Error Bounds For Bregman Denoising And Structured Natural Parameter Estimation
2017 IEEE INTERNATIONAL SYMPOSIUM ON INFORMATION THEORY (ISIT)(2017)
摘要
We analyze an estimator based on the Bregman divergence for recovery of structured models from additive noise. The estimator can be seen as a regularized maximum likelihood estimator for an exponential family where the natural parameter is assumed to be structured. For all such Bregman denoising estimators, we provide an error bound for a natural associated error measure. Our error bound makes it possible to analyze a wide range of estimators, such as those in proximal denoising and inverse covariance matrix estimation, in a unified manner. In the case of proximal denoising, we exactly recover the existing tight normalized mean squared error bounds. In sparse precision matrix estimation, our bounds provide optimal scaling with interpretable constants in terms of the associated error measure.
更多查看译文
关键词
error bounds,structured natural parameter estimation,additive noise,regularized maximum likelihood estimator,exponential family,Bregman denoising estimators,natural associated error measure,proximal denoising,inverse covariance matrix estimation,tight normalized mean squared error bounds,sparse precision matrix estimation,signal denoising
AI 理解论文
溯源树
样例
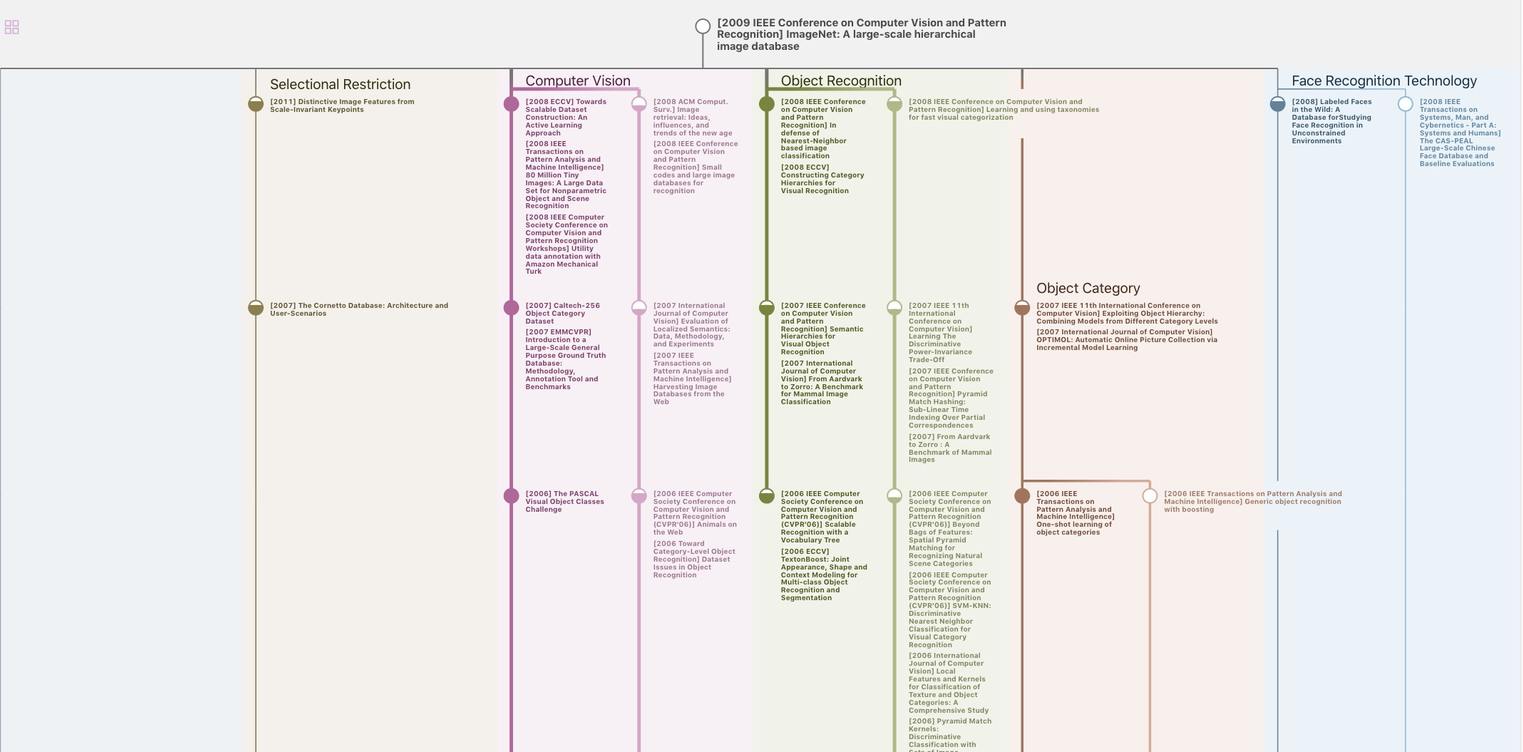
生成溯源树,研究论文发展脉络
Chat Paper
正在生成论文摘要