Bayesian Diffusion Tensor Estimation With Spatial Priors
COMPUTER ANALYSIS OF IMAGES AND PATTERNS(2017)
摘要
Spatial regularization is a technique that exploits the dependence between nearby regions to locally pool data, with the effect of reducing noise and implicitly smoothing the data. Most of the currently proposed methods are focused on minimizing a cost function, during which the regularization parameter must be tuned in order to find the optimal solution. We propose a fast Markov chain Monte Carlo (MCMC) method for diffusion tensor estimation, for both 2D and 3D priors data. The regularization parameter is jointly with the tensor using MCMC. We compare FA (fractional anisotropy) maps for various b-values using three diffusion tensor estimation methods: least-squares and MCMC with and without spatial priors. Coefficient of variation (CV) is calculated to measure the uncertainty of the FA maps calculated from the MCMC samples, and our results show that the MCMC algorithm with spatial priors provides a denoising effect and reduces the uncertainty of the MCMC samples.
更多查看译文
关键词
Spatial regularization, Diffusion tensor, Spatial priors, Markov chain Monte Carlo, Fractional anisotropy
AI 理解论文
溯源树
样例
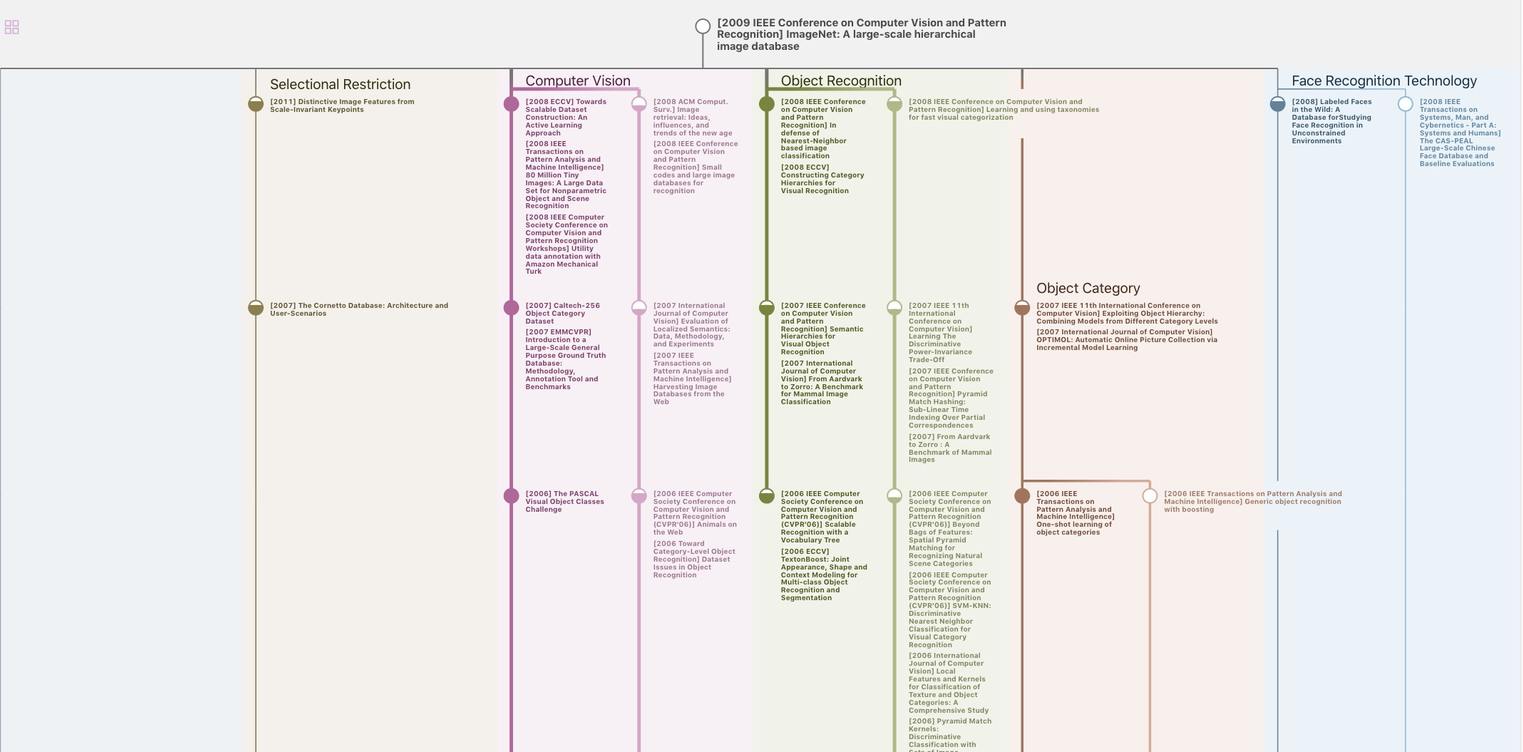
生成溯源树,研究论文发展脉络
Chat Paper
正在生成论文摘要