Compressive Sensing In Wireless Multimedia Sensor Networks Based On Low-Rank Approximation
2017 IEEE INTERNATIONAL CONFERENCE ON COMMUNICATIONS (ICC)(2017)
摘要
For the large number of the image data produced by the sensor nodes in wireless multimedia sensor networks (WMSNs), the reduction of the sensor data and energy efficient transmission of this data are the most challenging problem. Compressed sensing based image compression provides the dramatic reduction of image sampling rates, energy consumption for WMSNs data collection and transmission. For better suitable for real-time sensing of image and reduce the sensing matrix size, block compressed sensing method acquire and process image in a block-by-block manner, by the same operator. Recently, nonlocal sparsity has been evidenced to improve the reconstruction of image details in various compressed sensing studies. In this paper, based on block compressed sensing, both the local sparsity of the whole image and nonlocal sparsity of similar patches are integrated into a compressed sensing recovery framework. And the nonlocal sparsity of images is characterized by the low-rank approximation. For better approximation of the rank function, the non-convex low-rank regularization namely Schatten p-norm minimization is applied for block compressed sensing recovery. The experiments show that the proposed algorithm can reduce the reconstruction error and improve the quality of the reconstruction image. Moreover, the proposed algorithm is suitable for WMSNs with few memory and low transmission bandwidth.
更多查看译文
关键词
Wireless multimedia sensor networks, Block compressed sensing, Low-rank approximation, Schatten-p-norm
AI 理解论文
溯源树
样例
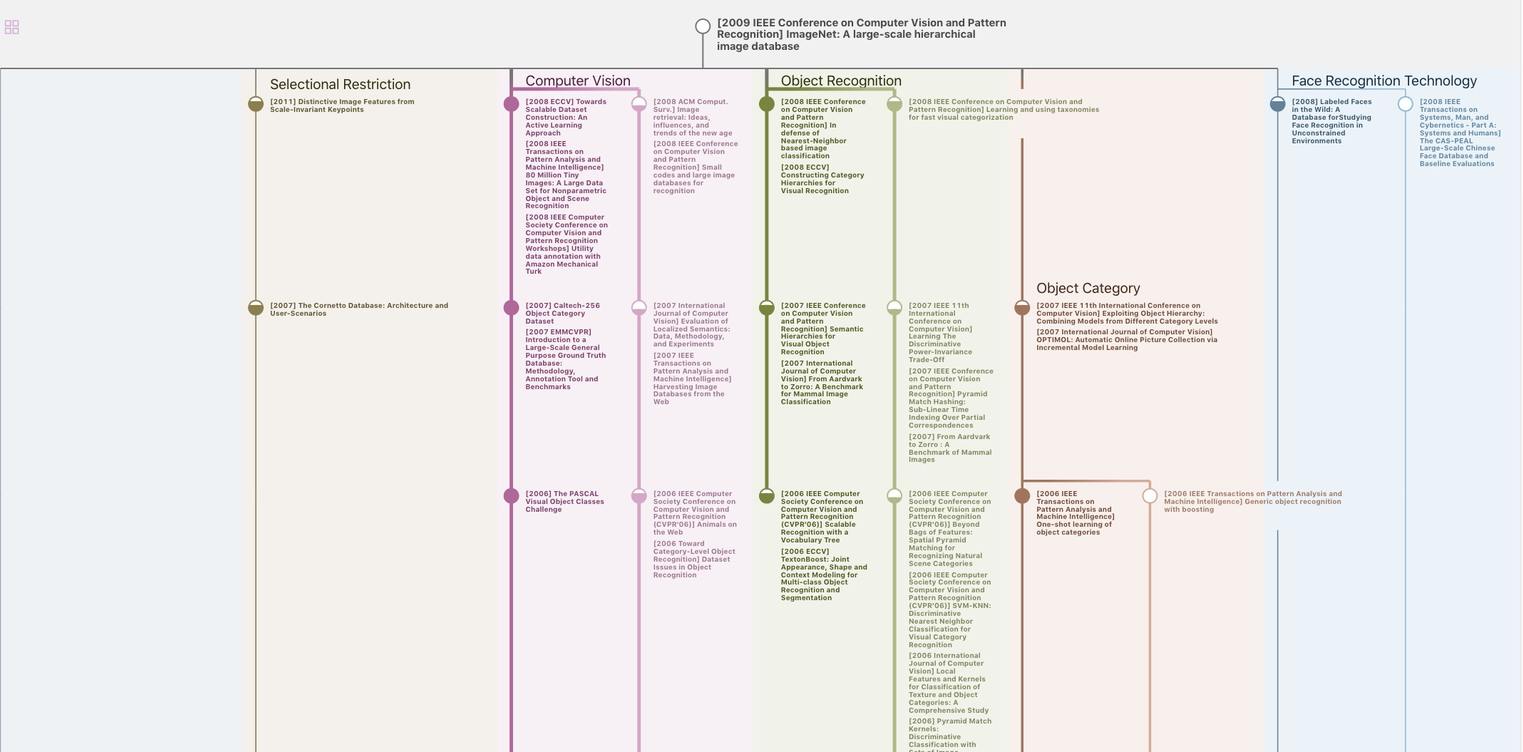
生成溯源树,研究论文发展脉络
Chat Paper
正在生成论文摘要