Offline reconstruction of missing vehicle trajectory data from 3D LIDAR
2017 28TH IEEE INTELLIGENT VEHICLES SYMPOSIUM (IV 2017)(2017)
摘要
LIDAR has become an important part of many autonomous vehicles with its advantages on distance measurement and obstacle detection. LIDAR produces point clouds which have important information about surrounding environment. In this paper, we collected trajectory data on a two lane urban road using a Velodyne VLP-16 Lidar. Due to dynamic nature of data collection and limited range of the sensor, some of these trajectories have missing points or gaps. In this paper, we propose a novel method for recovery of missing vehicle trajectory data points using microscopic traffic flow models. While short gaps (less than 5 seconds) can be recovered with simple linear regression, and longer gaps are recovered with the proposed method that makes use of car following models calibrated by assigning weights to known points based on proximity to the gaps. Newell's, Pipes, IDM and Gipps' car following models are calibrated and tested with the ground truth trajectory data from LIDAR and NGSIM I-80 dataset. Gipps' calibrated model yielded the best result.
更多查看译文
关键词
offline reconstruction,3D LIDAR,autonomous vehicles,distance measurement,obstacle detection,point clouds,surrounding environment,urban road,Velodyne VLP-16 Lidar,data collection,missing vehicle trajectory data points,microscopic traffic flow models,linear regression,car following models,ground truth trajectory data,NGSIM I-80 dataset,calibrated model
AI 理解论文
溯源树
样例
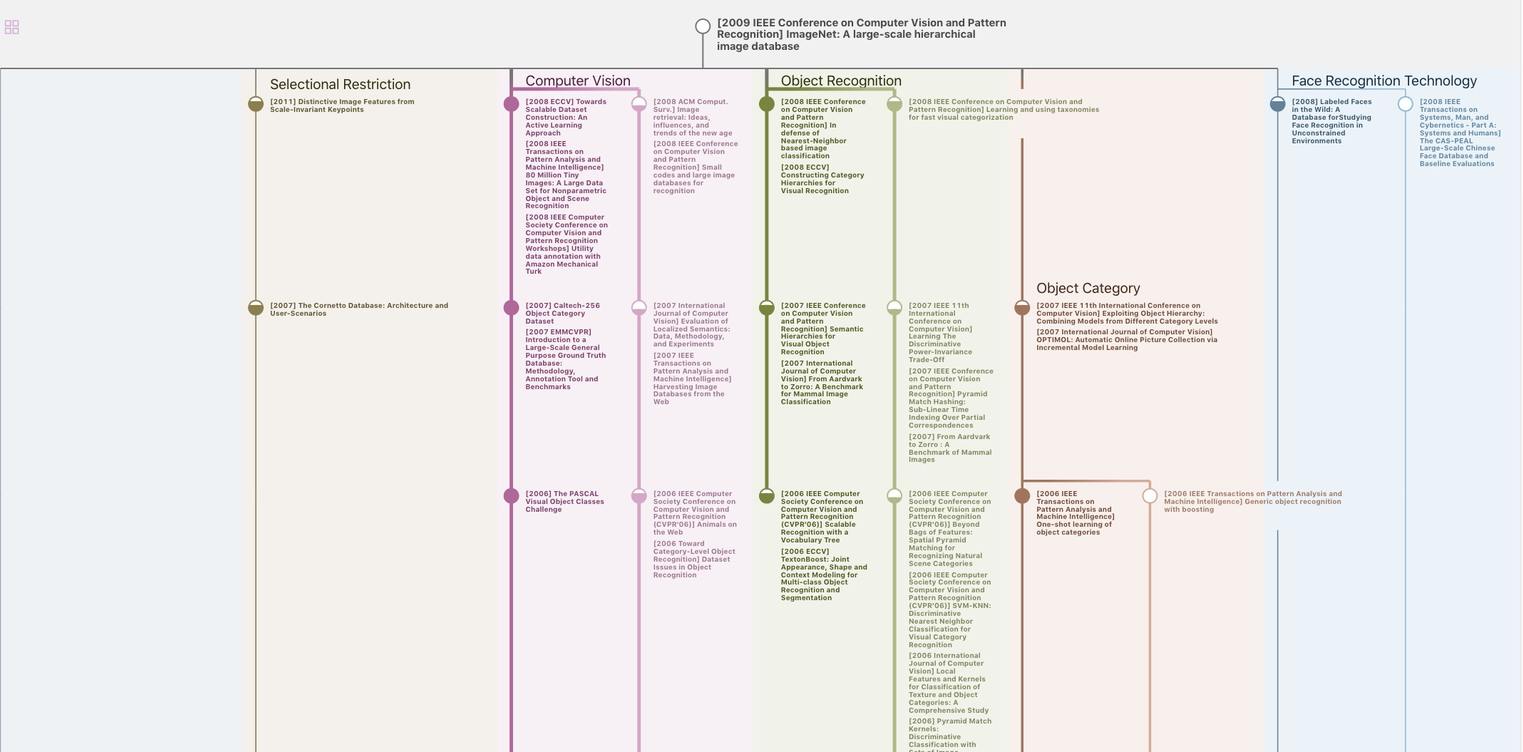
生成溯源树,研究论文发展脉络
Chat Paper
正在生成论文摘要