A Guidance Of Data Stream Characterization For Meta-Learning
INTELLIGENT DATA ANALYSIS(2017)
摘要
The problem of selecting learning algorithms has been studied by the meta-learning community for more than two decades. One of the most important task for the success of a meta-learning system is gathering data about the learning process. This data is used to induce a (meta) model able to map characteristics extracted from different data sets to the performance of learning algorithms on these data sets. These systems are built under the assumption that the data are generated by a stationary distribution, i.e., a learning algorithm will perform similarly for new data from the same problem. However, many applications generate data whose characteristics can change over time. Therefore, a suitable bias at a given time may become inappropriate at another time. Although meta-learning has been used to continuously select a learning algorithm in data streams, data characterization has received less attention in this context. In this study, we provide a set of guidelines to support the proposal of characteristics able to describe non-stationary data over time. This guidance considers both the order of arrival of the examples and the type of variables involved in the base-level learning. In addition, we analyze the influence of characteristics regarding their dependence on data morphology. Experimental results using real data streams showed the effectiveness of the proposed data characterization general scheme to support algorithm selection by meta-learning systems. Moreover, the dependent metafeatures provided crucial information for the success of some meta-models.
更多查看译文
关键词
Feature extraction, data streams, algorithm selection
AI 理解论文
溯源树
样例
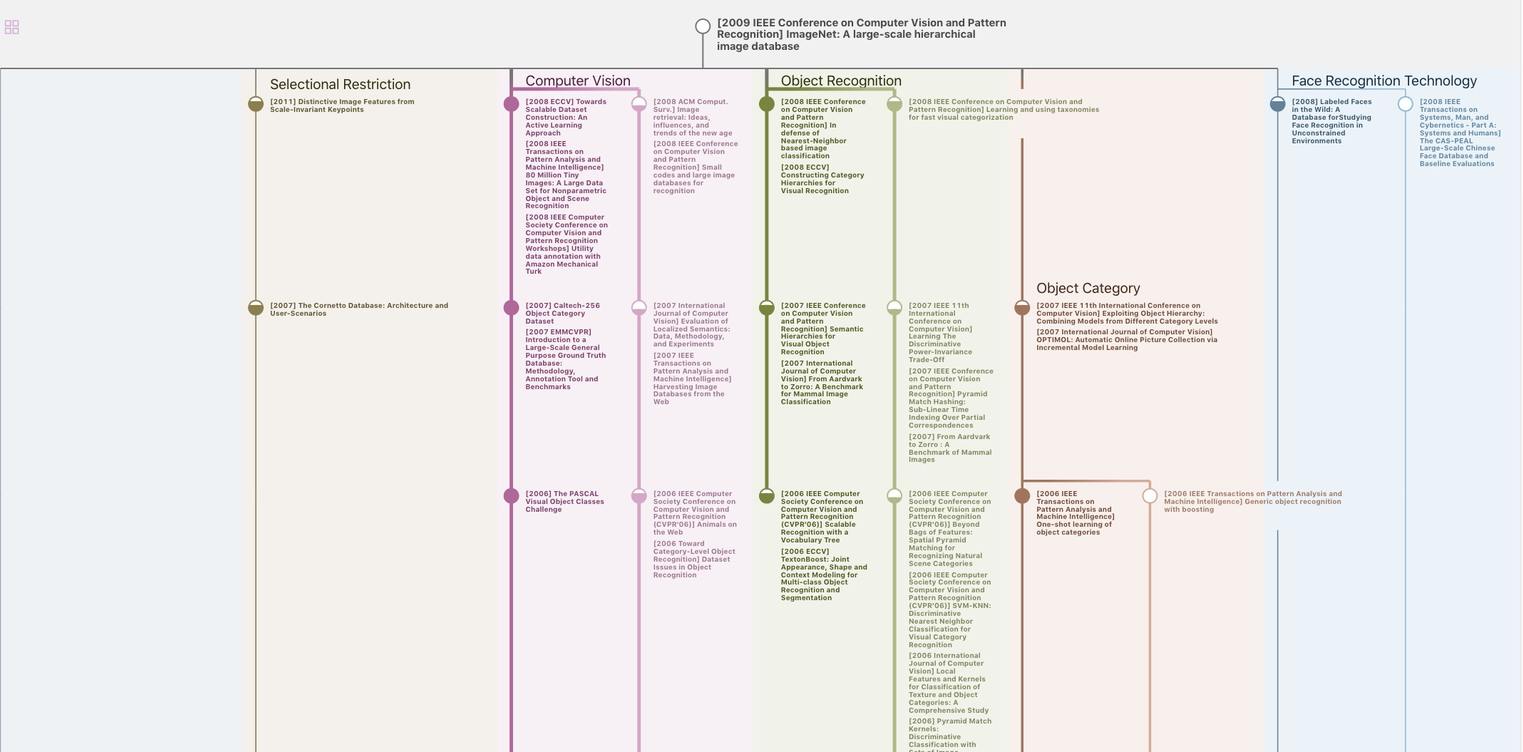
生成溯源树,研究论文发展脉络
Chat Paper
正在生成论文摘要