Globally-Optimal Anatomical Tree Extraction from 3D Medical Images Using Pictorial Structures and Minimal Paths.
MICCAI(2017)
摘要
Extracting centerlines of anatomical trees (e.g., vasculature and airways) from 3D medical images is a crucial preliminary step for various medical applications. We propose an automatic tree extraction method that leverages prior knowledge of tree topology and geometry and ensures globally-optimal solutions. We define a pictorial structure with a corresponding cost function to detect tree bifurcations in anatomical trees with predefined topology. The tree bifurcations are encoded as nodes in the pictorial structure and are associated with an artificial neural network (ANN) based unary term. The geometrical (direction and length) statistics of tree branches are learned from a training set and encoded as geometrical priors for regularizing the pictorial structure edges. Finally, detected bifurcations as well as the ANN tubularity scores, are leveraged to trace globally optimal minimal paths along 3D tree centrelines. Our method outperforms competing state-of-the-art when evaluated on 3D synthesized vasculature and lung airways in CT and our results demonstrate the advantages of incorporating tree statistics and global optimization for this task.
更多查看译文
关键词
Tree-like, Pictorial structure, Geometrical prior, Bifurcation detection, Centerline extraction, Global optimization
AI 理解论文
溯源树
样例
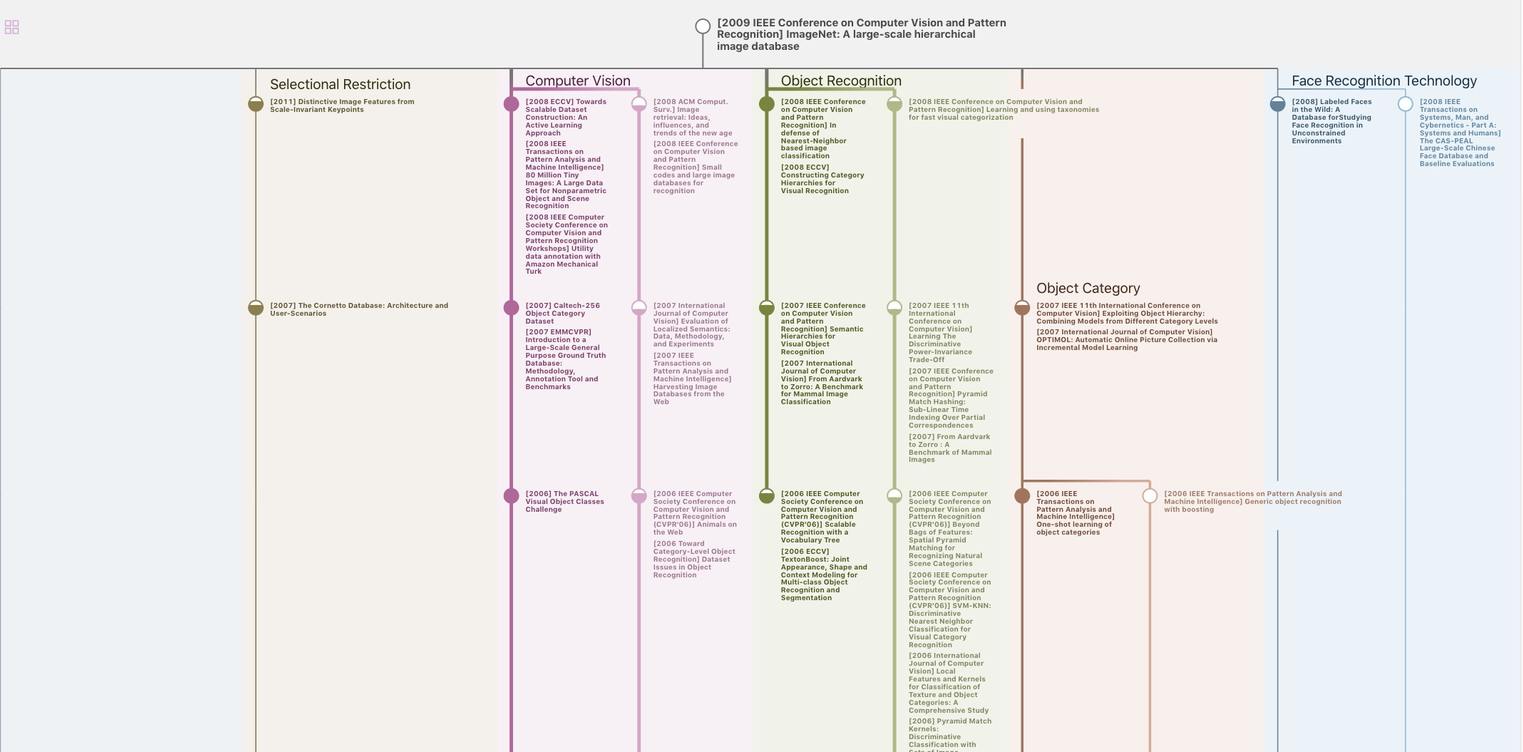
生成溯源树,研究论文发展脉络
Chat Paper
正在生成论文摘要