Improving Individual Predictions Using Social Networks Assortativity
2017 12TH INTERNATIONAL WORKSHOP ON SELF-ORGANIZING MAPS AND LEARNING VECTOR QUANTIZATION, CLUSTERING AND DATA VISUALIZATION (WSOM)(2017)
摘要
Social networks are known to be assortative with respect to many attributes, such as age, weight, wealth, level of education, ethnicity and gender. This can be explained by influences and homophilies. Independently of its origin, this assortativity gives us information about each node given its neighbors. Assortativity can thus be used to improve individual predictions in a broad range of situations, when data are missing or inaccurate. This paper presents a general framework based on probabilistic graphical models to exploit social network structures for improving individual predictions of node attributes. Using this framework, we quantify the assortativity range leading to an accuracy gain in several situations. We finally show how specific characteristics of the network can improve performances further. For instance, the gender assortativity in real-world mobile phone data changes significantly according to some communication attributes. In this case, individual predictions with 75% accuracy are improved by up to 3%.
更多查看译文
关键词
Belief propagation, assortativity, homophily, social networks, mobile phone metadata
AI 理解论文
溯源树
样例
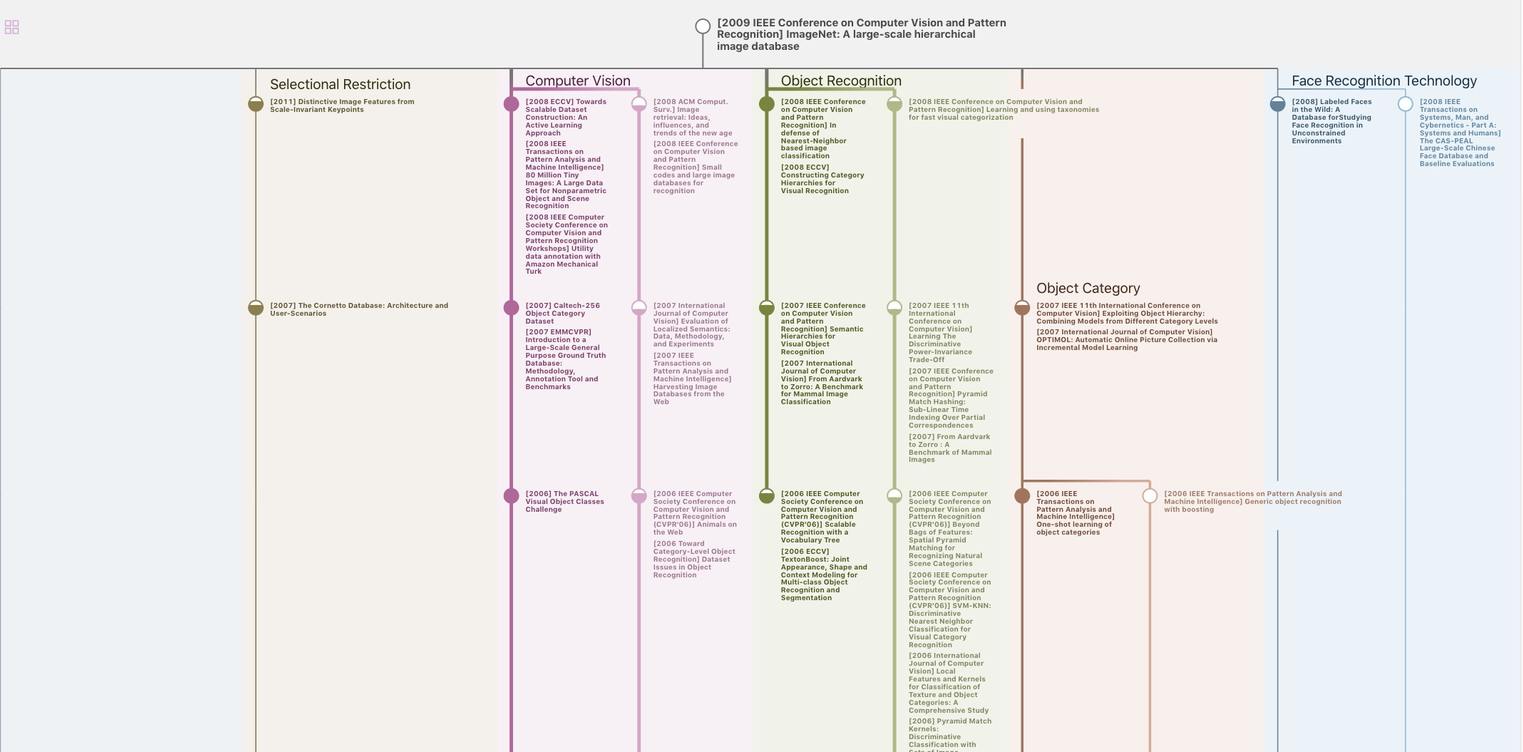
生成溯源树,研究论文发展脉络
Chat Paper
正在生成论文摘要