An MVPA method based on sparse representation for pattern localization in fMRI data analysis
Neurocomputing(2017)
摘要
A new MVPA method for fMRI data analysis.The ability of detecting subtle differences between experimental conditions.We localized two category-specific brain activation patterns corresponding to two experimental conditions.The two sets consisted of a maximal number of informative features.The wrong selected features (noises) were removed by permutation tests. Multivariate pattern analysis (MVPA) approach applied to neuroimaging data, such as functional magnetic resonance imaging (fMRI) data, has received a great deal of attention because of its sensitivity to distinguishing patterns of neural activities associated with different stimuli or cognitive states. Generally, when using MVPA approach to decode the mental states or stimuli, a set of discriminative variables (e.g., voxels) is first selected. However, in most of existing MVPA methods, the selected variables do not contain all informative variables, since these selected variables are sufficient for decoding. In this paper, we propose a multivariate pattern analysis method based on sparse representation for decoding the brain states and localizing category-specific brain activation areas corresponding to two experimental conditions/tasks at the same time. Unlike traditional MVPA approaches, this method is designed to find informative variables as many as possible. We applied the proposed method to two judgement experiments: a gender discrimination and an emotion discrimination task, data analysis results demonstrate its effectiveness and potential applications.
更多查看译文
关键词
Sparse representation,Localizing,Decoding,fMRI
AI 理解论文
溯源树
样例
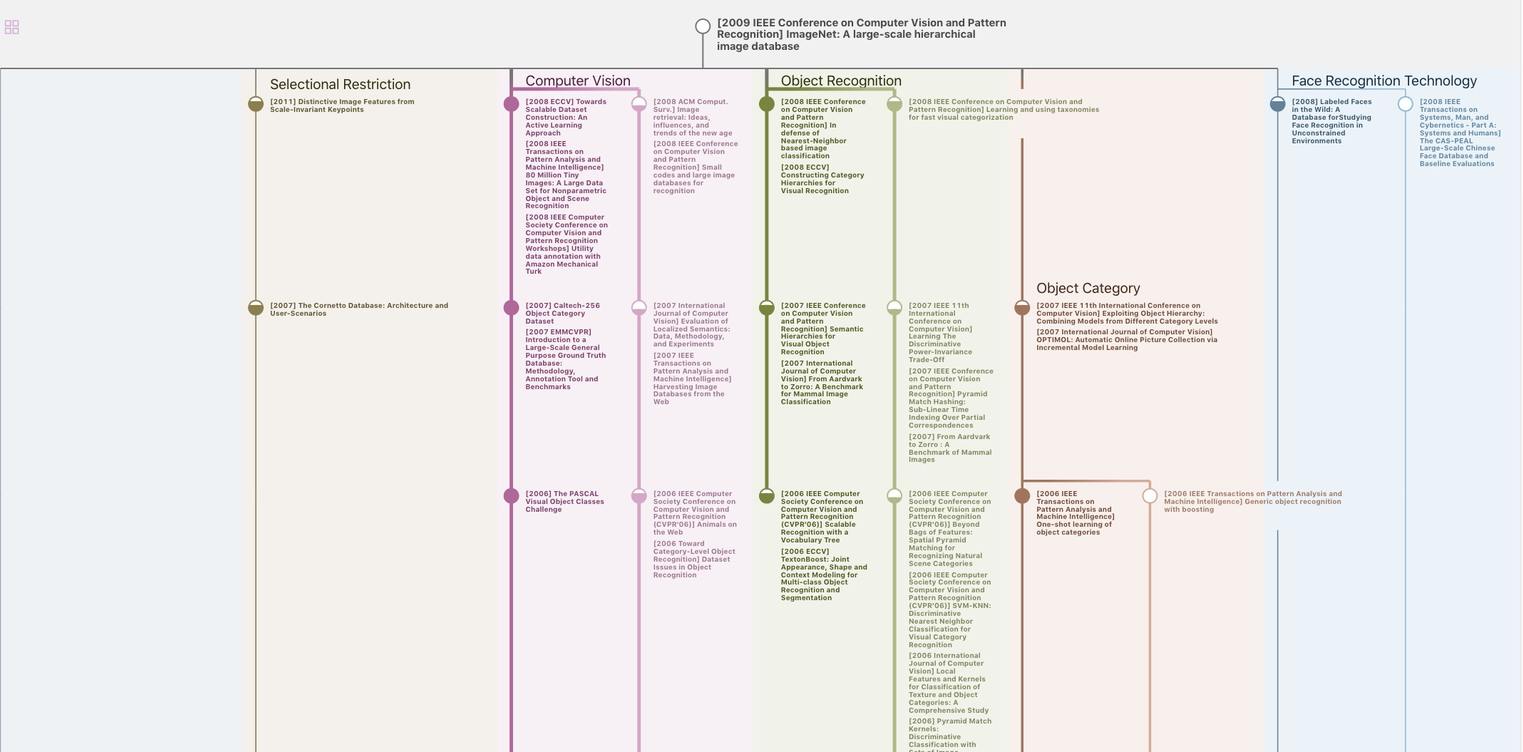
生成溯源树,研究论文发展脉络
Chat Paper
正在生成论文摘要