Multiview and Multimodal Pervasive Indoor Localization.
MM '17: ACM Multimedia Conference Mountain View California USA October, 2017(2017)
摘要
Pervasive indoor localization (PIL) aims to locate an indoor mobile-phone user without any infrastructure assistance. Conventional PIL approaches employ a single probe (i.e., target) measurement to localize by identifying its best match out of a fingerprint gallery. However, a single measurement usually captures limited and inadequate location features. More importantly, the reliance on a single measurement bears the inherent risk of being inaccurate and unreliable, due to the fact that the measurement could be noisy and even corrupted.
In this paper, we address the deficiency of using a single measurement by proposing the original idea of localization based on multi-view and multi-modal measurements. Specifically, a location is represented as a multi-view graph (MVG), which captures both local features and global contexts. We then formulate the location retrieval problem into an MVG matching problem. In MVG matching, a collaborative-reconstruction based measure is proposed to evaluate the node/edge similarity between two MVGs, which can explicitly address noisy measurements or outliers. Extensive experiments have been conducted on three different types of buildings with a total area of 18,719 m^2. We show that even with 30% noisy measurements or outliers, our method is able to achieve a promising accuracy of 1 meter. As another contribution, we construct a benchmark dataset for the PIL task and make it publicly available, which to our knowledge, is the first public dataset that is tailored for multi-view multi-modal indoor localization and contains both magnetic and visual signals.
更多查看译文
关键词
Infrastructure-free, pervasive indoor localization, multiview, multimodal, graph matching
AI 理解论文
溯源树
样例
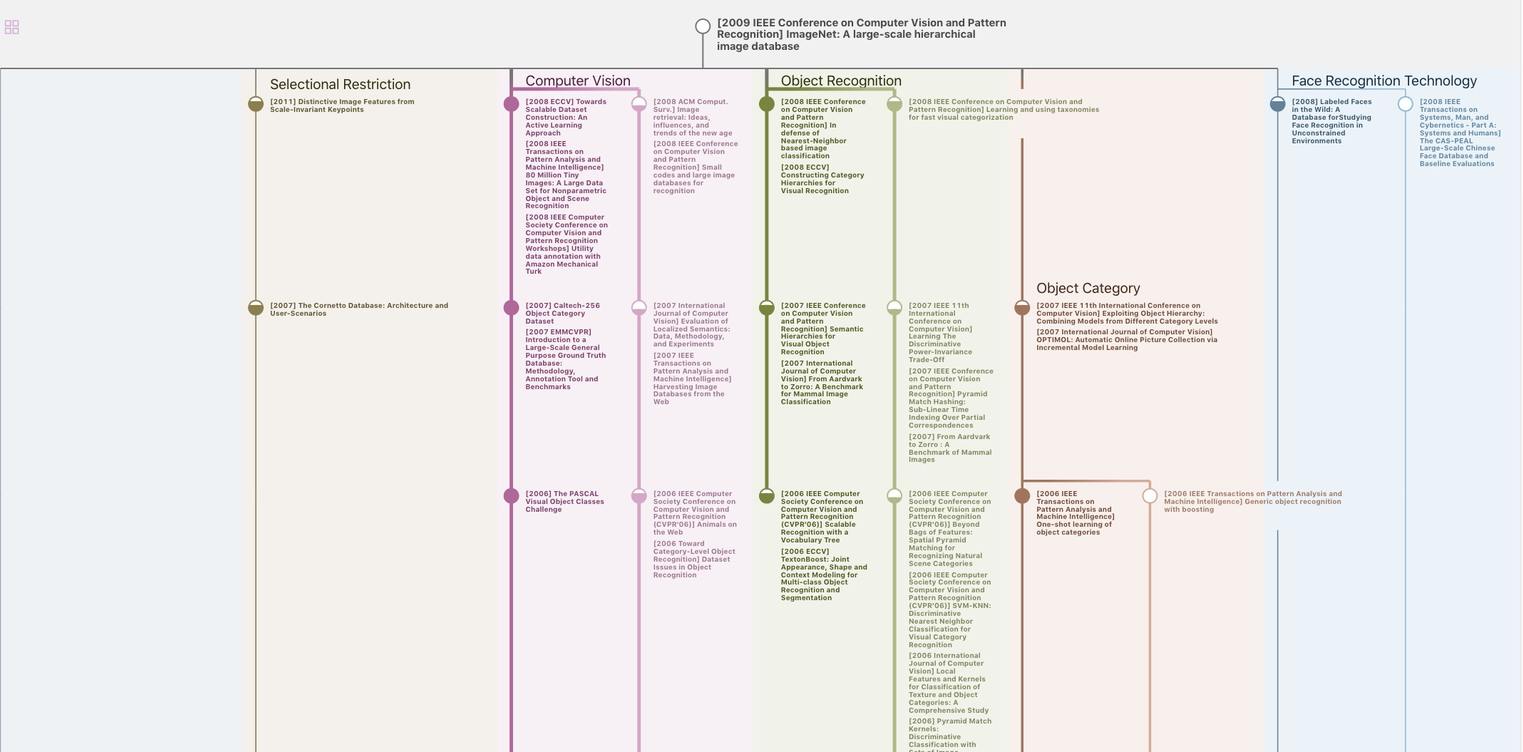
生成溯源树,研究论文发展脉络
Chat Paper
正在生成论文摘要