Detection and Prediction of Natural Hazards Using Large-Scale Environmental Data.
ADVANCES IN SPATIAL AND TEMPORAL DATABASES, SSTD 2017(2017)
摘要
Recent developments in remote sensing have made it possible to instrument and sense the physical world with high resolution and fidelity. Consequently, very large spatio-temporal environmental data sets, have become available to the research community. Such data consists of time-series, starting as early as 1973, monitoring up to thousands of environmental parameters, for each spatial region of a resolution as low as 0.5' x 0.5'. To make this flood of data actionable, in this work, we employ a data driven approach to detect and predict natural hazards. Our supervised learning approach learns from labeled historic events. We describe each event by a three-mode tensor, covering space, time and environmental parameters. Due to the very large number of environmental parameters, and the possibility of latent features hidden within these parameters, we employ a tensor factorization approach to learn latent factors. As the corresponding tensors can grow very large, we propose to employ an outlier-score for sparsification, thus explicitly modeling interesting (location, time, parameter) triples only. In our experimental evaluation, we apply our data-driven learning approach to the use-case of predicting the rapid-intensification of tropical storms. Learning from past tropical storms, we show that our approach is able to predict the future rapid-intesification of tropical storms with high accuracy, matching the accuracy of domain specific solutions, yet without using any domain knowledge.
更多查看译文
AI 理解论文
溯源树
样例
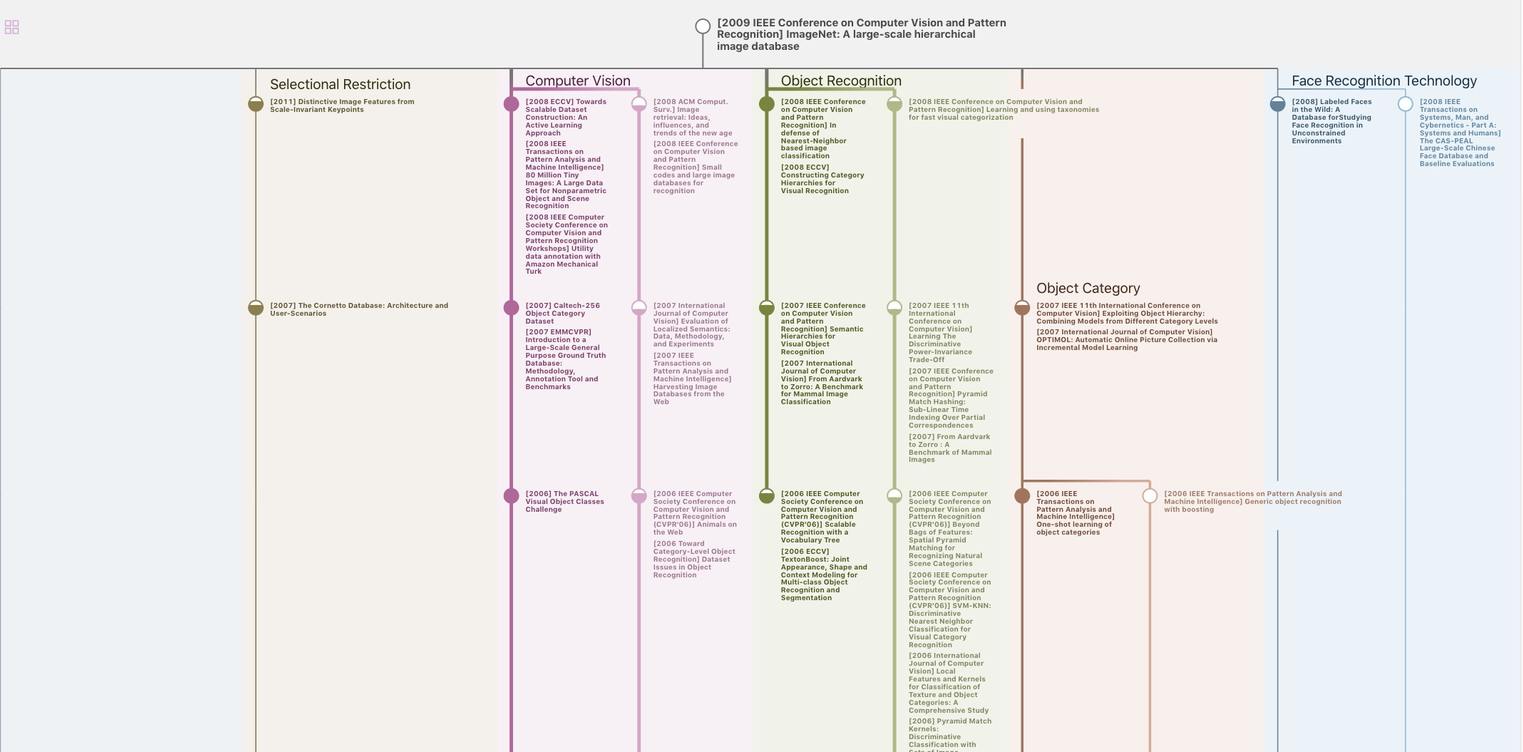
生成溯源树,研究论文发展脉络
Chat Paper
正在生成论文摘要