Integrating Extractive and Abstractive Models for Long Text Summarization
2017 IEEE International Congress on Big Data (BigData Congress)(2017)
摘要
With the explosive growth of information on the Internet, it becomes more and more important to improve the efficiency of information acquisition. Automatic text summarization provides a good means for quick acquisition of information through compression and refinement. While existing methods for automatic text summarization achieve elegant performance on short sequences, however, they are facing the challenges of low efficiency and accuracy when dealing with long text. In this paper, we present a twophase approach towards long text summarization, namely, EA-LTS. In the extraction phase, it conceives a hybrid sentence similarity measure by combining sentence vector and Levenshtein distance, and integrates it into graph model to extract key sentences. In the abstraction phase, it constructs a recurrent neural network based encoder-decoder, and devises pointer and attention mechanisms to generate summaries. We test our model on a real-life long text corpora, collected from sina.com, experimental results verify the accuracy and validity of the proposed method, which is demonstrated to be superior to state-of-the-art methods.
更多查看译文
关键词
automatic text summarization,graph model,recurrent neural network
AI 理解论文
溯源树
样例
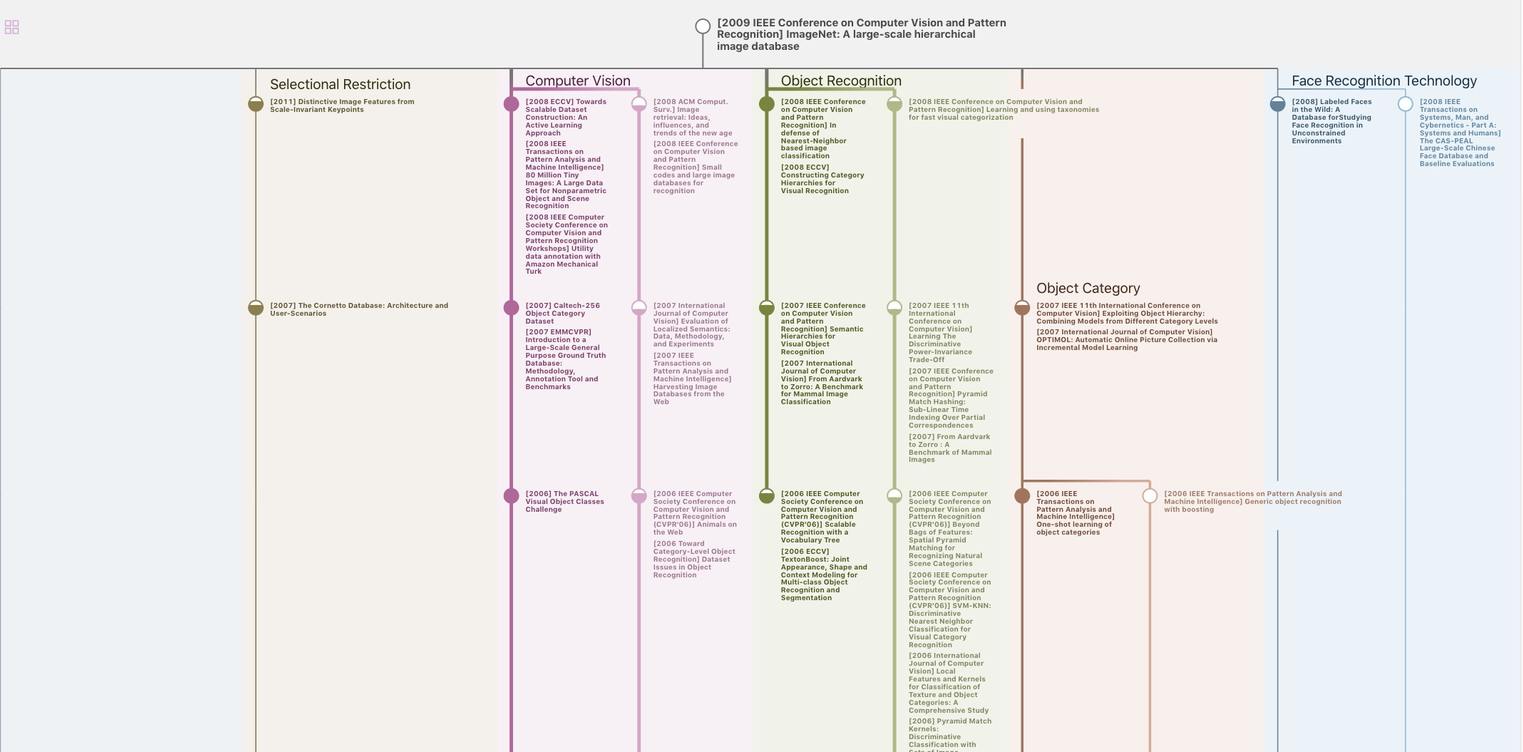
生成溯源树,研究论文发展脉络
Chat Paper
正在生成论文摘要