A Generalized I-Elm Algorithm For Handling Node Noise In Single-Hidden Layer Feedforward Networks
NEURAL INFORMATION PROCESSING, ICONIP 2017, PT I(2017)
摘要
The incremental extreme learning machine (I-ELM) algorithm provides a low computational complexity training mechanism for single-hidden layer feedforward neworks (SLFNs). However, the original I-ELM algorithm does not consider the node noise situation, and node noise may greatly degrade the performance of a trained SLFN. This paper presents a generalized node noise resistant I-ELM (GNNR-I-ELM) for SLFNs. We first define a noise resistant training objective function for SLFNs. Afterwards, we develop the GNNR-I-ELM algorithm which adds tau nodes into the network at each iteration. The GNNR-I-ELM algorithm estimates the output weights of the newly additive nodes and does not change all the previously trained output weights. Its noise tolerant ability is much better than that of the original I-ELM. Besides, we prove that in terms of the training set mean squared error of noisy networks, the GNNR-I-ELM algorithm converges.
更多查看译文
关键词
Multiplicative noise,Additive noise,Feedforward networks
AI 理解论文
溯源树
样例
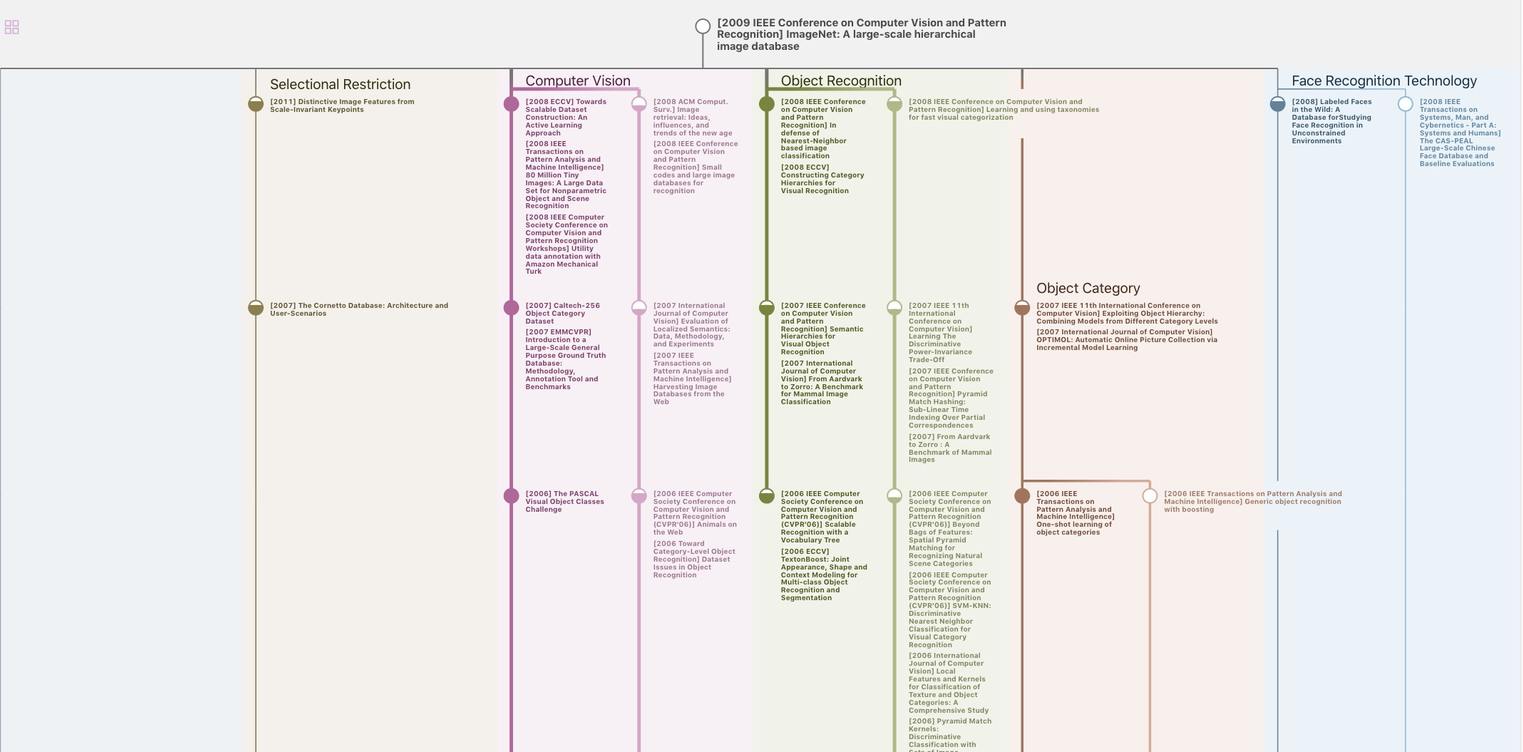
生成溯源树,研究论文发展脉络
Chat Paper
正在生成论文摘要