Learning To Teach Reinforcement Learning Agents
MACHINE LEARNING AND KNOWLEDGE EXTRACTION(2019)
摘要
In this article, we study the transfer learning model of action advice under a budget. We focus on reinforcement learning teachers providing action advice to heterogeneous students playing the game of Pac-Man under a limited advice budget. First, we examine several critical factors affecting advice quality in this setting, such as the average performance of the teacher, its variance and the importance of reward discounting in advising. The experiments show that the best performers are not always the best teachers and reveal the non-trivial importance of the coefficient of variation (CV) as a statistic for choosing policies that generate advice. The CV statistic relates variance to the corresponding mean. Second, the article studies policy learning for distributing advice under a budget. Whereas most methods in the relevant literature rely on heuristics for advice distribution, we formulate the problem as a learning one and propose a novel reinforcement learning algorithm capable of learning when to advise or not. The proposed algorithm is able to advise even when it does not have knowledge of the student's intended action and needs significantly less training time compared to previous learning approaches. Finally, in this article, we argue that learning to advise under a budget is an instance of a more generic learning problem: Constrained Exploitation Reinforcement Learning.
更多查看译文
关键词
machine learning, reinforcement learning, transfer learning, action advice, machine teaching
AI 理解论文
溯源树
样例
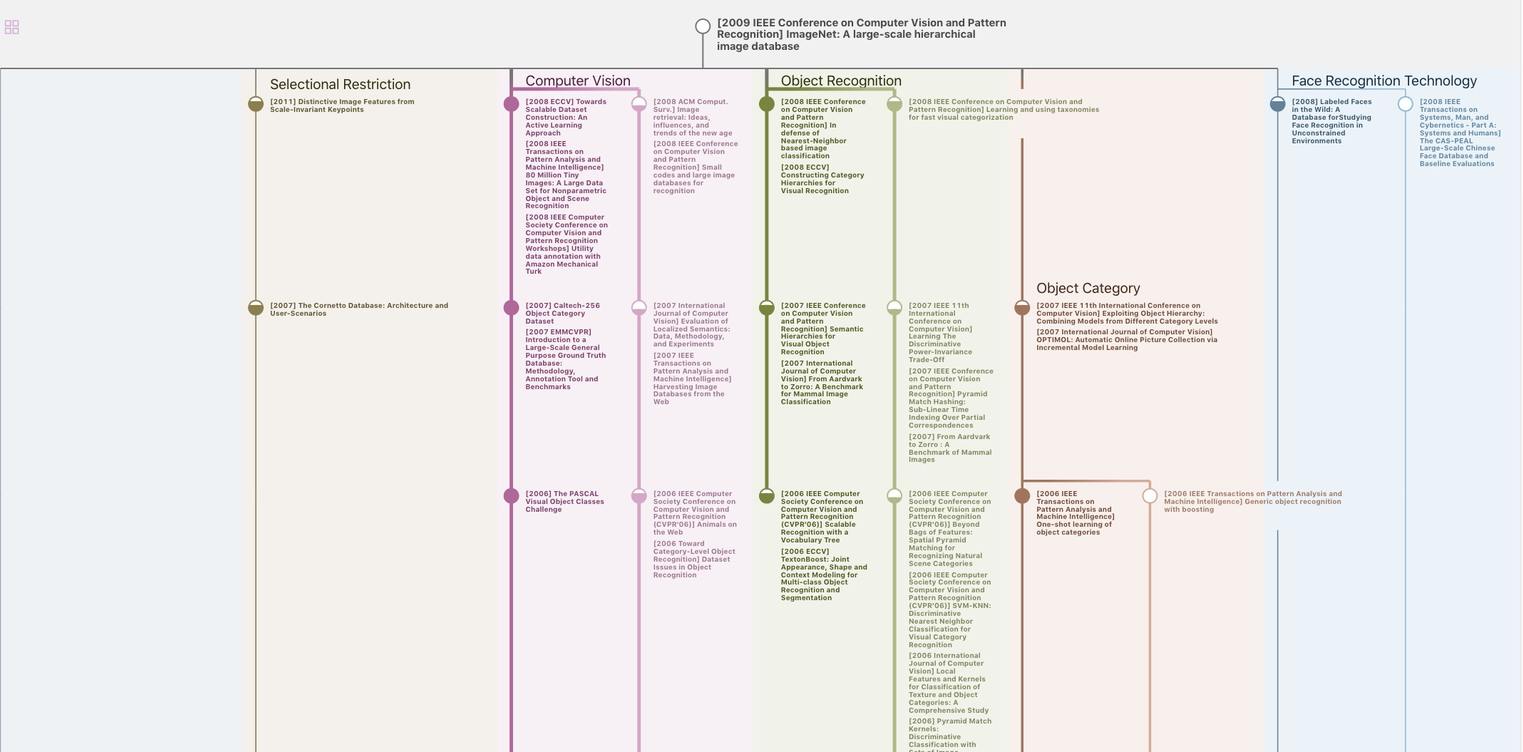
生成溯源树,研究论文发展脉络
Chat Paper
正在生成论文摘要