L2-Net: Deep Learning Of Discriminative Patch Descriptor In Euclidean Space
30TH IEEE CONFERENCE ON COMPUTER VISION AND PATTERN RECOGNITION (CVPR 2017)(2017)
摘要
The research focus of designing local patch descriptors has gradually shifted from handcrafted ones (e.g., SIFT) to learned ones. In this paper, we propose to learn high performance descriptor in Euclidean space via the Convolutional Neural Network (CNN). Our method is distinctive in four aspects: (i) We propose a progressive sampling strategy which enables the network to access billions of training samples in a few epochs. (ii) Derived from the basic concept of local patch matching problem, we emphasize the relative distance between descriptors. (iii) Extra supervision is imposed on the intermediate feature maps. (iv) Compactness of the descriptor is taken into account. The proposed network is named as L2-Net since the output descriptor can be matched in Euclidean space by L-2 distance. L2-Net achieves state-of-the-art performance on the Brown datasets [16], Oxford dataset [18] and the newly proposed Hpatches dataset [11]. The good generalization ability shown by experiments indicates that L2-Net can serve as a direct substitution of the existing handcrafted descriptors. The pre-trained L2-Net is publicly available.
更多查看译文
关键词
euclidean space,Convolutional Neural Network,progressive sampling strategy,training samples,local patch matching problem,relative distance,intermediate feature maps,output descriptor,deep learning,discriminative patch descriptor,local patch descriptors,high performance descriptor,L2-Net
AI 理解论文
溯源树
样例
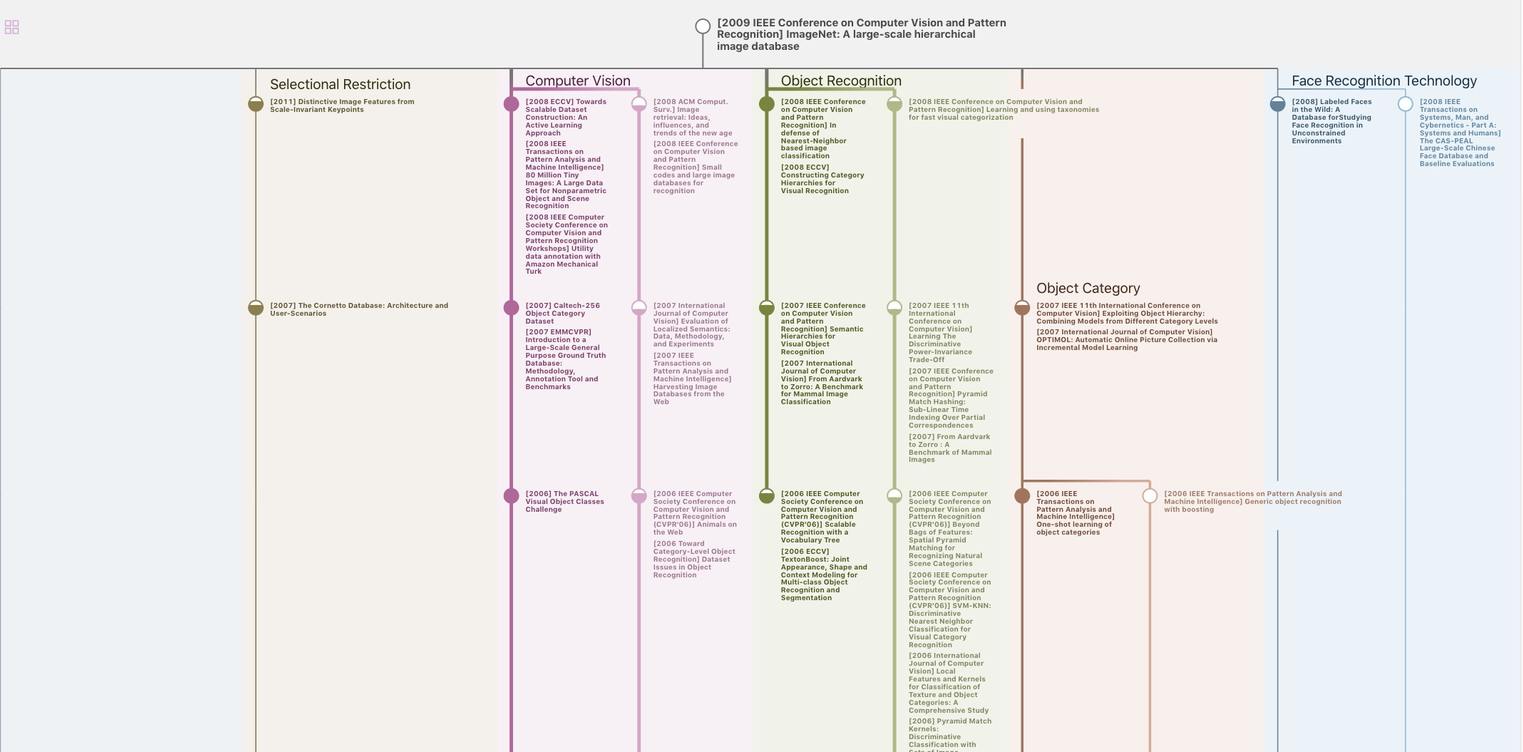
生成溯源树,研究论文发展脉络
Chat Paper
正在生成论文摘要