Context-Based Normalization Of Histological Stains Using Deep Convolutional Features
DEEP LEARNING IN MEDICAL IMAGE ANALYSIS AND MULTIMODAL LEARNING FOR CLINICAL DECISION SUPPORT(2017)
摘要
While human observers are able to cope with variations in color and appearance of histological stains, digital pathology algorithms commonly require a well-normalized setting to achieve peak performance, especially when a limited amount of labeled data is available. This work provides a fully automated, end-to-end learning-based setup for normalizing histological stains, which considers the texture context of the tissue. We introduce Feature Aware Normalization, which extends the framework of batch normalization in combination with gating elements from Long Short-Term Memory units for normalization among different spatial regions of interest. By incorporating a pretrained deep neural network as a feature extractor steering a pixelwise processing pipeline, we achieve excellent normalization results and ensure a consistent representation of color and texture. The evaluation comprises a comparison of color histogram deviations, structural similarity and measures the color volume obtained by the different methods.
更多查看译文
关键词
Normalization, Machine learning, Deep learning, Histology
AI 理解论文
溯源树
样例
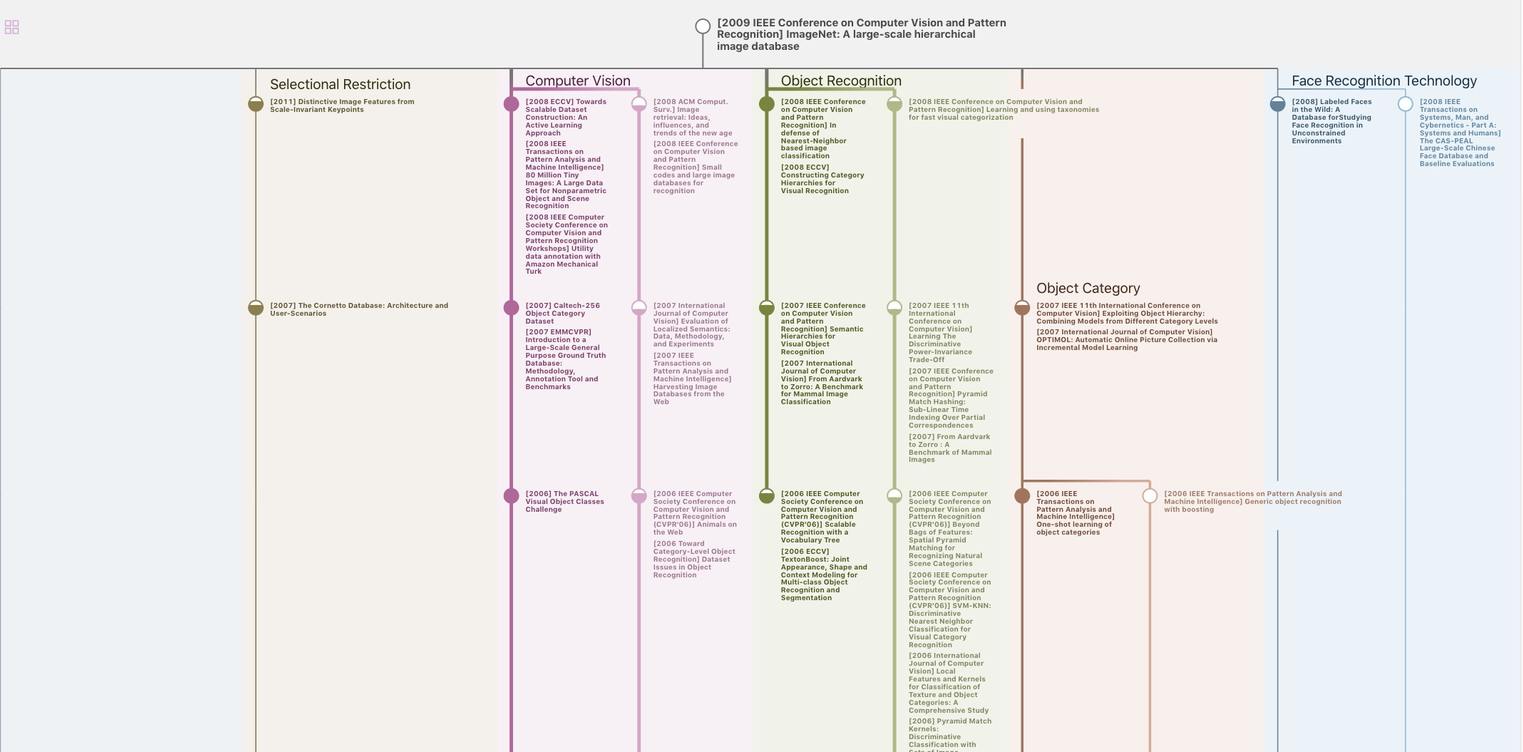
生成溯源树,研究论文发展脉络
Chat Paper
正在生成论文摘要