Towards a More General XCS: Classifier Fusion and Don’t Cares in Actions
ADVANCES IN SOFT COMPUTING, MICAI 2016, PT II(2017)
摘要
Wilson’s XCS represents and stores the knowledge it has acquired from an environment as a set of classifiers. In the XCS, don’t cares (#) may be used in the conditions of classifiers to express generalization. This paper is focused on the representation of knowledge with the minimal number of classifiers. For this purpose, a new process called fusion is implemented. Fusion promotes the emergence of more generalized yet accurate classifiers and the reduction of the number of macroclassifiers. Furthermore, to get even more compact rules sets, the implementation of the # symbol in the action of the classifiers is proposed; this allows generalization when possible, and the existence non-competing classifiers in the population if a state has multiple equally correct actions that can be performed. The proposed modified generalized extended XCS (gXCS) was compared with the XCS on the Woods2 environment and a modification of this environment, modified-Woods2, that has locations where there are multiple equally good actions. The performances of XCS and gXCS are very similar; yet, gXCS obtains more parsimonious rule sets. Furthermore, gXCS can find good rule sets even when the probability of # is set zero, contrary to the XCS.
更多查看译文
关键词
XCS,gXCS,# symbol,Don’t cares,Learning classifier systems,Generalization,Fusion
AI 理解论文
溯源树
样例
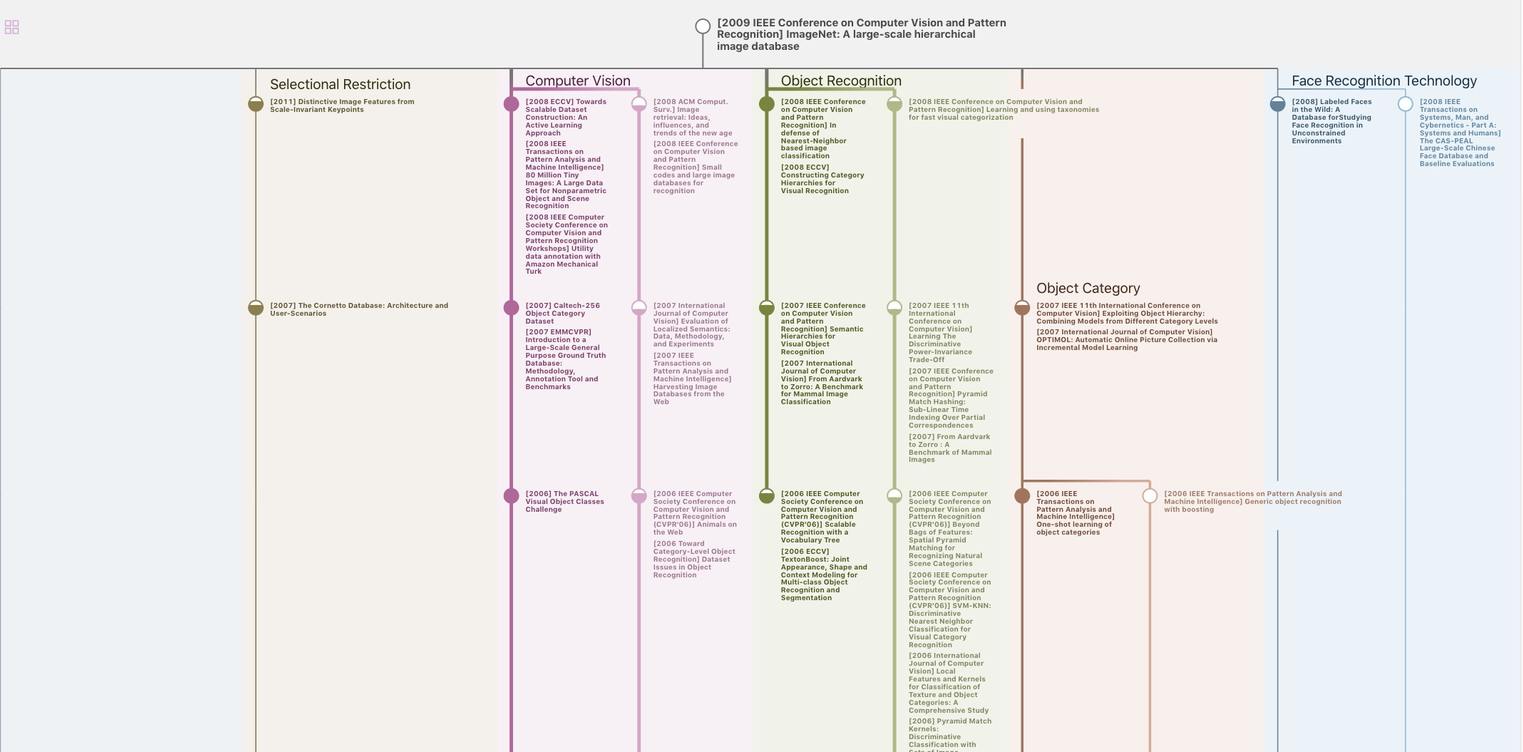
生成溯源树,研究论文发展脉络
Chat Paper
正在生成论文摘要