Unsupervised detection of density changes through principal component analysis for lung lesion classification
Multimedia Tools Appl.(2017)
摘要
Lung cancer remains one of the most common cancers worldwide. Temporal evaluation is a useful tool for analyzing the malignant behavior of a lesion during treatment or that of indeterminate lesions which may be benign. Thereby, this work proposes a methodology for analysis, quantification and visualization of unsupervised changes in lung lesions, through principal component analysis. From change regions, we extracted texture features for lesion classification as benign or malignant. To reach this purpose, two databases with distinct behavior were used, one of which concerning malign under treatment and another indeterminate, but likely benign, lesions. The results have shown that the lesion’s density changes in a public database of malignant lesions under treatment were greater than the private database of benign lung nodules. From the texture analysis of the regions where the density changes occurred, we were able to discriminate lung lesions with an accuracy of 98.41 %, showing that these changes could point out the nature of the lesion. Other contribution was visualization of changes occurring in the lesions over time. Besides, we quantified these changes and analyzed the entire set through volumetry, the most commonly used technique to evaluate progression of lung lesions.
更多查看译文
关键词
Medical image, Lung lesion, Detection of changes in tissues, Principal component analysis, Temporal analysis and evaluation
AI 理解论文
溯源树
样例
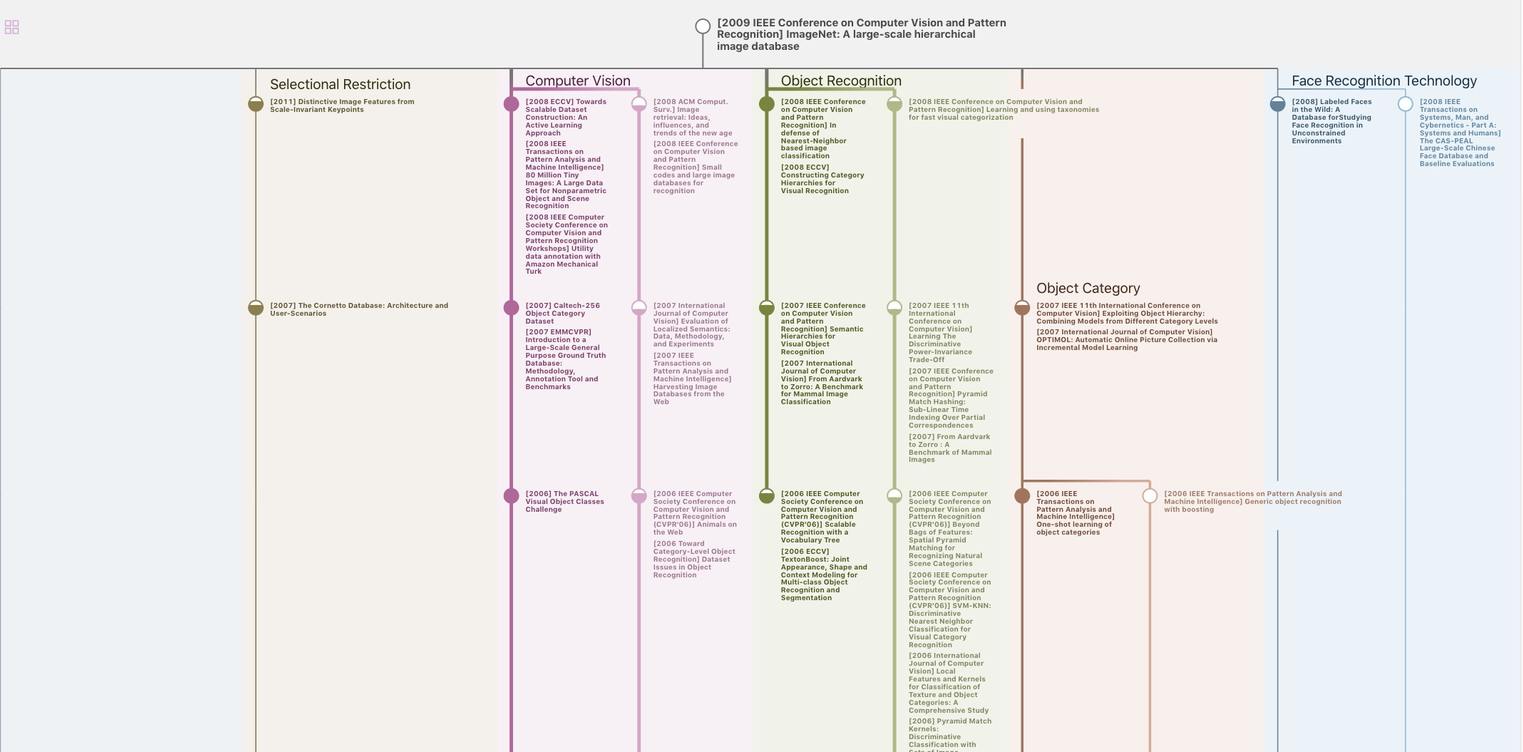
生成溯源树,研究论文发展脉络
Chat Paper
正在生成论文摘要