Exploring Complex Networks with Failure-Prone Agents.
ADVANCES IN SOFT COMPUTING, MICAI 2016, PT II(2017)
摘要
Distributed data-collection and synchronization is essential in sensor networks and the Internet of Things (IoT), as well as for datareplication in server farms, clusters and clouds. Generally, such systems consist of a set of interconnected components, which cooperate and coordinate to achieve a collective task, while acting locally and being failureprone. An important challenge is hence to define efficient and robust algorithms for data collection and synchronisation in large-scale, distributed and failure-prone platforms. This paper studies the performance and robustness of different multi-agent algorithms in complex networks with different topologies (Lattice, Small-world, Community and Scale-free) and different agent failure rates. Agents proceed from random locations and explore the network to collect local data hosted in each node. Their exploration algorithm determines how fast they cover unexplored nodes to collect new data, and how often they meet other agents to exchange complementary data and speed-up the process. Two exploration algorithms are studied: one random and one using a stigmergy model (that we propose). Experimental results show how network topologies and agent failure-rates impact data-collection and synchronization, and how a stigmergy-based approach can improve performance and success rates across most scenarios. We believe these results offer key insights into the suitability of various decentralised algorithms in different networked environments, which are increasingly at the core of modern information and communication technology (ICT) systems.
更多查看译文
AI 理解论文
溯源树
样例
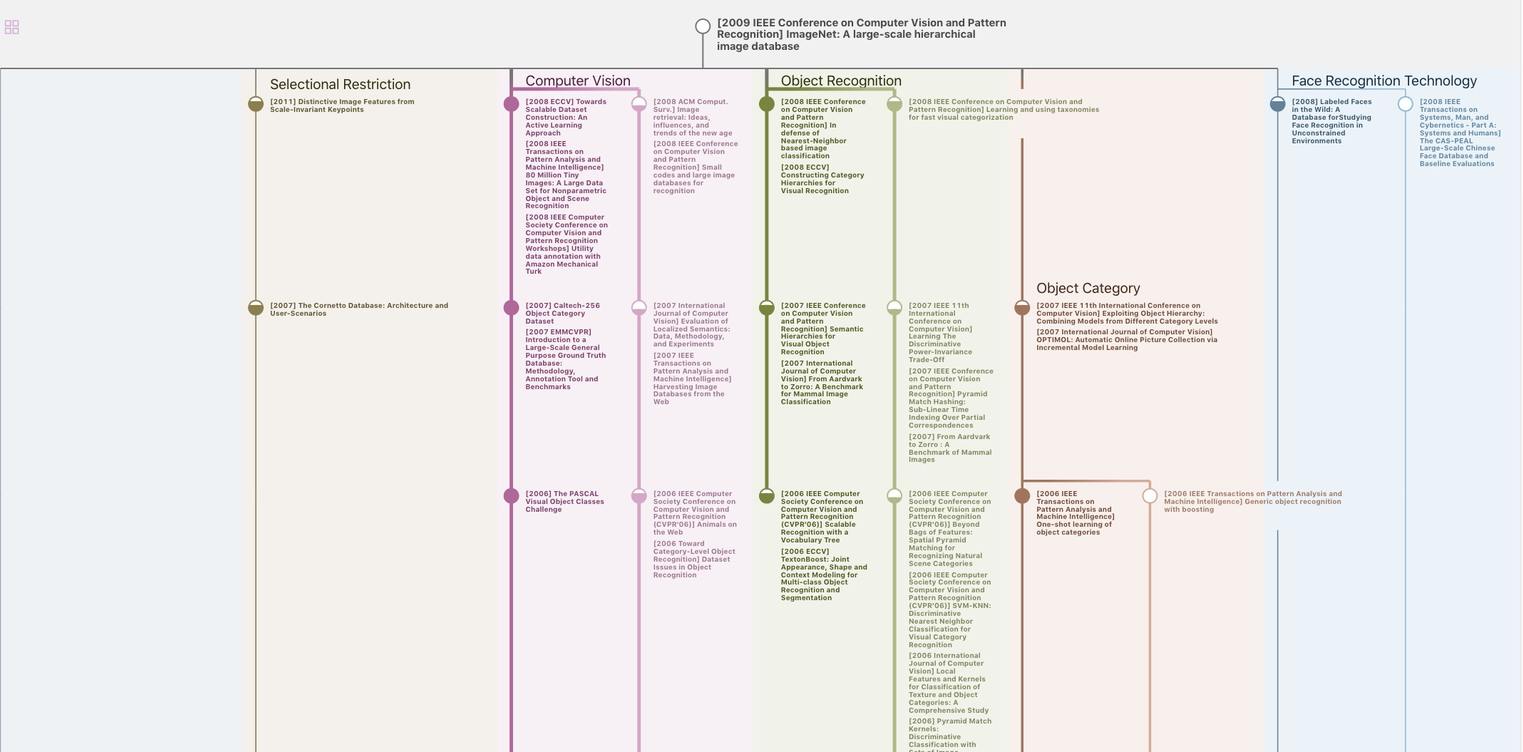
生成溯源树,研究论文发展脉络
Chat Paper
正在生成论文摘要