Classification Of Seizure And Non-Seizure Eeg Signals Based On Emd-Tqwt Method
2017 22ND INTERNATIONAL CONFERENCE ON DIGITAL SIGNAL PROCESSING (DSP)(2017)
摘要
In this work, we have proposed a novel filtering approach based on empirical mode decomposition (EMD) and tunable-Q wavelet transform (TQWT) for the detection of epileptic seizure electroencephalogram (EEG) signals, which is termed as EMD-TQWT method. In this EMD-TQWT method, the intrinsic mode functions (IMFs) obtained from EEG signals using EMD method are considered as a set of amplitude modulated and frequency modulated (AM-FM) components, which are further processed using TQWT method to generate sub-band signals, which can be considered as narrow-band signals. After that, we have measured the information potential (IP) of these obtained sub-band signals using information theory learning based technique. Finally, the IP features are fed to least squares support vector machine (LS-SVM) classifier with radial basis function (RBF) kernel. We have computed the optimal subset of features with feature ranking methods. The proposed approach has been applied on a publicly available EEG database which include healthy, seizure-free, and seizure EEG signals. We have achieved the highest classification accuracy of 99% for classification of seizure and non-seizure EEG signals.
更多查看译文
关键词
Electroencephalogram (EEG) signals, Empirical mode decomposition (EMD), Tunable-Q wavelet transform (TQWT), Information potential (IP), Classifier, Feature ranking
AI 理解论文
溯源树
样例
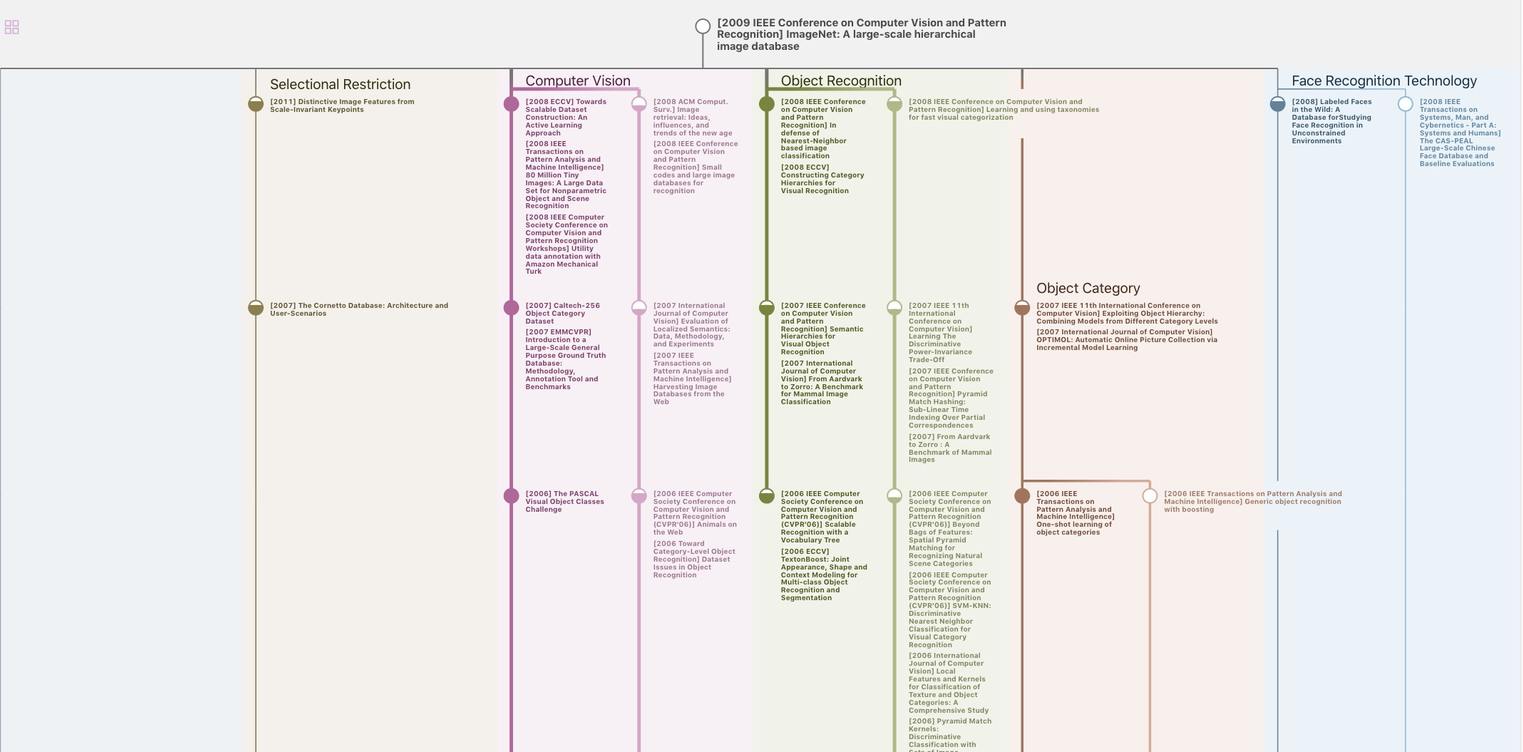
生成溯源树,研究论文发展脉络
Chat Paper
正在生成论文摘要