Privacy Preserving Visualization For Social Network Data With Ontology Information
2017 IEEE PACIFIC VISUALIZATION SYMPOSIUM (PACIFICVIS)(2017)
摘要
Analyzing social network data helps sociologists understand the behaviors of individuals and groups as well as the relationships between them. With additional ontology information, the semantics behind the network structure can be further explored. Unfortunately, creating network visualizations with these datasets for presentation can inadvertently expose the private and sensitive information of individuals that reside in the data. To deal with this problem, we generalize conventional data anonymization models (originally designed for relational data) and formally apply them in the context of privacy preserving ontological network visualization. We use these models to identify the privacy leaks that exist in a visualization, provide graph modification actions that remove and/or perceptually minimize the effect of the identified leaks, and discuss strategies for what types of privacy actions to choose depending on the context of the leaks. We implement an ontological visualization interface with associated privacy preserving operations, and demonstrate with two case studies using real-world datasets to show that our approach can identify and solve potential privacy issues while balancing overall graph readability and utility.
更多查看译文
关键词
social network data analysis,ontology information,individual behavior,network structure,private information,sensitive information,generalize conventional data anonymization models,privacy preserving ontological network visualization,graph modification actions,ontological visualization interface,privacy preserving operations,privacy issues,graph readability,graph utility
AI 理解论文
溯源树
样例
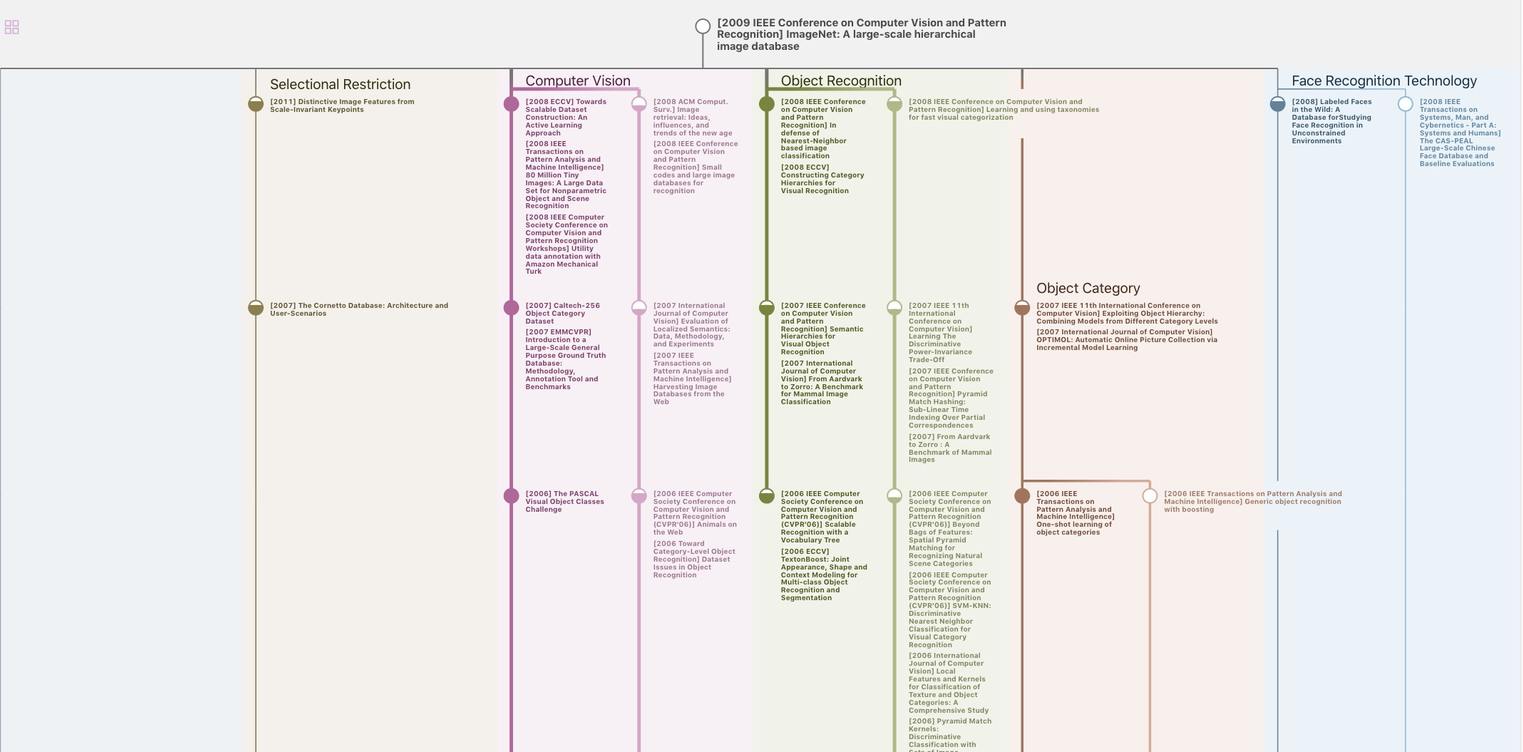
生成溯源树,研究论文发展脉络
Chat Paper
正在生成论文摘要