Product Space Decompositions for Continuous Representations of Brain Connectivity.
Lecture Notes in Computer Science(2017)
摘要
We develop a method for the decomposition of structural brain connectivity estimates into locally coherent components, leveraging a non-parametric Bayesian hierarchical mixture model with tangent Gaussian components. This model provides a mechanism to share information across subjects while still including explicit mixture distributions of connections for each subject. It further uses mixture components defined directly on the surface of the brain, eschewing the usual graph-theoretic framework of structural connectivity in favor of a continuous model that avoids a priori assumptions of parcellation configuration. The results of two experiments on a test-retest dataset are presented, to validate the method. We also provide an example analysis of the components.
更多查看译文
关键词
Brain connectivity,Non-parametric bayes,Unsupervised learning
AI 理解论文
溯源树
样例
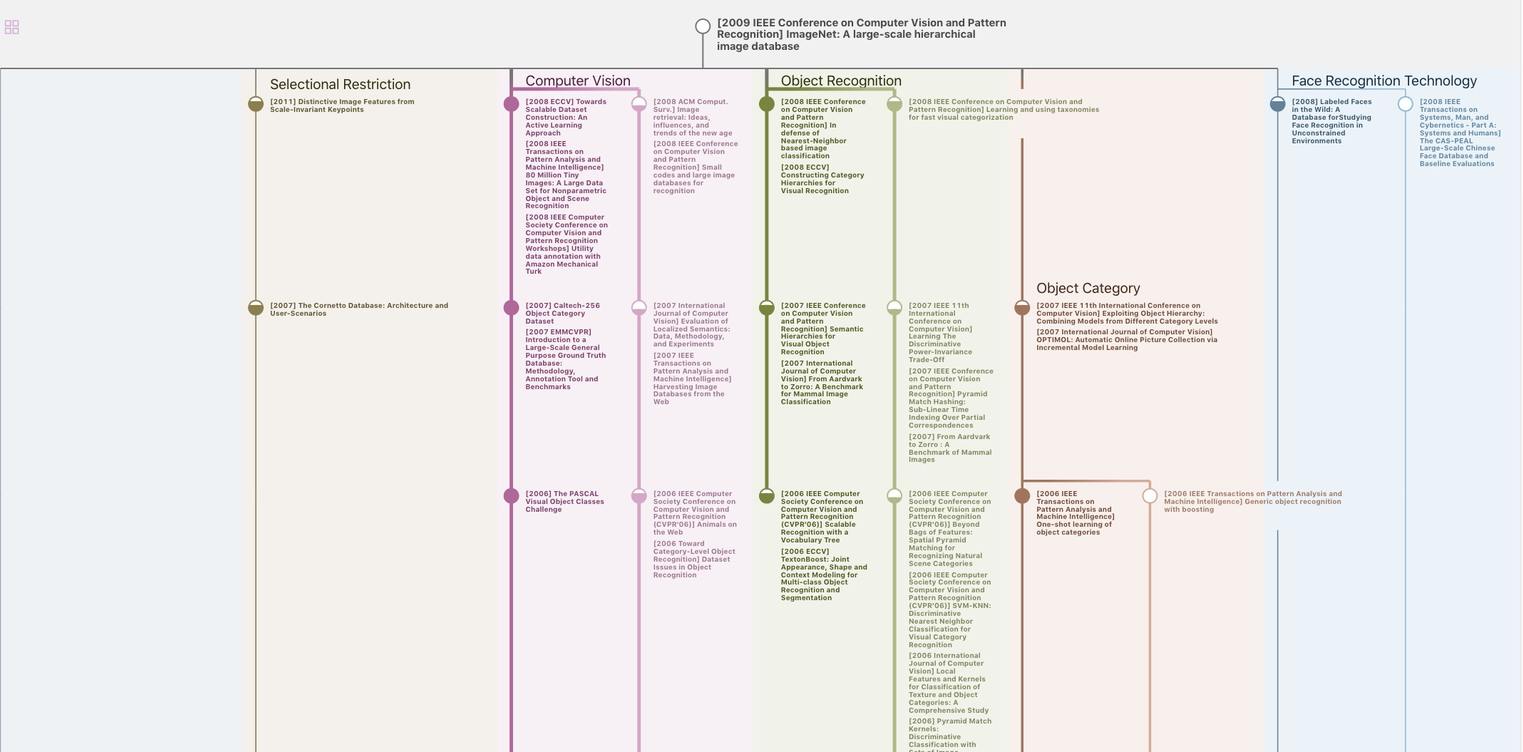
生成溯源树,研究论文发展脉络
Chat Paper
正在生成论文摘要