Shape Representations For Maya Codical Glyphs: Knowledge-Driven Or Deep?
PROCEEDINGS OF THE 15TH INTERNATIONAL WORKSHOP ON CONTENT-BASED MULTIMEDIA INDEXING (CBMI)(2017)
摘要
This paper investigates two-types of shape representations for individual Maya codical glyphs: traditional bag-of-words built on knowledge-driven local shape descriptors (HOOSC), and Convolutional Neural Networks (CNN) based representations, learned from data. For CNN representations, first, we evaluate the activations of typical CNNs that are pretrained on large-scale image datasets; second, we train a CNN from scratch with all the available individual segments. One of the main challenges while training CNNs is the limited amount of available data (and handling data imbalance issue). Here, we attempt to solve this imbalance issue by introducing class-weights into the loss computation during training. Another possibility is oversampling the minority class samples during batch selection. We show that deep representations outperform the other, but CNN training requires special care for small-scale unbalanced data, that is usually the case in the cultural heritage domain.
更多查看译文
关键词
Maya glyphs, convolutional neural networks, shape recognition
AI 理解论文
溯源树
样例
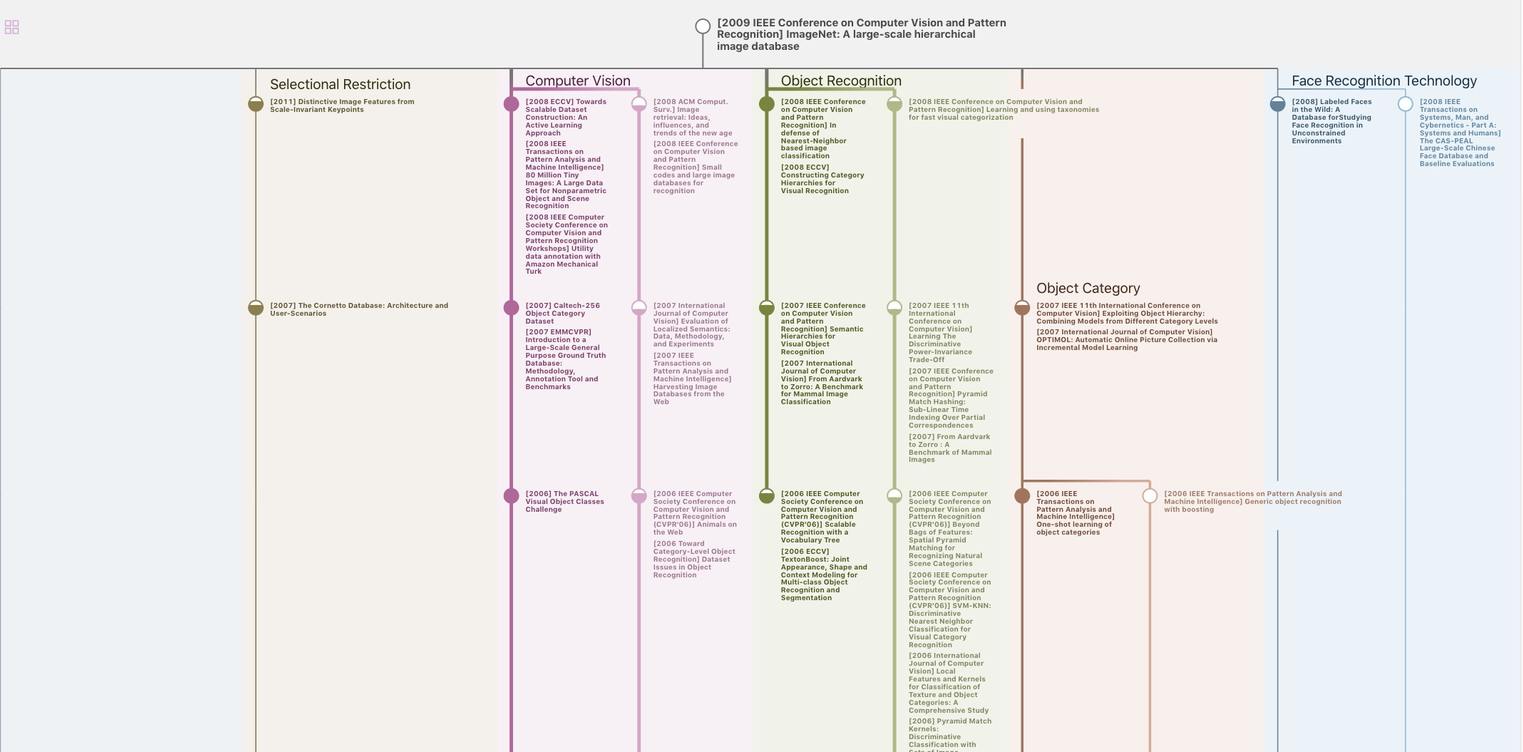
生成溯源树,研究论文发展脉络
Chat Paper
正在生成论文摘要