Algorithms for approximate subtropical matrix factorization
Data Mining and Knowledge Discovery(2018)
摘要
Matrix factorization methods are important tools in data mining and analysis. They can be used for many tasks, ranging from dimensionality reduction to visualization. In this paper we concentrate on the use of matrix factorizations for finding patterns from the data. Rather than using the standard algebra—and the summation of the rank-1 components to build the approximation of the original matrix—we use the subtropical algebra, which is an algebra over the nonnegative real values with the summation replaced by the maximum operator. Subtropical matrix factorizations allow “winner-takes-it-all” interpretations of the rank-1 components, revealing different structure than the normal (nonnegative) factorizations. We study the complexity and sparsity of the factorizations, and present a framework for finding low-rank subtropical factorizations. We present two specific algorithms, called Capricorn and Cancer, that are part of our framework. They can be used with data that has been corrupted with different types of noise, and with different error metrics, including the sum-of-absolute differences, Frobenius norm, and Jensen–Shannon divergence. Our experiments show that the algorithms perform well on data that has subtropical structure, and that they can find factorizations that are both sparse and easy to interpret.
更多查看译文
关键词
Tropical algebra,Max-times algebra,Matrix factorizations,Data mining
AI 理解论文
溯源树
样例
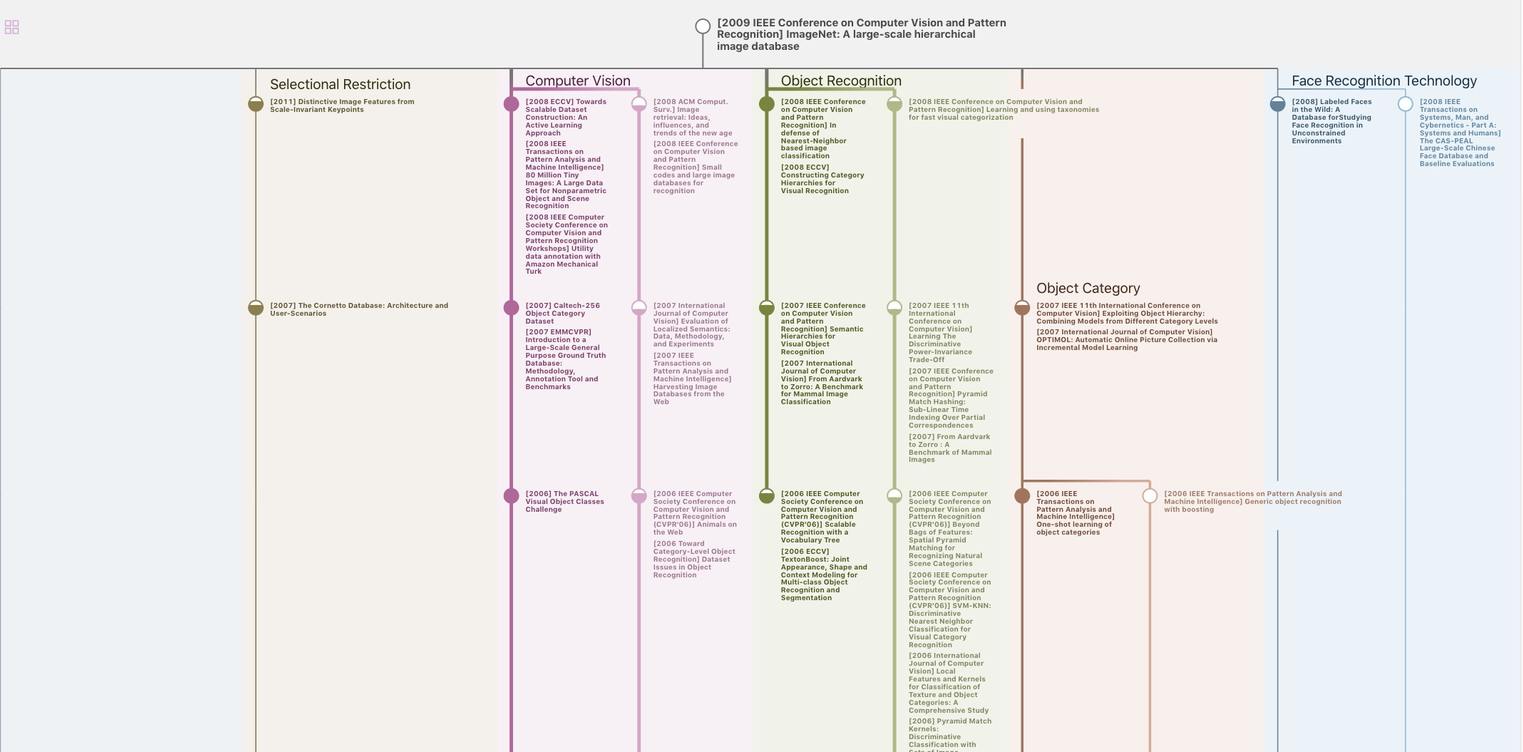
生成溯源树,研究论文发展脉络
Chat Paper
正在生成论文摘要