Face Hallucination And Recognition Using Kernel Canonical Correlation Analysis
NEURAL INFORMATION PROCESSING (ICONIP 2017), PT VI(2017)
摘要
Canonical correlation analysis (CCA) is a classical but powerful tool for image super-resolution tasks. Since CCA in essence is a linear projection learning method, it usually fails to uncover the nonlinear relationships between high-resolution (HR) and low-resolution (LR) facial image features. In order to solve this issue, we propose a new face hallucination and recognition algorithm based on kernel CCA, where the nonlinear correlation between HR and LR face features can be well depicted by implicit high-dimensional nonlinear mappings determined by specific kernels. First, our proposed method respectively extracts the principal component features from high-resolution and low-resolution facial images for computational efficiency and noise removal. Then, it makes use of kernel CCA to learn the nonlinear consistency of HR and LR facial features. The proposed approach is compared with existing face hallucination algorithms. A number of experimental results on LR face recognition have demonstrated the effectiveness and robustness of our proposed method.
更多查看译文
关键词
Face hallucination,Kernel CCA,Face recognition
AI 理解论文
溯源树
样例
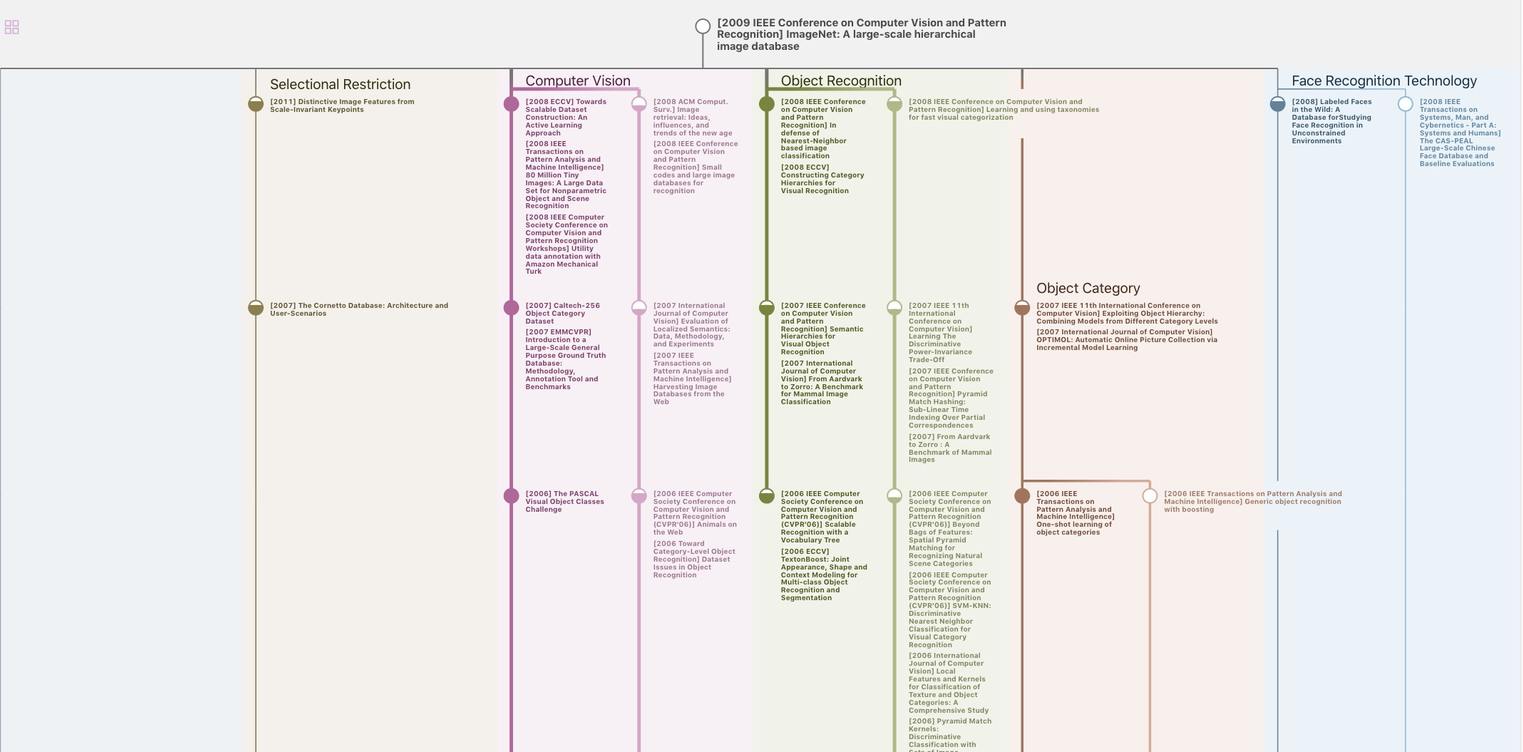
生成溯源树,研究论文发展脉络
Chat Paper
正在生成论文摘要