A space decomposition scheme for maximum eigenvalue functions and its applications
Mathematical Methods of Operations Research(2017)
摘要
In this paper, we study nonlinear optimization problems involving eigenvalues of symmetric matrices. One of the difficulties in solving these problems is that the eigenvalue functions are not differentiable when the multiplicity of the function is not one. We apply the 𝒰 -Lagrangian theory to analyze the largest eigenvalue function of a convex matrix-valued mapping which extends the corresponding results for linear mapping in the literature. We also provides the formula of first-and second-order derivatives of the 𝒰 -Lagrangian under mild assumptions. These theoretical results provide us new second-order information about the largest eigenvalue function along a suitable smooth manifold, and leads to a new algorithmic framework for analyzing the underlying optimization problem.
更多查看译文
关键词
Nonsmooth optimization,Eigenvalue optimization,Matrix-convex,Semidefinite programming,𝒱𝒰 -decomposition,𝒰 -Lagrangian,Smooth manifold,Second-order derivative,Bilinear matrix inequality
AI 理解论文
溯源树
样例
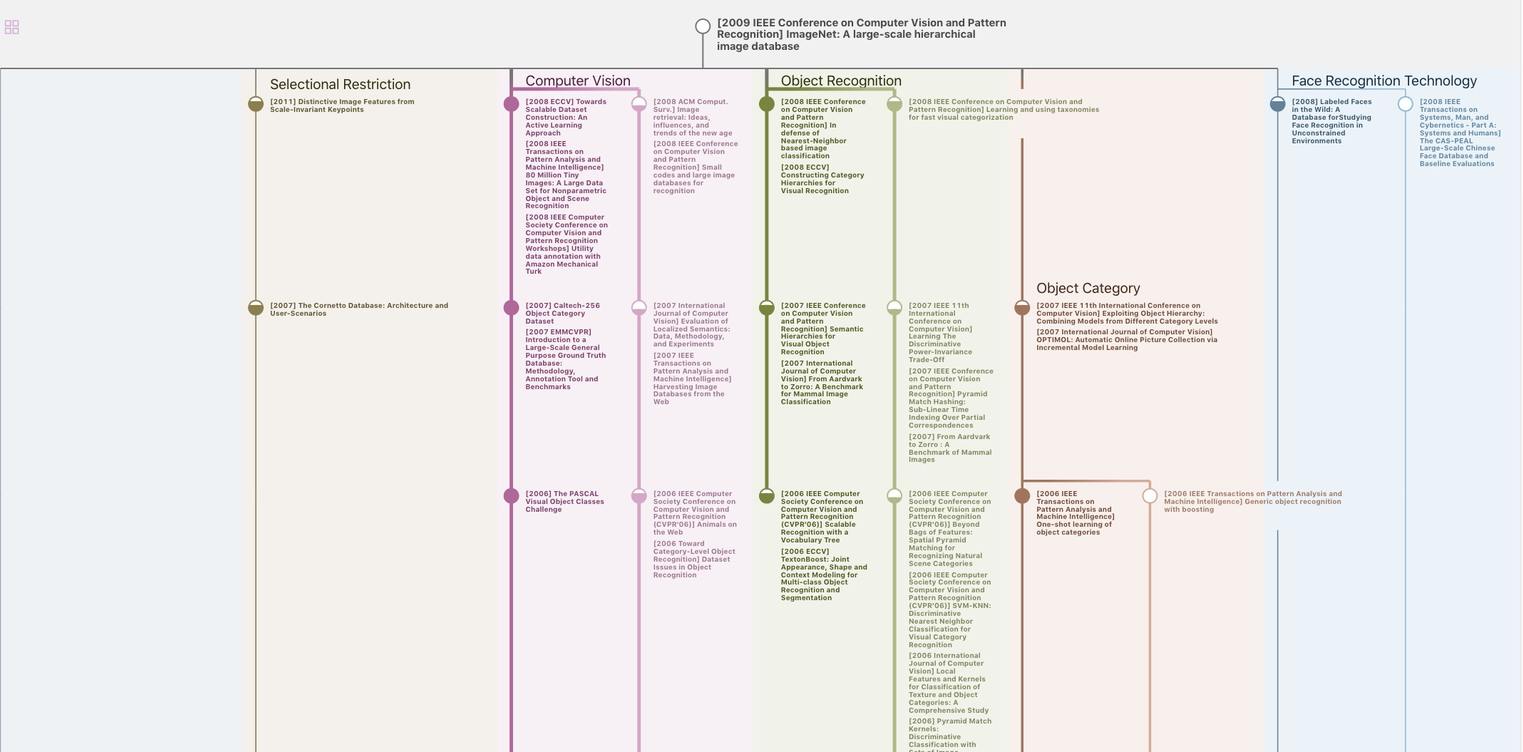
生成溯源树,研究论文发展脉络
Chat Paper
正在生成论文摘要