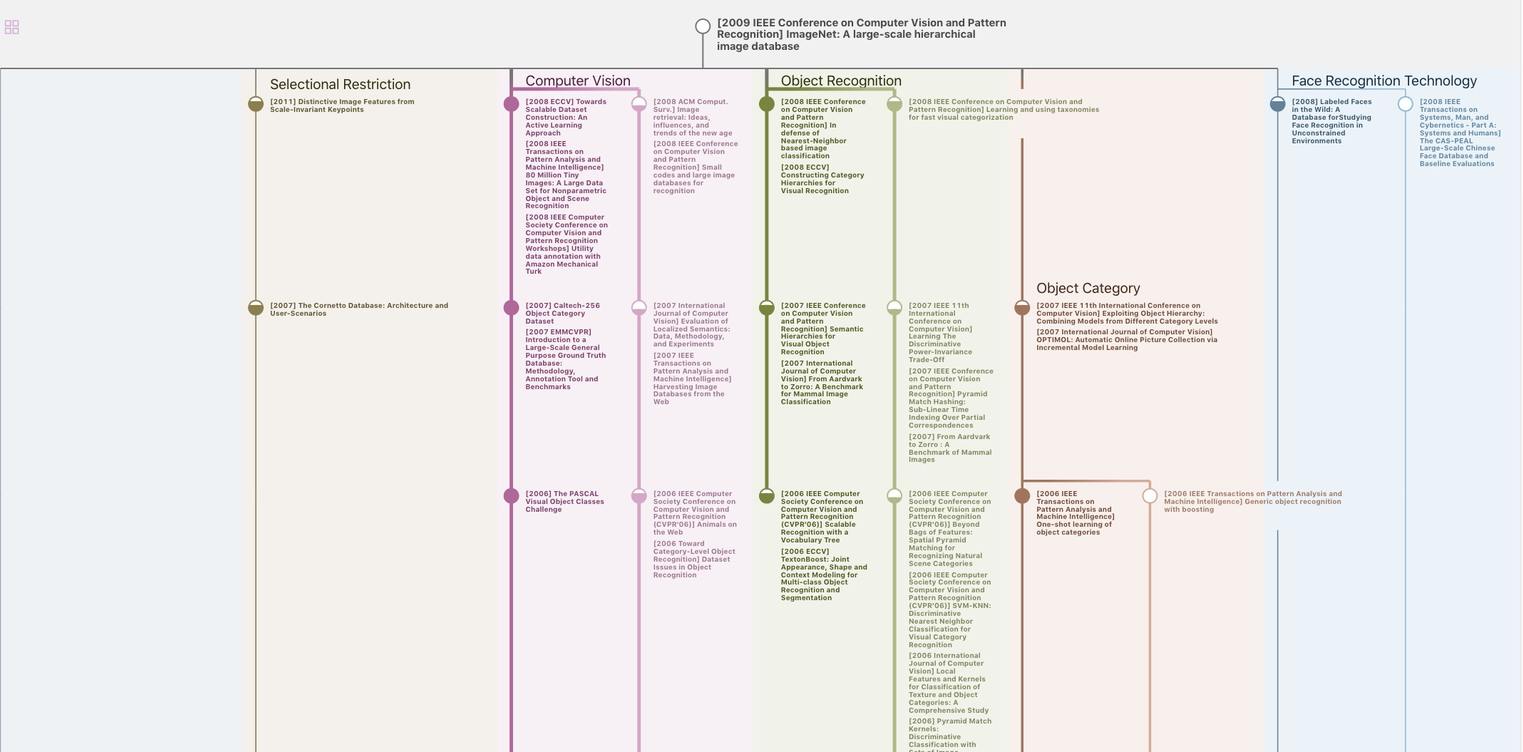
生成溯源树,研究论文发展脉络
Chat Paper
正在生成论文摘要