A Hybrid Bundle Adjustment/Pose-Graph Approach To Vslam/Gps Fusion For Low-Capacity Platforms
2017 28TH IEEE INTELLIGENT VEHICLES SYMPOSIUM (IV 2017)(2017)
摘要
We focus on the real-time fusion of monocular visual SLAM with GPS data in order to obtain city-scale, georeferenced pose estimations and reconstructions. Recently, GPS/VSLAM fusion through constrained local key-frame based Bundle Adjustment (BA) using Barrier Term Optimization (BTO) has proven to be (to the best of our knowledge) the most robust and accurate method. However, this approach requires a higher number of cameras to be considered in the optimization: in practice, more than 30 cameras are necessary, while a typical vision-only BA can succeed with as few as 10 cameras. This problem dimensionality makes the method unsuitable for autonomous embedded platforms of low computational capacity (e.g. MAVs). In this paper, we present a hybrid constrained BA/pose-graph approach using BTO, which is motivated by theoretical observations about covariance changes as a function of the gauge. We show that our method has desirable properties that allows its successful use in a BTO context, and present two different formulations. The experimental validation of our method shows that both our formulations reduce the computational cost in comparison with constrained BA using BTO, without any significant loss of precision. In particular, our first formulation yields a 60% reduction in execution time.
更多查看译文
关键词
hybrid bundle adjustment/pose-graph approach,VSLAM/GPS fusion,low-capacity platforms,real-time fusion,monocular visual SLAM,GPS data,city-scale georeferenced pose estimations,pose reconstructions,constrained local key-frame,barrier term optimization,BTO,vision-only BA,hybrid constrained BA/pose-graph approach,visual simultaneous localization and mapping
AI 理解论文
溯源树
样例
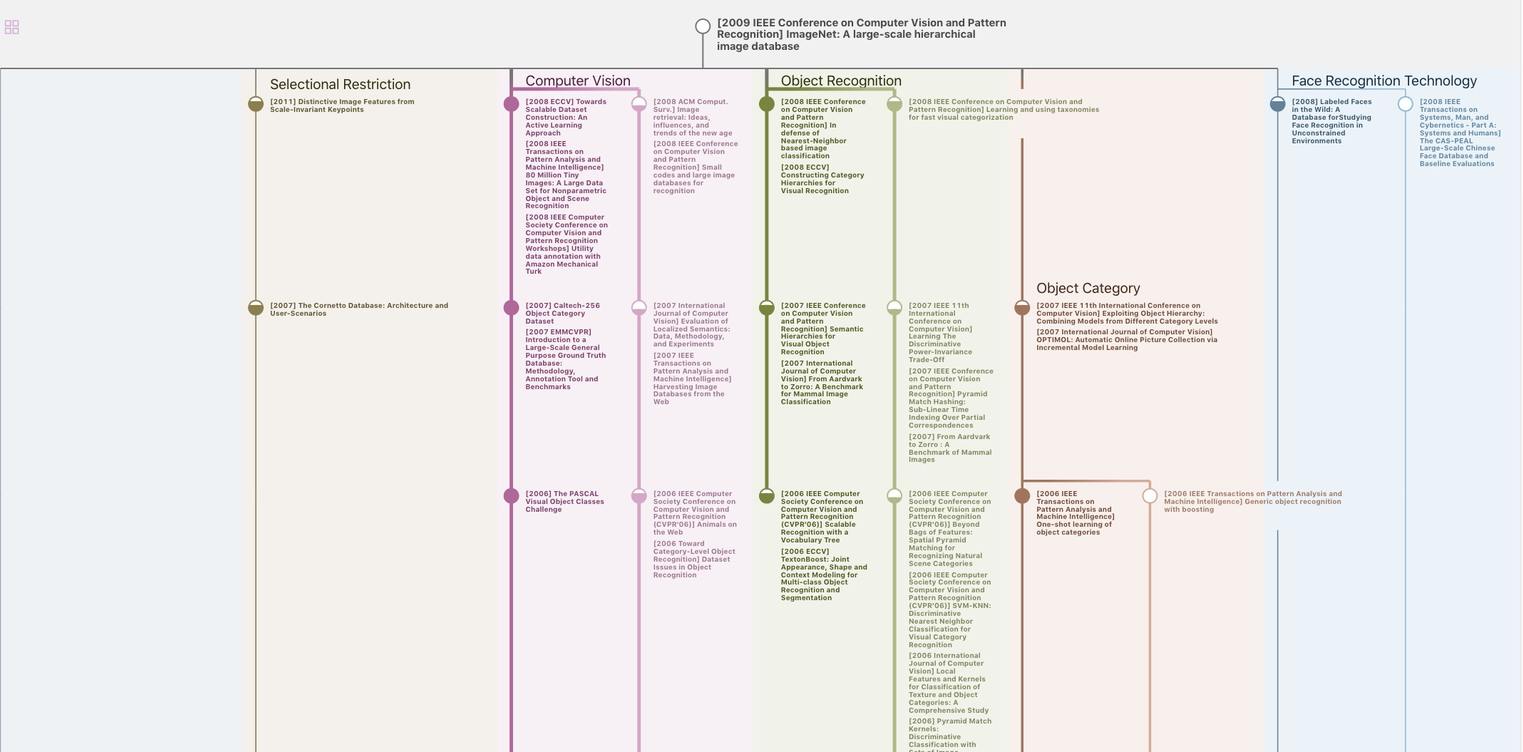
生成溯源树,研究论文发展脉络
Chat Paper
正在生成论文摘要