Guest Editorial: Large-Scale Multimedia Data Retrieval, Classification, and Understanding.
IEEE Trans. Multimedia(2017)
摘要
The papers in this special section focus on multimedia data retrieval and classification via large-scale systems. Today, large collections of multimedia data are explosively created in different fields and have attracted increasing interest in the multimedia research area. Large-scale multimedia data provide great unprecedented opportunities to address many challenging research problems, e.g., enabling generic visual classification to bridge the well-known semantic gap by exploring large-scale data, offering a promising possibility for in-depth multimedia understanding, as well as discerning patterns and making better decisions by analyzing the large pool of data. Therefore, the techniques for large-scale multimedia retrieval, classification, and understanding are highly desired. Simultaneously, the explosion of multimedia data puts urgent needs for more sophisticated and robust models and algorithms to retrieve, classify, and understand these data. Another interesting challenge is, how can the traditional machine learning algorithms be scaled up to millions and even billions of items with thousands of dimensionalities? This motivated the community to design parallel and distributed machine learning platforms, exploiting GPUs as well as developing practical algorithms. Besides, it is also important to exploit the commonalities and differences between different tasks, e.g., image retrieval and classification have much in common while different indexing methods evolve in a mutually supporting way.
更多查看译文
AI 理解论文
溯源树
样例
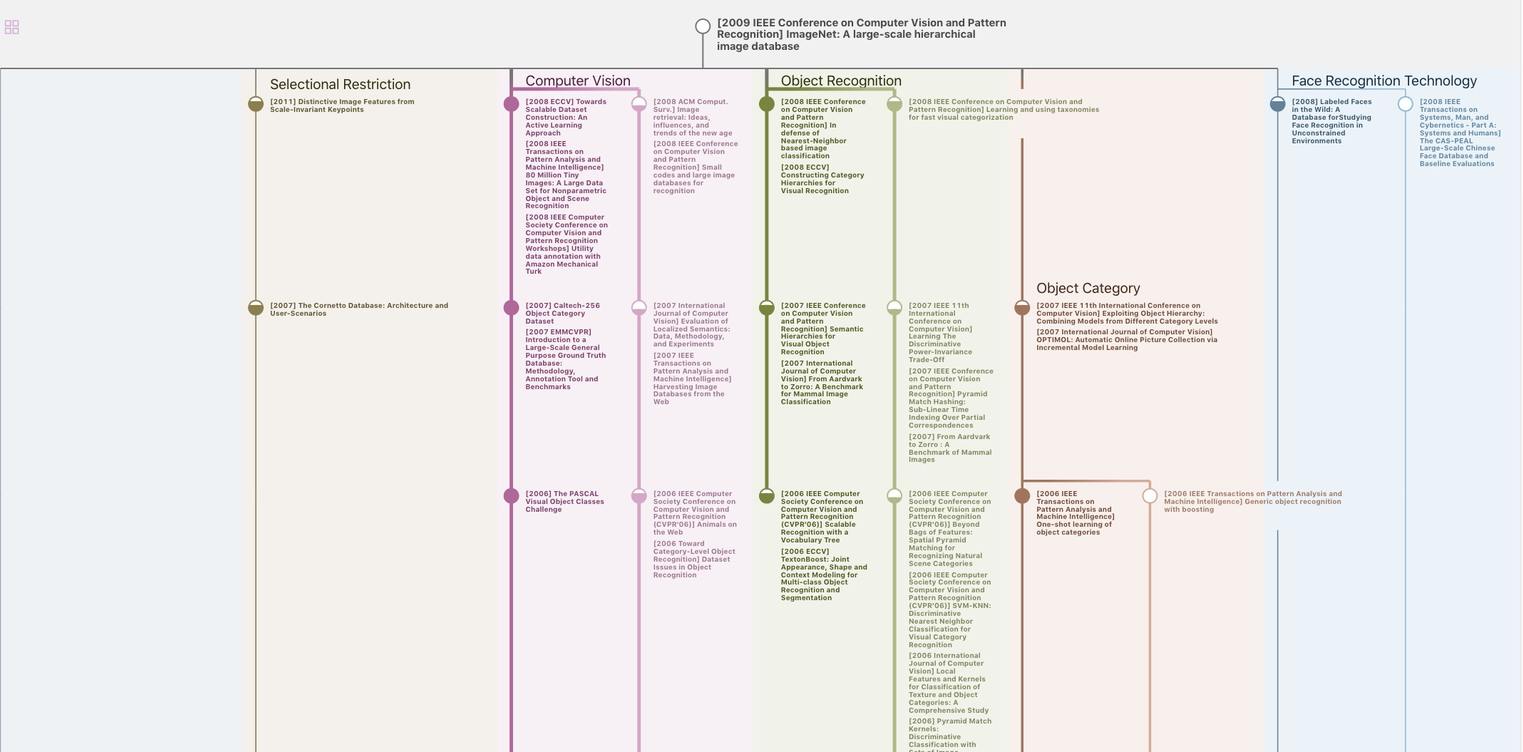
生成溯源树,研究论文发展脉络
Chat Paper
正在生成论文摘要