Temporal Attention Neural Network For Video Understanding
NEURAL INFORMATION PROCESSING (ICONIP 2017), PT II(2017)
摘要
Deep learning based vision understanding algorithms have recently approached human-level performance in object recognition and image captioning. These performance evaluations are, however, limited to static data and these algorithms are also limited. Few limitations of these methods include their inability to selectively encode human behavior, movement of multiple objects and time-varying variations in the background. To address these limitations and to extend these algorithms for analyzing dynamic videos, we propose a temporal attention CNN-RNN network with motion saliency map. Our proposed model overcome scarcity of usable information in encoded data and efficiently integrate motion features by incorporating dynamic nature of information present in successive frames. We evaluate our proposed model over UCF101 public dataset and our experiments demonstrate that our proposed model successfully extract motion information for video understanding without any computationally intensive preprocessing.
更多查看译文
关键词
Video understanding,Action recognition,Saliency map,Convolutional neural network,Long short term memory,Deep learning
AI 理解论文
溯源树
样例
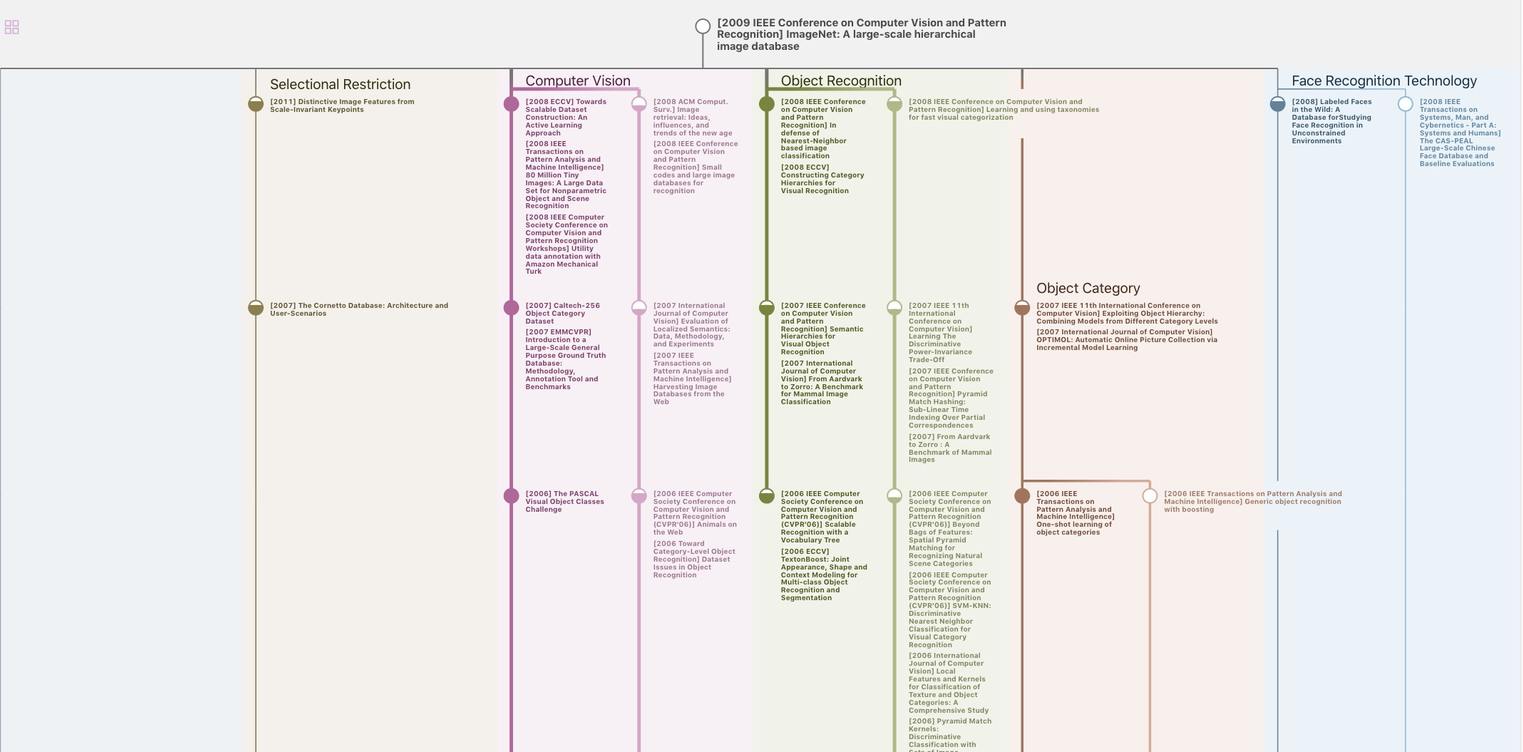
生成溯源树,研究论文发展脉络
Chat Paper
正在生成论文摘要