Air-water interface of submerged superhydrophobic surfaces imaged by atomic force microscopy.
BEILSTEIN JOURNAL OF NANOTECHNOLOGY(2017)
摘要
Underwater air retention of superhydrophobic hierarchically structured surfaces is of increasing interest for technical applications. Persistent air layers (the Salvinia effect) are known from biological species, for example, the floating fern Salvinia or the back-swimmer Notonecta. The use of this concept opens up new possibilities for biomimetic technical applications in the fields of drag reduction, antifouling, anticorrosion and under water sensing. Current knowledge regarding the shape of the air-water interface is insufficient, although it plays a crucial role with regards to stability in terms of diffusion and dynamic conditions. Optical methods for imaging the interface have been limited to the micrometer regime. In this work, we utilized a nondynamic and nondestructive atomic force microscopy (AFM) method to image the interface of submerged superhydrophobic structures with nanometer resolution. Up to now, only the interfaces of nanobubbles (acting almost like solids) have been characterized by AFM at these dimensions. In this study, we show for the first time that it is possible to image the air-water interface of submerged hierarchically structured (micro-pillars) surfaces by AFM in contact mode. By scanning with zero resulting force applied, we were able to determine the shape of the interface and thereby the depth of the water penetrating into the underlying structures. This approach is complemented by a second method: the interface was scanned with different applied force loads and the height for zero force was determined by linear regression. These methods open new possibilities for the investigation of air-retaining surfaces, specifically in terms of measuring contact area and in comparing different coatings, and thus will lead to the development of new applications.
更多查看译文
关键词
AFM in liquid,air retention,atomic force microscopy,bionics,Salvinia effect
AI 理解论文
溯源树
样例
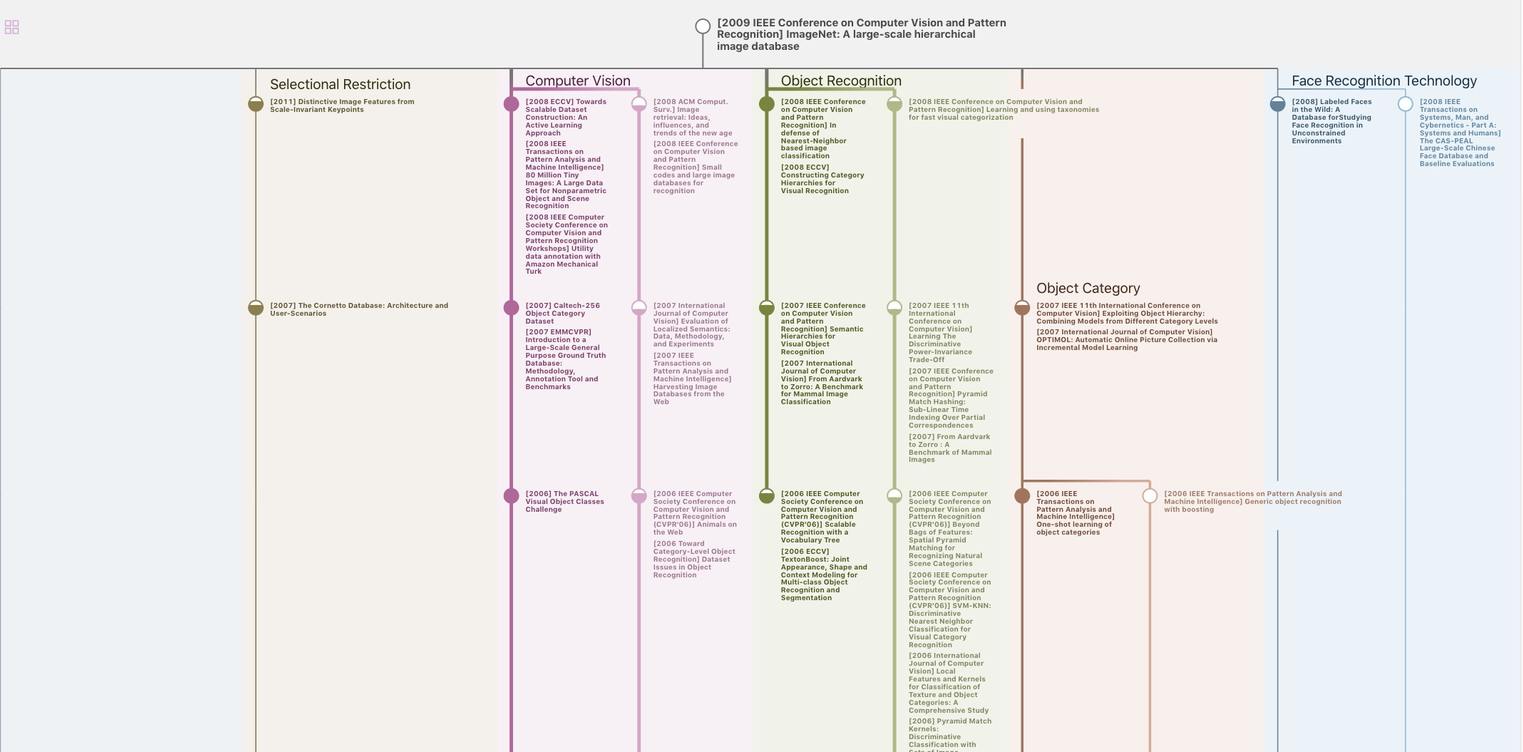
生成溯源树,研究论文发展脉络
Chat Paper
正在生成论文摘要