BranchOut: Regularization for Online Ensemble Tracking with Convolutional Neural Networks
2017 IEEE Conference on Computer Vision and Pattern Recognition (CVPR)(2017)
摘要
We propose an extremely simple but effective regularization technique of convolutional neural networks (CNNs), referred to as BranchOut, for online ensemble tracking. Our algorithm employs a CNN for target representation, which has a common convolutional layers but has multiple branches of fully connected layers. For better regularization, a subset of branches in the CNN are selected randomly for online learning whenever target appearance models need to be updated. Each branch may have a different number of layers to maintain variable abstraction levels of target appearances. BranchOut with multi-level target representation allows us to learn robust target appearance models with diversity and handle various challenges in visual tracking problem effectively. The proposed algorithm is evaluated in standard tracking benchmarks and shows the state-of-the-art performance even without additional pretraining on external tracking sequences.
更多查看译文
关键词
online ensemble tracking,CNN,fully connected layers,variable abstraction levels,BranchOut,multilevel target representation,visual tracking problem,external tracking sequences,convolutional neural networks,convolutional layers,regularization technique,robust target appearance model learning
AI 理解论文
溯源树
样例
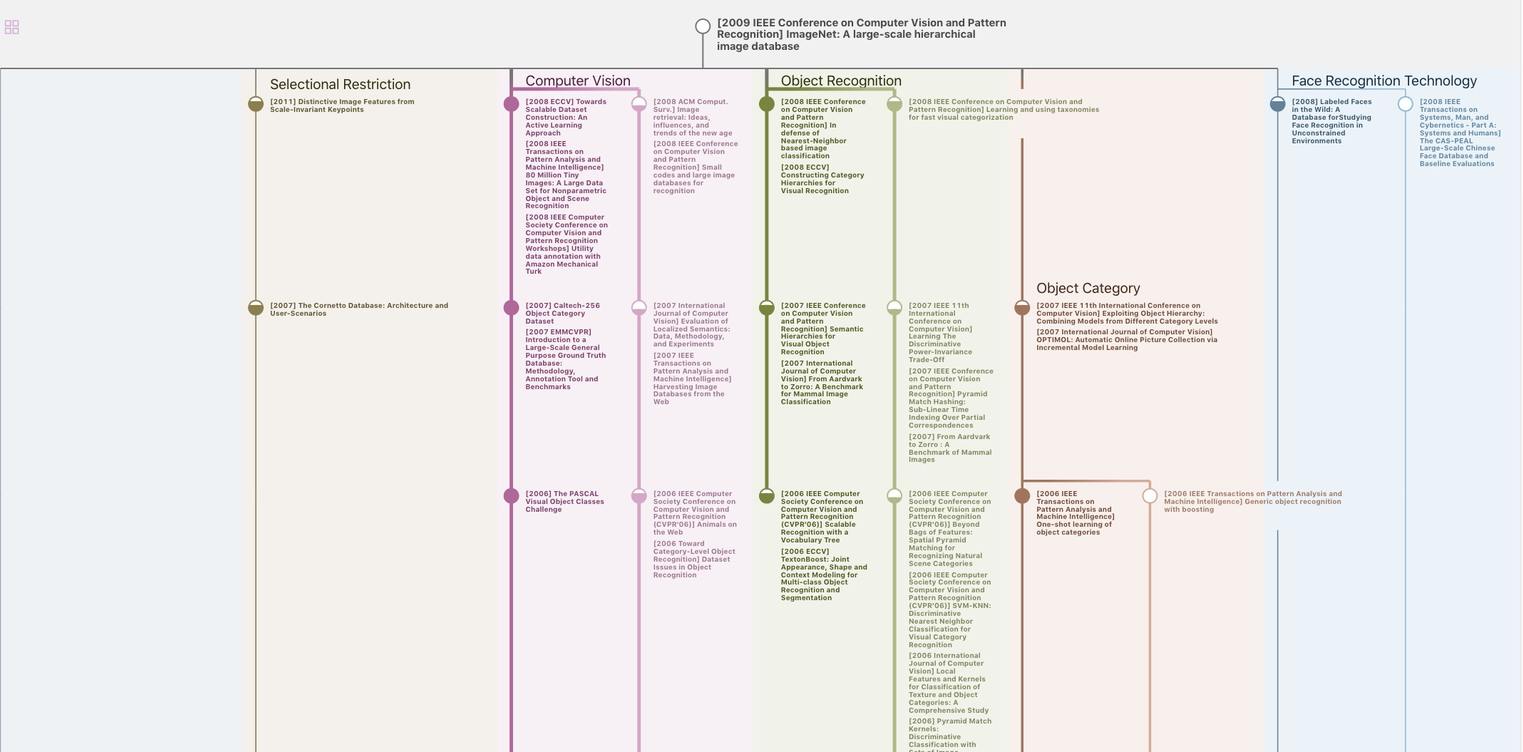
生成溯源树,研究论文发展脉络
Chat Paper
正在生成论文摘要