Adaptive pattern search for large-scale optimization
Appl. Intell.(2017)
摘要
The emergence of high-dimensional data requires the design of new optimization methods. Indeed, conventional optimization methods require improvements, hybridization, or parameter tuning in order to operate in spaces of high dimensions. In this paper, we present a new adaptive variant of a pattern search algorithm to solve global optimization problems exhibiting such a character. The proposed method has no parameters visible to the user and the default settings, determined by almost no a priori experimentation, are highly robust on the tested datasets. The algorithm is evaluated and compared with 11 state-of-the-art methods on 20 benchmark functions of 1000 dimensions from the CEC’2010 competition. The results show that this approach obtains good performances compared to the other methods tested.
更多查看译文
关键词
Pattern search,Scatter search,Optimization,Continuous,High-dimension,Large-scale,Adaptive methods
AI 理解论文
溯源树
样例
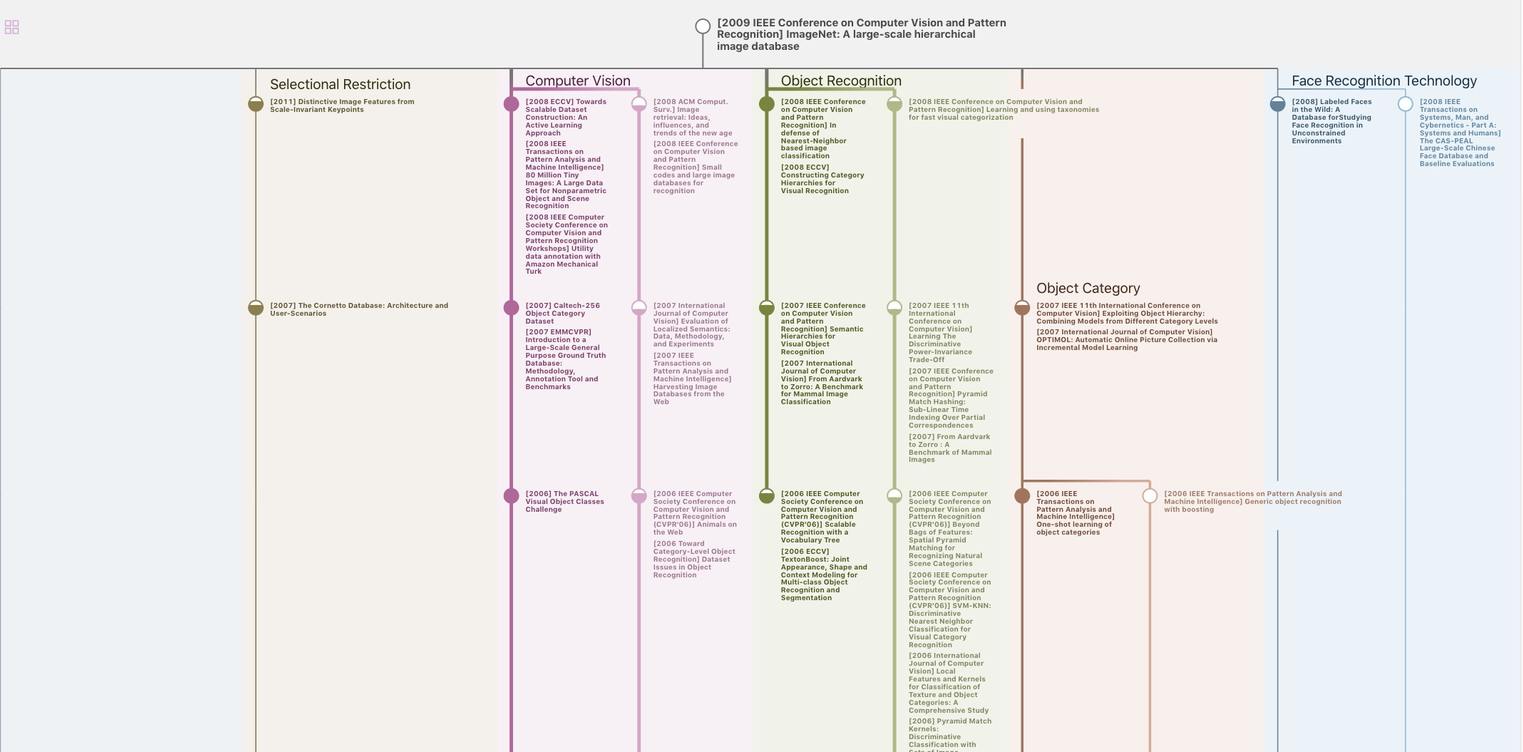
生成溯源树,研究论文发展脉络
Chat Paper
正在生成论文摘要