A Graph Regularized Deep Neural Network For Unsupervised Image Representation Learning
30TH IEEE CONFERENCE ON COMPUTER VISION AND PATTERN RECOGNITION (CVPR 2017)(2017)
摘要
Deep Auto-Encoder (DAE) has shown its promising power in high-level representation learning. From the perspective of manifold learning, we propose a graph regularized deep neural network (GR-DNN) to endue traditional DAEs with the ability of retaining local geometric structure. A deep-structured regularizer is formulated upon multi-layer perceptions to capture this structure. The robust and discriminative embedding space is learned to simultaneously preserve the high-level semantics and the geometric structure within local manifold tangent space. Theoretical analysis presents the close relationship between the proposed graph regularizer and the graph Laplacian regularizer in terms of the optimization objective. We also alleviate the growth of the network complexity by introducing the anchor-based bipartite graph, which guarantees the good scalability for large scale data. The experiments on four datasets show the comparable results of the proposed GR-DNN with the state-of-the-art methods.
更多查看译文
关键词
graph regularized deep neural network,unsupervised image representation learning,high-level representation learning,manifold learning,deep-structured regularizer,robust embedding space,discriminative embedding space,high-level semantics,local manifold tangent space,graph Laplacian regularizer,bipartite graph,deep auto-encoder,local-geometric structure,GR-DNN,DAE
AI 理解论文
溯源树
样例
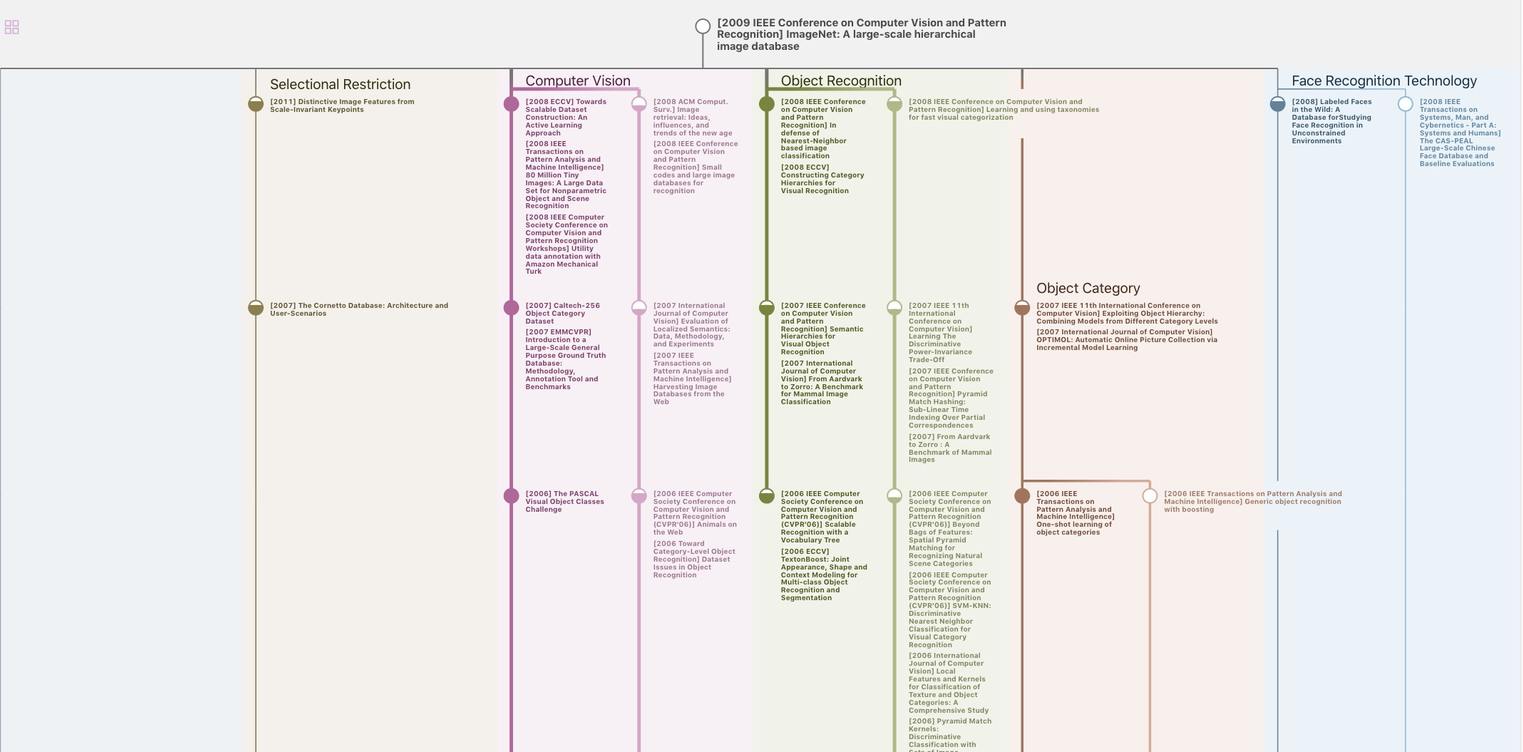
生成溯源树,研究论文发展脉络
Chat Paper
正在生成论文摘要