Latent embeddings for collective activity recognition
2017 14th IEEE International Conference on Advanced Video and Signal Based Surveillance (AVSS)(2017)
摘要
Rather than simply recognizing the action of a person individually, collective activity recognition aims to find out what a group of people is acting in a collective scene. Previous state-of-the-art methods using hand-crafted potentials in conventional graphical model which can only define a limited range of relations. Thus, the complex structural dependencies among individuals involved in a collective scenario cannot be fully modeled. In this paper, we overcome these limitations by embedding latent variables into feature space and learning the feature mapping functions in a deep learning framework. The embeddings of latent variables build a global relation containing person-group interactions and richer contextual information by jointly modeling broader range of individuals. Besides, we assemble attention mechanism during embedding for achieving more compact representations. We evaluate our method on three collective activity datasets, where we contribute a much larger dataset in this work. The proposed model has achieved clearly better performance as compared to the state-of-the-art methods in our experiments.
更多查看译文
关键词
latent embeddings,collective activity recognition,collective scene,latent variables,feature space,feature mapping functions,deep learning framework,collective activity datasets,person-group interactions,contextual information,video clips
AI 理解论文
溯源树
样例
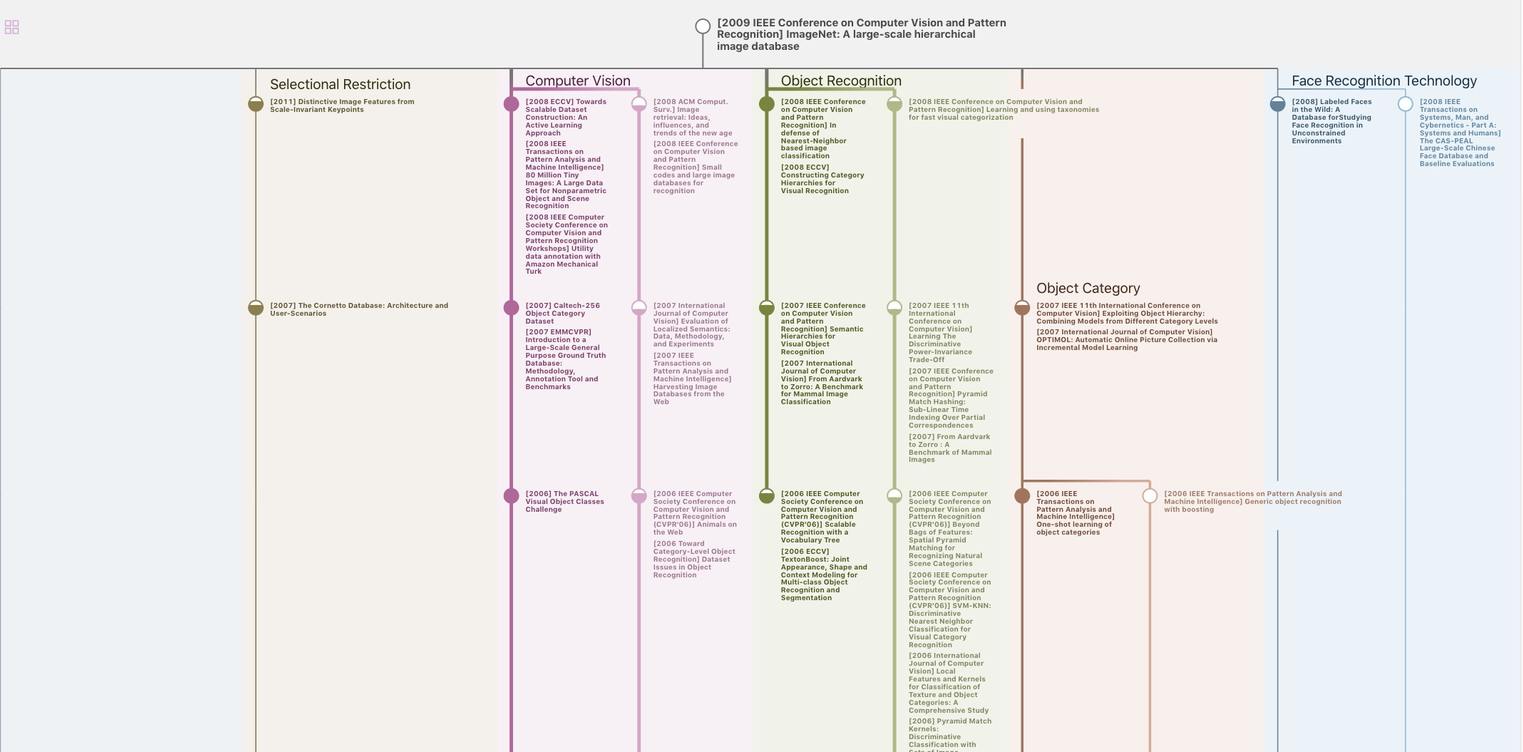
生成溯源树,研究论文发展脉络
Chat Paper
正在生成论文摘要