Structure-Preserving Image Super-resolution via Contextualized Multi-task Learning.
IEEE Trans. Multimedia(2017)
摘要
Single-image super-resolution (SR), which refers to reconstructing a higher resolution image from the observed lowresolution (LR) image, has received substantial attention due to its tremendous application potentials. Despite the breakthroughs of recently proposed SR methods using convolutional neural networks, their generated results usually lack of preserving structural (high-frequency) details. In this paper, regarding global boundary context and residual context as complimentary information for enhancing structural details in image restoration, we develop a contextualized multitask learning framework to address the SR problem. Specifically, our method first extracts convolutional features from the input LR image and applies one deconvolutional module to interpolate the LR feature maps in a content-adaptive way. Then, the resulting feature maps are fed into two branched subnetworks. On several standard benchmarks (e.g., Set5, Set14, and BSD200), our extensive evaluations demonstrate the effectiveness of our SR method on achieving both higher restoration quality and computational efficiency compared with several state-of-the-art SR approaches.
更多查看译文
关键词
Convolutional neural networks,Machine learning,Feature extraction,Image resolution,Multimedia computing,Training,Context,Image quality
AI 理解论文
溯源树
样例
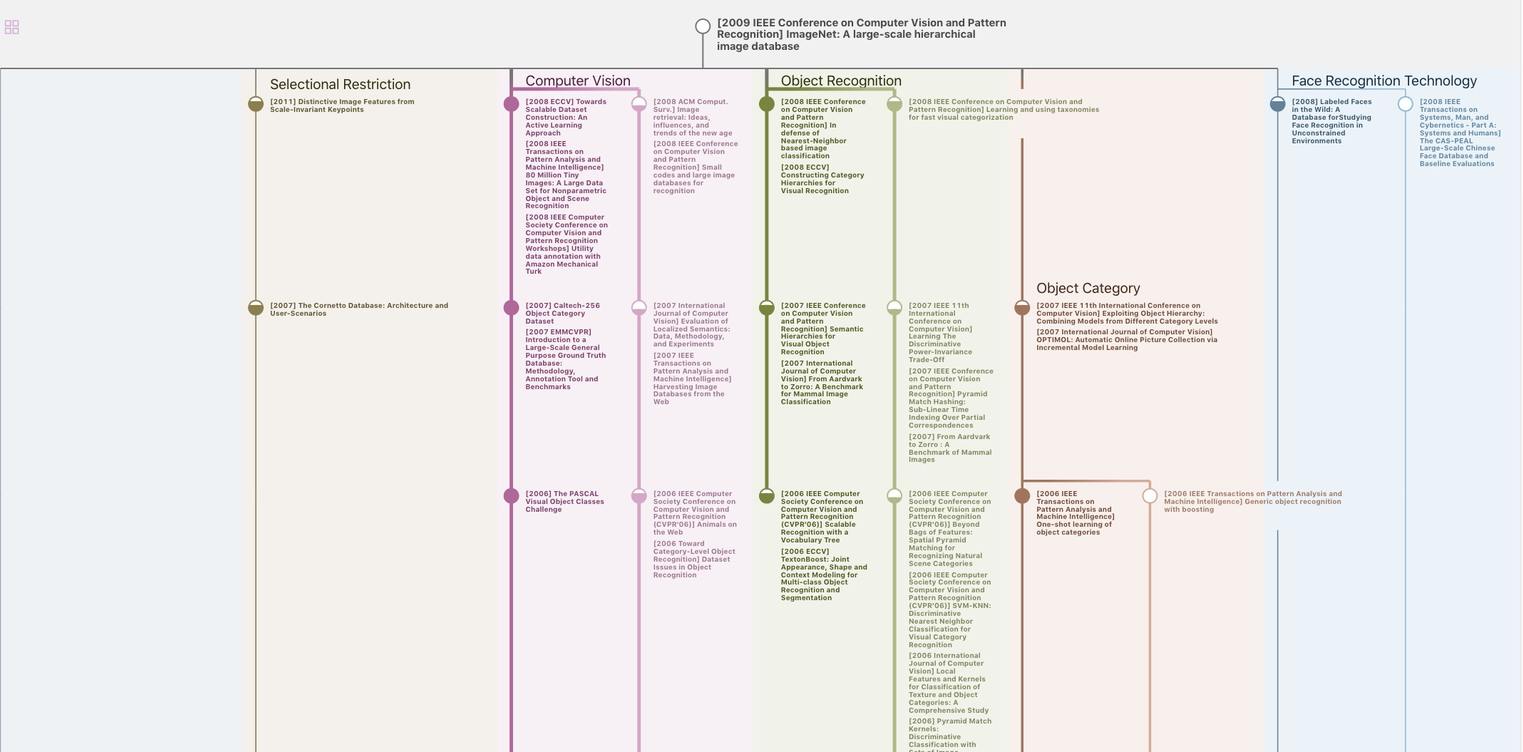
生成溯源树,研究论文发展脉络
Chat Paper
正在生成论文摘要