Robust Features For Snapshot Hyperspectral Terrain-Classification
COMPUTER ANALYSIS OF IMAGES AND PATTERNS(2017)
摘要
Hyperspectral imaging increases the amount of information incorporated per pixel in comparison to normal RGB color cameras. Conventional spectral cameras as used in satellite imaging use spatial or spectral scanning during acquisition which is only suitable for static scenes. In dynamic scenarios, such as in autonomous driving applications, the acquisition of the entire hyperspectral cube at the same time is mandatory. We investigate the eligibility of novel snapshot hyperspectral cameras which capture an entire hyperspectral cube without requiring moving parts or line-scanning. Captured hyperspectral data is used for multi class terrain classification utilizing machine learning techniques. Prior to classification, the data is segmented using Superpixel segmentation which is modified to work successfully on hyperspectral data. We further investigate a simple approach to normalize the hyperspectral data in terms of illumination, which yields vast improvements in classification accuracy, preventing most errors caused by shading and other influences. Furthermore we utilize Gabor texture features which add spatial information to the feature space without increasing the data dimensionality in an excessive fashion. The multi-class classification is evaluated against a novel hyperspectral ground truth dataset specifically created for this purpose.
更多查看译文
关键词
Hyperspectral imaging, Terrain classification, Spectral analysis, Autonomous vehicles
AI 理解论文
溯源树
样例
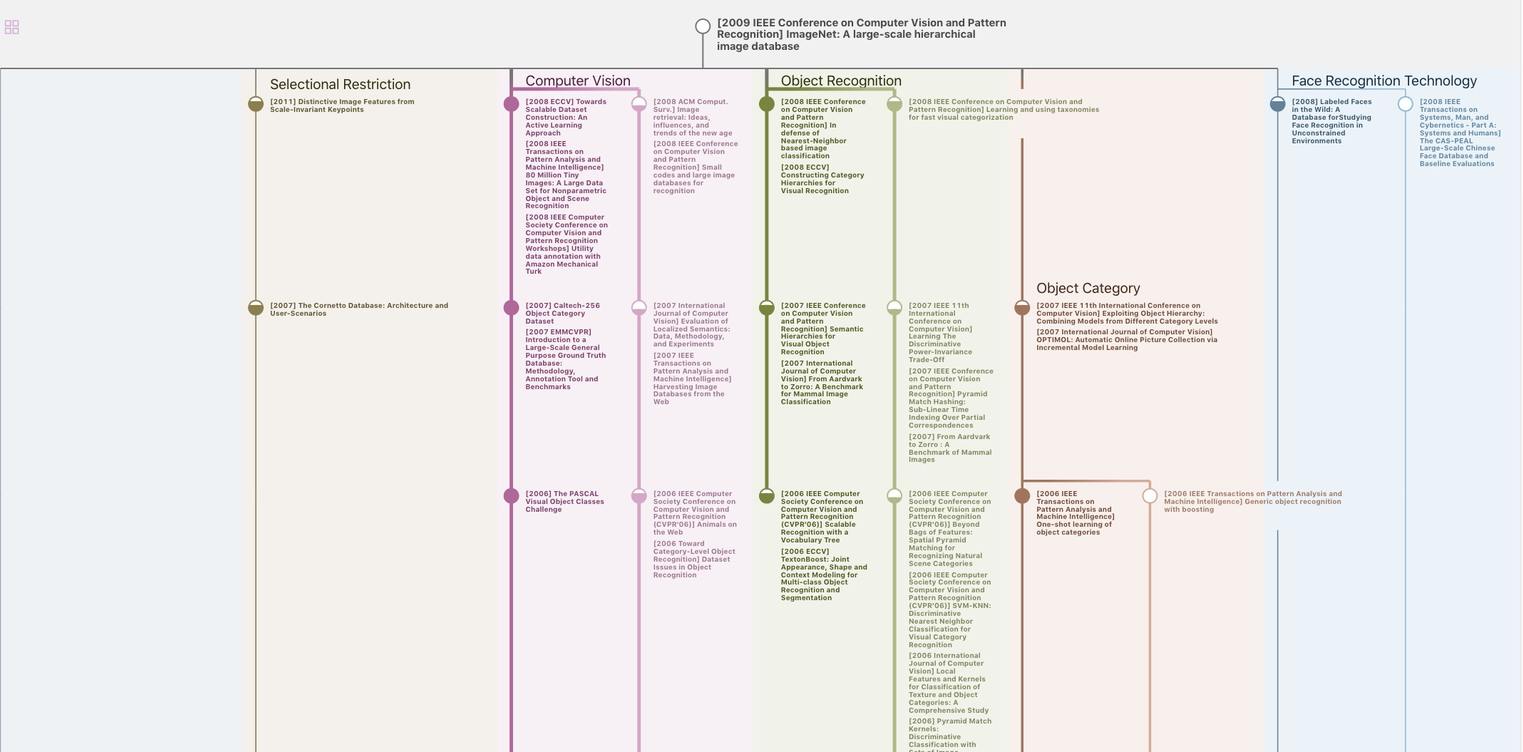
生成溯源树,研究论文发展脉络
Chat Paper
正在生成论文摘要