Generalized Scalar-on-Image Regression Models via Total Variation.
JOURNAL OF THE AMERICAN STATISTICAL ASSOCIATION(2017)
摘要
The use of imaging markers to predict clinical outcomes can have a great impact in public health. The aim of this article is to develop a class of generalized scalar-on-image regression models via total variation (GSIRM-TV), in the sense of generalized linear models, for scalar response and imaging predictor with the presence of scalar covariates. A key novelty of GSIRM-TV is that it is assumed that the slope function (or image) of GSIRM-TV belongs to the space of bounded total variation to explicitly account for the piecewise smooth nature of most imaging data. We develop an efficient penalized total variation optimization to estimate the unknown slope function and other parameters. We also establish nonasymptotic error bounds on the excess risk. These bounds are explicitly specified in terms of sample size, image size, and image smoothness. Our simulations demonstrate a superior performance of GSIRM-TV against many existing approaches. We apply GSIRM-TV to the analysis of hippocampus data obtained from the Alzheimers Disease Neuroimaging Initiative (ADNI) dataset. Supplementary materials for this article are available online.
更多查看译文
关键词
Excess risk,Functional regression,Generalized scalar-on-image regression,Prediction,Total variation
AI 理解论文
溯源树
样例
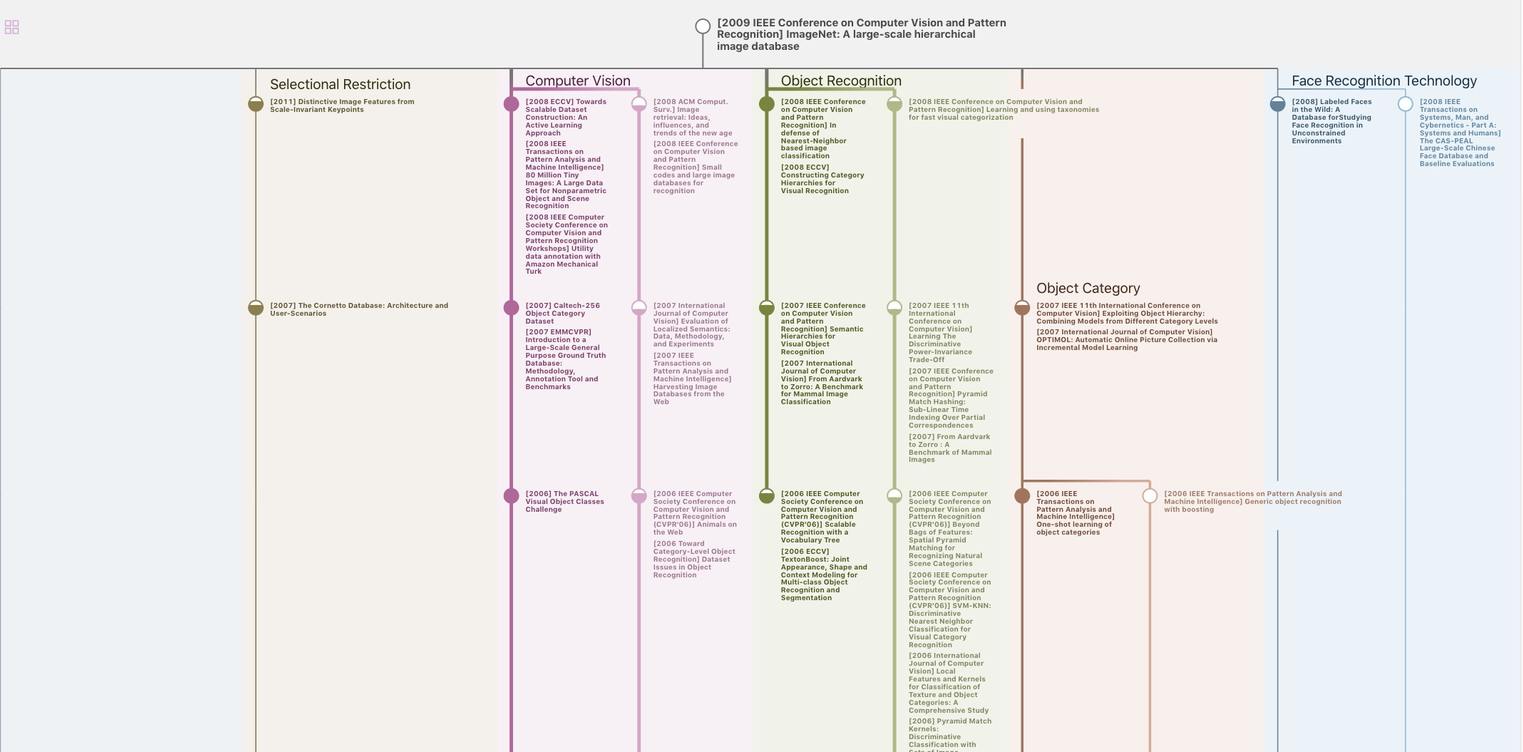
生成溯源树,研究论文发展脉络
Chat Paper
正在生成论文摘要