Generative diffeomorphic modelling of large MRI data sets for probabilistic template construction.
NeuroImage(2018)
摘要
In this paper we present a hierarchical generative model of medical image data, which can capture simultaneously the variability of both signal intensity and anatomical shapes across large populations. Such a model has a direct application for learning average-shaped probabilistic tissue templates in a fully automated manner. While in principle the generality of the proposed Bayesian approach makes it suitable to address a wide range of medical image computing problems, our work focuses primarily on neuroimaging applications. In particular we validate the proposed method on both real and synthetic brain MR scans including the cervical cord and demonstrate that it yields accurate alignment of brain and spinal cord structures, as compared to state-of-the-art tools for medical image registration. At the same time we illustrate how the resulting tissue probability maps can readily be used to segment, bias correct and spatially normalise unseen data, which are all crucial pre-processing steps for MR imaging studies.
更多查看译文
关键词
Atlas construction,Image segmentation,Image registration,Generative modelling,MRI
AI 理解论文
溯源树
样例
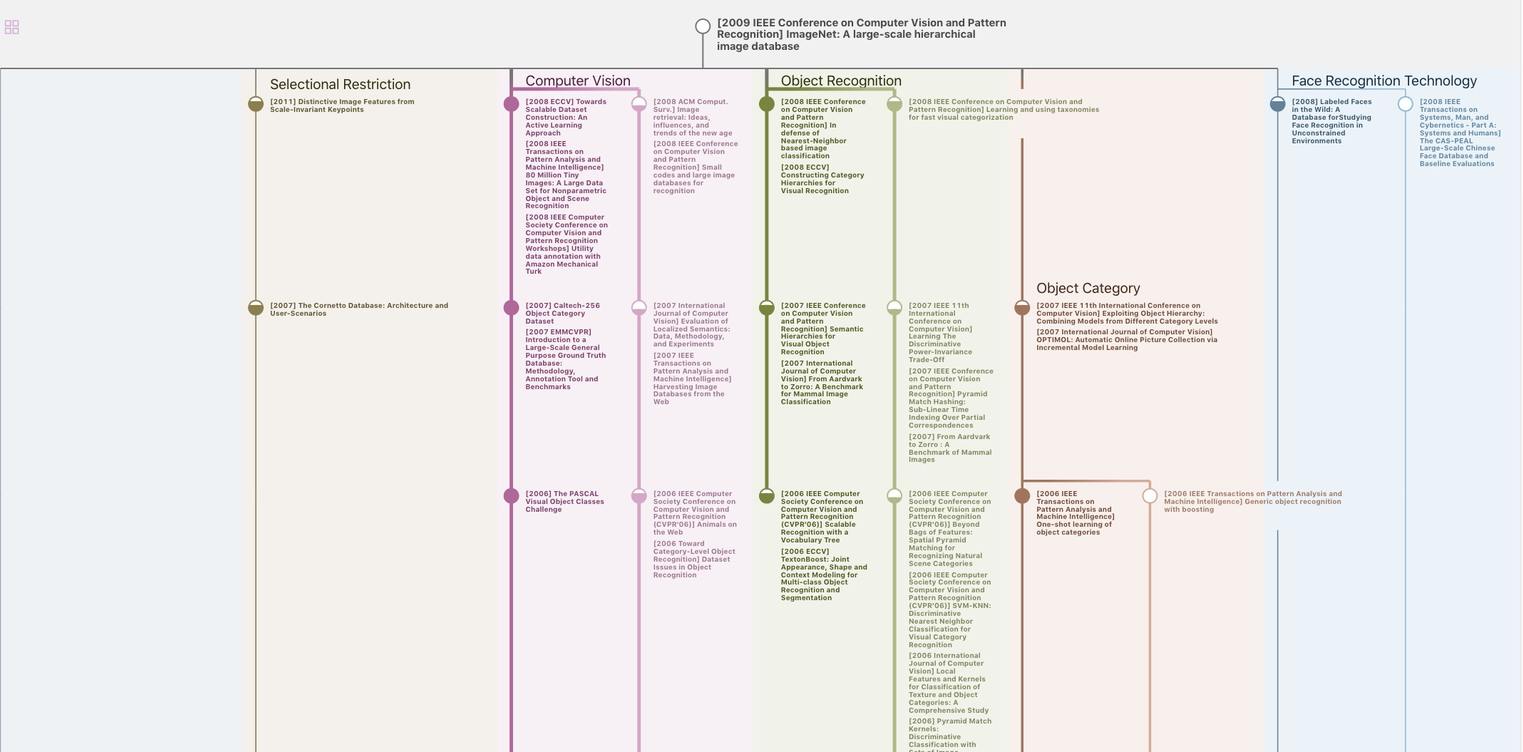
生成溯源树,研究论文发展脉络
Chat Paper
正在生成论文摘要