Human Shape from Silhouettes Using Generative HKS Descriptors and Cross-Modal Neural Networks
30TH IEEE CONFERENCE ON COMPUTER VISION AND PATTERN RECOGNITION (CVPR 2017)(2017)
摘要
In this work, we present a novel method for capturing human body shape from a single scaled silhouette. We combine deep correlated features capturing different 2D views, and embedding spaces based on 3D cues in a novel convolutional neural network (CNN) based architecture. We first train a CNN to find a richer body shape representation space from pose invariant 3D human shape descriptors. Then, we learn a mapping from silhouettes to this representation space, with the help of a novel architecture that exploits correlation of multi-view data during training time, to improve prediction at test time. We extensively validate our results on synthetic and real data, demonstrating significant improvements in accuracy as compared to the state-of-the-art, and providing a practical system for detailed human body measurements from a single image.
更多查看译文
关键词
silhouettes,generative HKS descriptors,cross-modal neural networks,human body shape,deep correlated features,CNN training,convolutional neural network architecture,body shape representation,pose invariant 3D human shape descriptors,Heat Kernel Signature
AI 理解论文
溯源树
样例
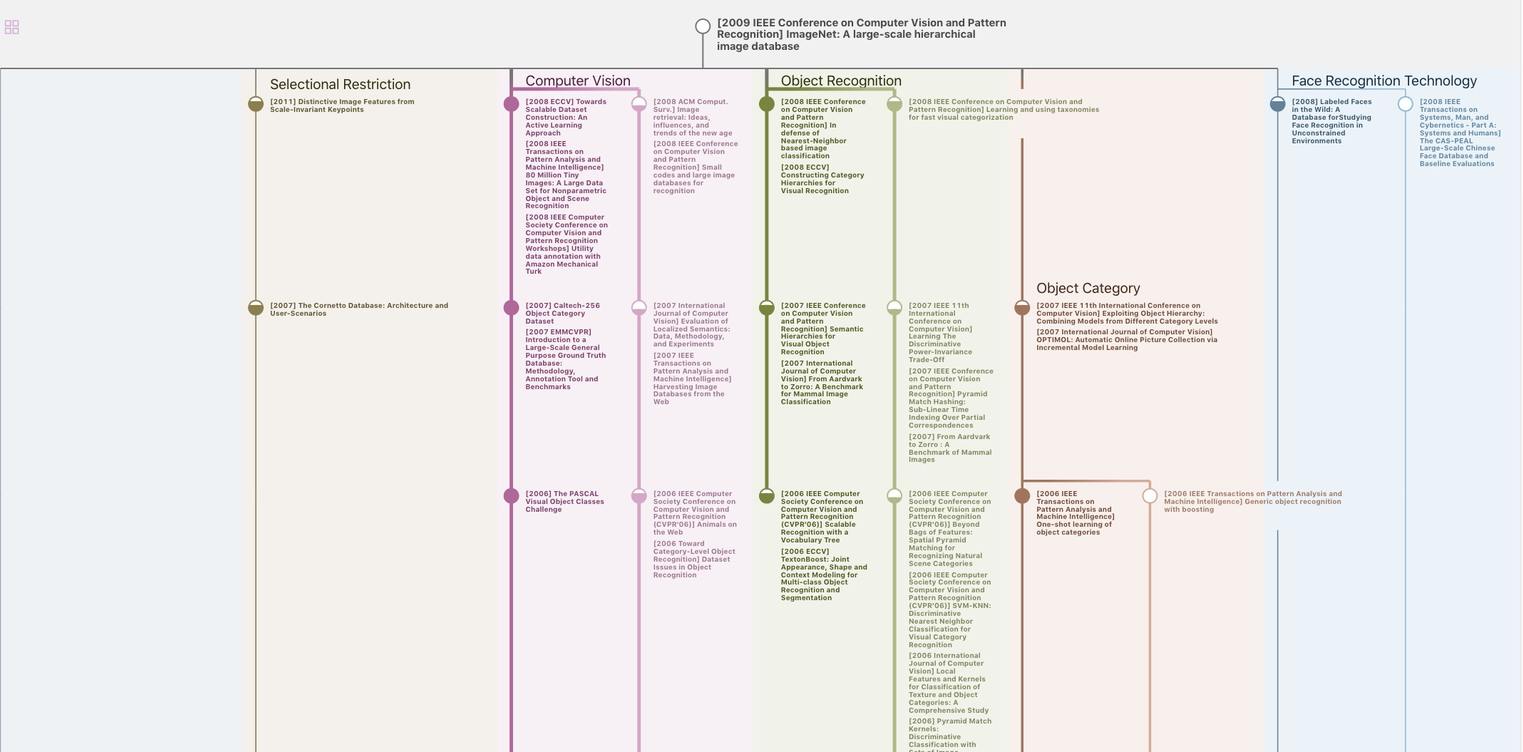
生成溯源树,研究论文发展脉络
Chat Paper
正在生成论文摘要