Improved Neonatal Seizure Detection Using Adaptive Learning
2017 39TH ANNUAL INTERNATIONAL CONFERENCE OF THE IEEE ENGINEERING IN MEDICINE AND BIOLOGY SOCIETY (EMBC)(2017)
摘要
In neonatal intensive care units performing continuous EEG monitoring, there is an unmet need for around-the-clock interpretation of EEG, especially for recognizing seizures. In recent years, a few automated seizure detection algorithms have been proposed. However, these are suboptimal in detecting brief-duration seizures (< 30s), which frequently occur in neonates with severe neurological problems. Recently, a multi-stage neonatal seizure detector, composed of a heuristic and a data-driven classifier was proposed by our group and showed improved detection of brief seizures. In the present work, we propose to add a third stage to the detector in order to use feedback of the Clinical Neurophysiologist and adaptively retune a threshold of the second stage to improve the performance of detection of brief seizures. As a result, the false alarm rate (FAR) of the brief seizure detections decreased by 50% and the positive predictive value (PPV) increased by 18%. At the same time, for all detections, the FAR decreased by 35% and PPV increased by 5% while the good detection rate remained unchanged.
更多查看译文
关键词
Algorithms,Electroencephalography,Heuristics,Humans,Infant, Newborn,Infant, Newborn, Diseases,Seizures
AI 理解论文
溯源树
样例
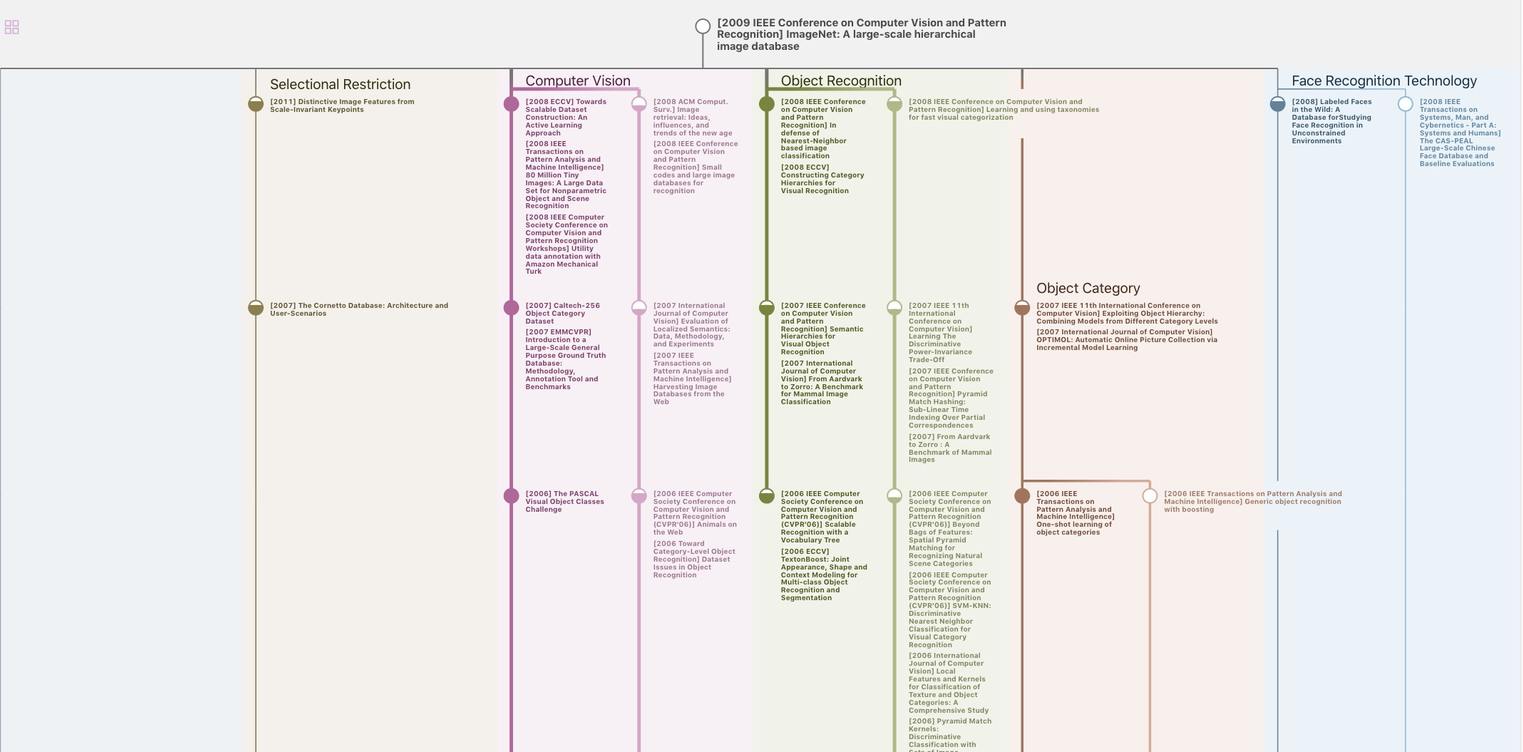
生成溯源树,研究论文发展脉络
Chat Paper
正在生成论文摘要