Stochastic Segmentation Trees For Multiple Ground Truths
CONFERENCE ON UNCERTAINTY IN ARTIFICIAL INTELLIGENCE (UAI2017)(2017)
摘要
A strong machine learning system should aim to produce the full range of valid interpretations rather than a single mode in tasks involving inherent ambiguity. This is particularly true for image segmentation, in which there are many sensible ways to partition an image into regions. We formulate a tree-structured probabilistic model, the stochastic segmentation tree, that represents a distribution over segmentations of a given image. We train this model by optimizing a novel objective that quantifies the degree of match between statistics of the model and ground truth segmentations. Our method allows learning of both the parameters in the tree and the structure itself. We demonstrate on two datasets, including the challenging Berkeley Segmentation Dataset, that our model is able to successfully capture the range of ground truths and to produce novel plausible segmentations beyond those found in the data.
更多查看译文
AI 理解论文
溯源树
样例
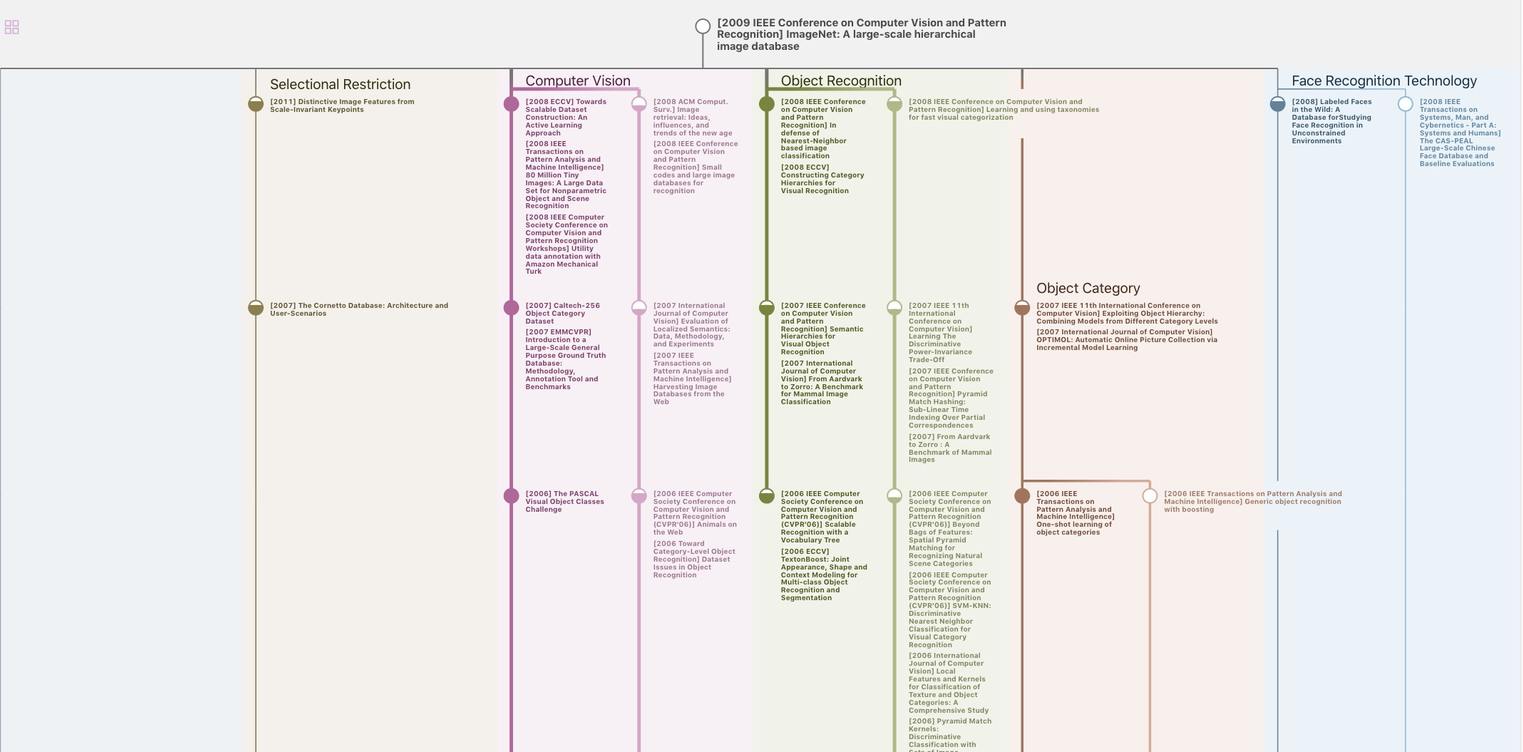
生成溯源树,研究论文发展脉络
Chat Paper
正在生成论文摘要