Temporal reflected logistic regression for probabilistic heart failure survival score prediction
2017 IEEE International Conference on Bioinformatics and Biomedicine (BIBM)(2017)
摘要
Heart failure (HF) has a highly variable annual mortality rate and there is an urgent need of determining patient prognosis to enable informed decision-making about heart failure treatment strategies. Existing survival risk prediction models either require features that limit their applicability or pose difficulties for parameter estimation as physicians have to use a limited set of variables with known hazard ratios published in literature. We propose a new model to predict the probabilistic survival score after HF diagnosis based on all clinical variables derived from the electronic health record (EHR). We formalize the parameter estimation problem by using the maximum likelihood estimation (MLE) principle and devise an effective and efficient algorithm to solve the optimization problem. Experimental results using EHR data of 234 HF patients validate the superiority of this new model in predicting prognosis over the currently used Seattle Heart Failure Model.
更多查看译文
关键词
Temporal Models,Heart Failure (HF) Survival Score Prediction,Logistic Regression,Electronic Health Records
AI 理解论文
溯源树
样例
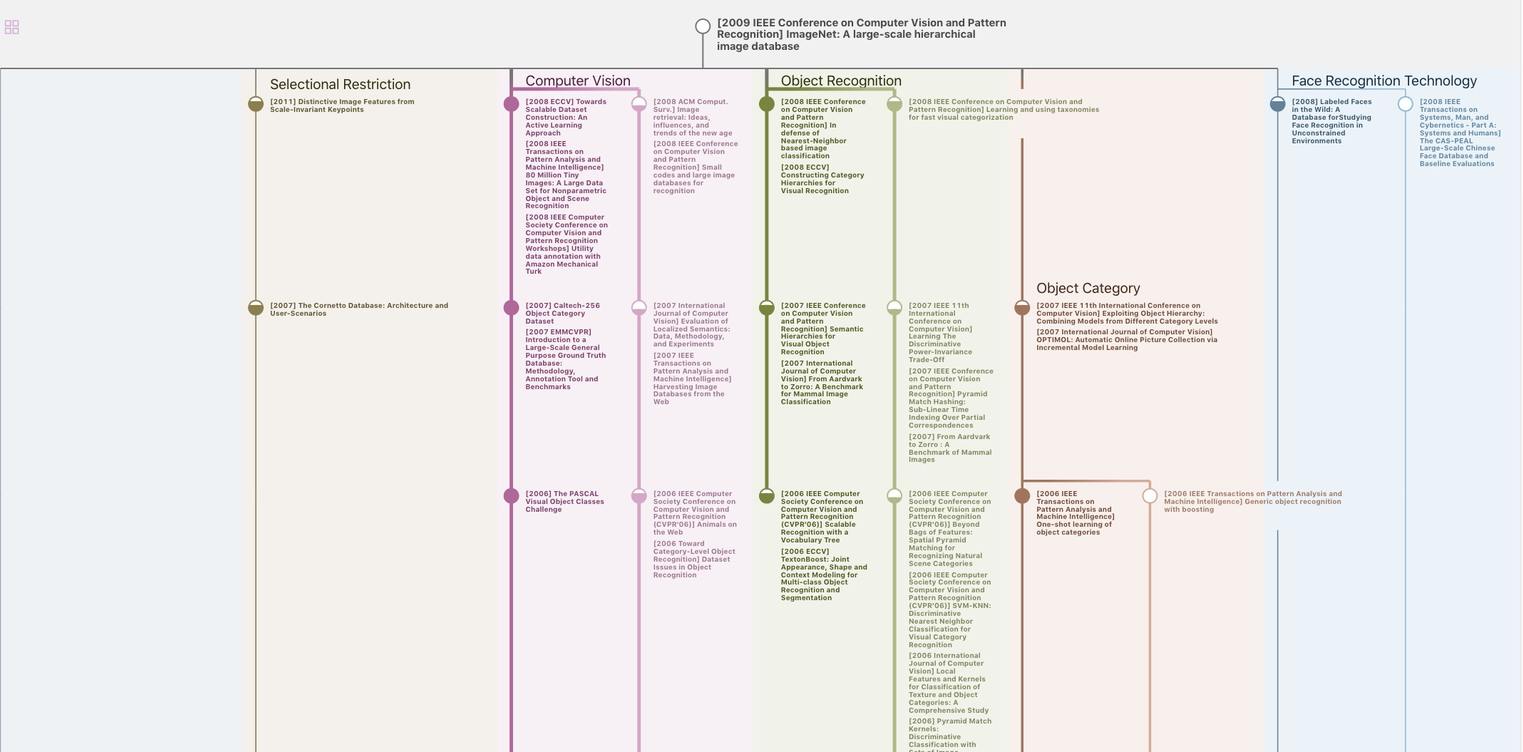
生成溯源树,研究论文发展脉络
Chat Paper
正在生成论文摘要