Probabilistic Long-Term Prediction For Autonomous Vehicles
2017 28TH IEEE INTELLIGENT VEHICLES SYMPOSIUM (IV 2017)(2017)
摘要
Long-term prediction of traffic participants is crucial to enable autonomous driving on public roads. The quality of the prediction directly affects the frequency of trajectory planning. With a poor estimation of the future development, more computational effort has to be put in re-planning, and a safe vehicle state at the end of the planning horizon is not guaranteed. A holistic probabilistic prediction, considering inputs, results and parameters as random variables, highly reduces the problem. A time frame of several seconds requires a probabilistic description of the scene evolution, where uncertainty or accuracy is represented by the trajectory distribution. Following this strategy, a novel evaluation method is needed, coping with the fact, that the future evolution of a scene is also uncertain. We present a method to evaluate the probabilistic prediction of real traffic scenes with varying start conditions. The proposed prediction is based on a particle filter, estimating behavior describing parameters of a microscopic traffic model. Experiments on real traffic data with random leading vehicles show the applicability in terms of convergence, enabling long-term prediction using forward propagation.
更多查看译文
关键词
probabilistic long-term prediction,autonomous vehicles,traffic participants,public roads,trajectory planning,safe vehicle state,holistic probabilistic prediction,random variables,probabilistic description,trajectory distribution,particle filter,microscopic traffic model,convergence
AI 理解论文
溯源树
样例
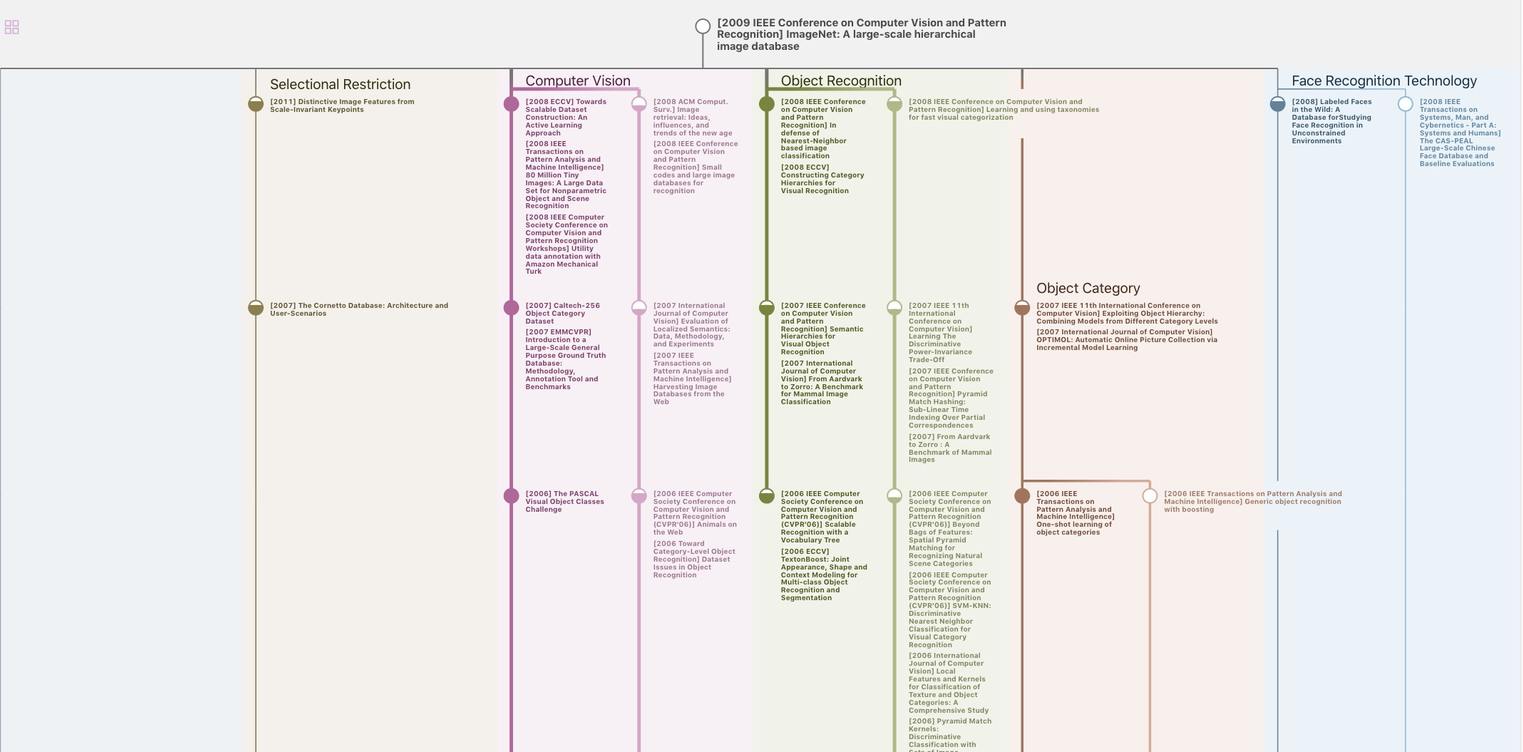
生成溯源树,研究论文发展脉络
Chat Paper
正在生成论文摘要