Initialising kernel adaptive filters via probabilistic inference
2017 22nd International Conference on Digital Signal Processing (DSP)(2017)
摘要
We present a probabilistic framework for both (i) determining the initial settings of kernel adaptive filters (KAFs) and (ii) constructing fully-adaptive KAFs whereby in addition to weights and dictionaries, kernel parameters are learnt sequentially. This is achieved by formulating the estimator as a probabilistic model and defining dedicated prior distributions over the kernel parameters, weights and dictionary, enforcing desired properties such as sparsity. The model can then be trained using a subset of data to initialise standard KAFs or updated sequentially each time a new observation becomes available. Due to the nonlinear/non-Gaussian properties of the model, learning and inference is achieved using gradient-based maximum-aposteriori optimisation and Markov chain Monte Carlo methods, and can be confidently used to compute predictions. The proposed framework was validated on nonlinear time series of both synthetic and real-world nature, where it outperformed standard KAFs in terms of mean square error and the sparsity of the learnt dictionaries.
更多查看译文
关键词
nonlinear time series,probabilistic inference,KAF,gradient-based maximum-aposteriori optimisation,dictionary learning,kernel adaptive filters,nonGaussian properties,Markov chain Monte Carlo,probabilistic model
AI 理解论文
溯源树
样例
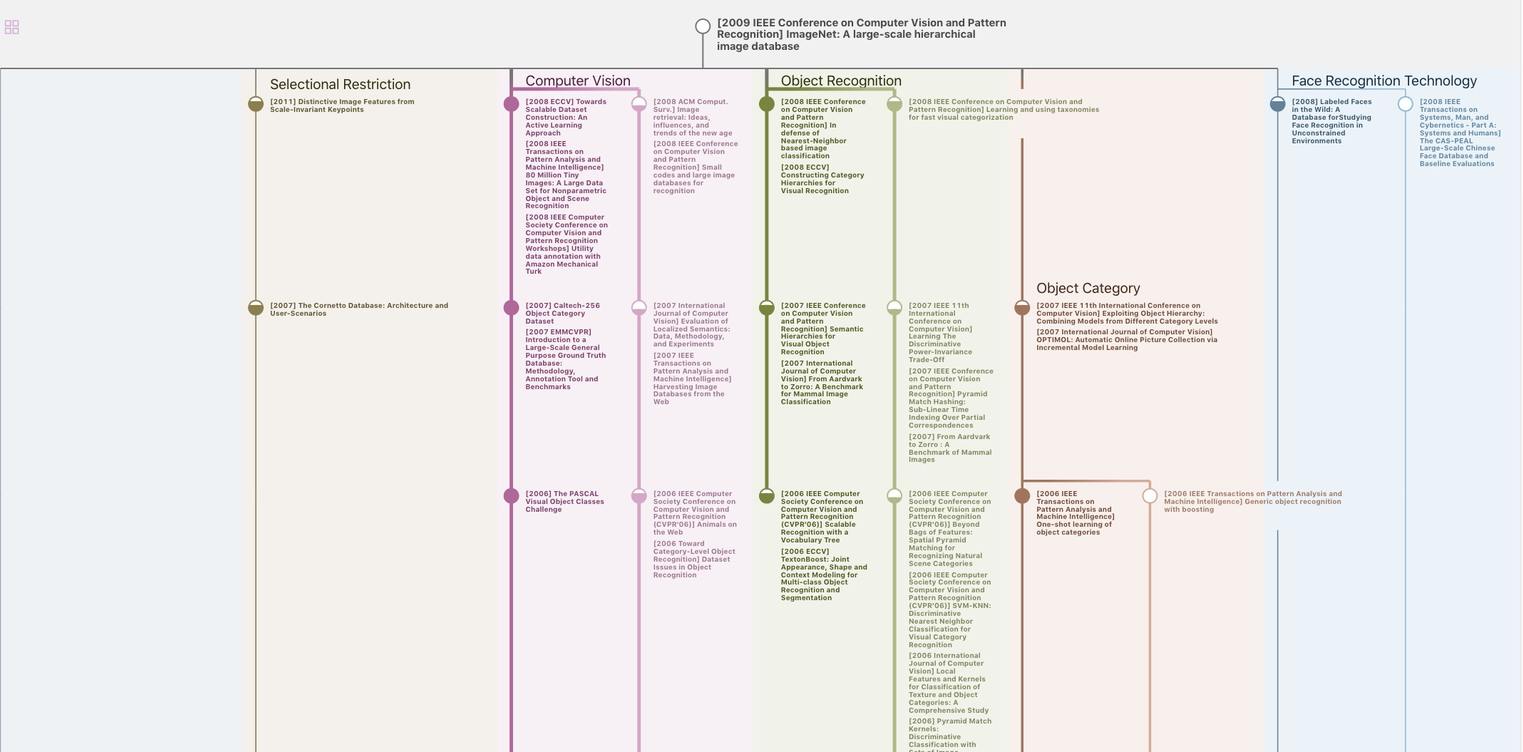
生成溯源树,研究论文发展脉络
Chat Paper
正在生成论文摘要