RUSH!: Targeted Time-limited Coupons via Purchase Forecasts
KDD(2017)
摘要
Time-limited promotions that exploit consumers' sense of urgency to boost sales account for billions of dollars in consumer spending each year. However, it is challenging to discover the right timing and duration of a promotion to increase its chances of being redeemed. In this work, we consider the problem of delivering time-limited discount coupons, where we partner with a large national bank functioning as a commission-based third-party coupon provider. Specifically, we use large-scale anonymized transaction records to model consumer spending and forecast future purchases, based on which we generate data-driven, personalized coupons. Our proposed model RUSH! (1) predicts {both the time and category} of the next event; (2) captures correlations between purchases in different categories (such as shopping triggering dining purchases); (3) incorporates temporal dynamics of purchase behavior (such as increased spending on weekends); (4) is composed of additive factors that are easily interpretable; and finally (5) scales linearly to millions of transactions. We design a cost-benefit framework that facilitates systematic evaluation in terms of our application, and show that RUSH! provides higher expected value than various baselines that do not jointly model time and category information.
更多查看译文
关键词
transaction data,targeted promotions,point processes,cost-benefit
AI 理解论文
溯源树
样例
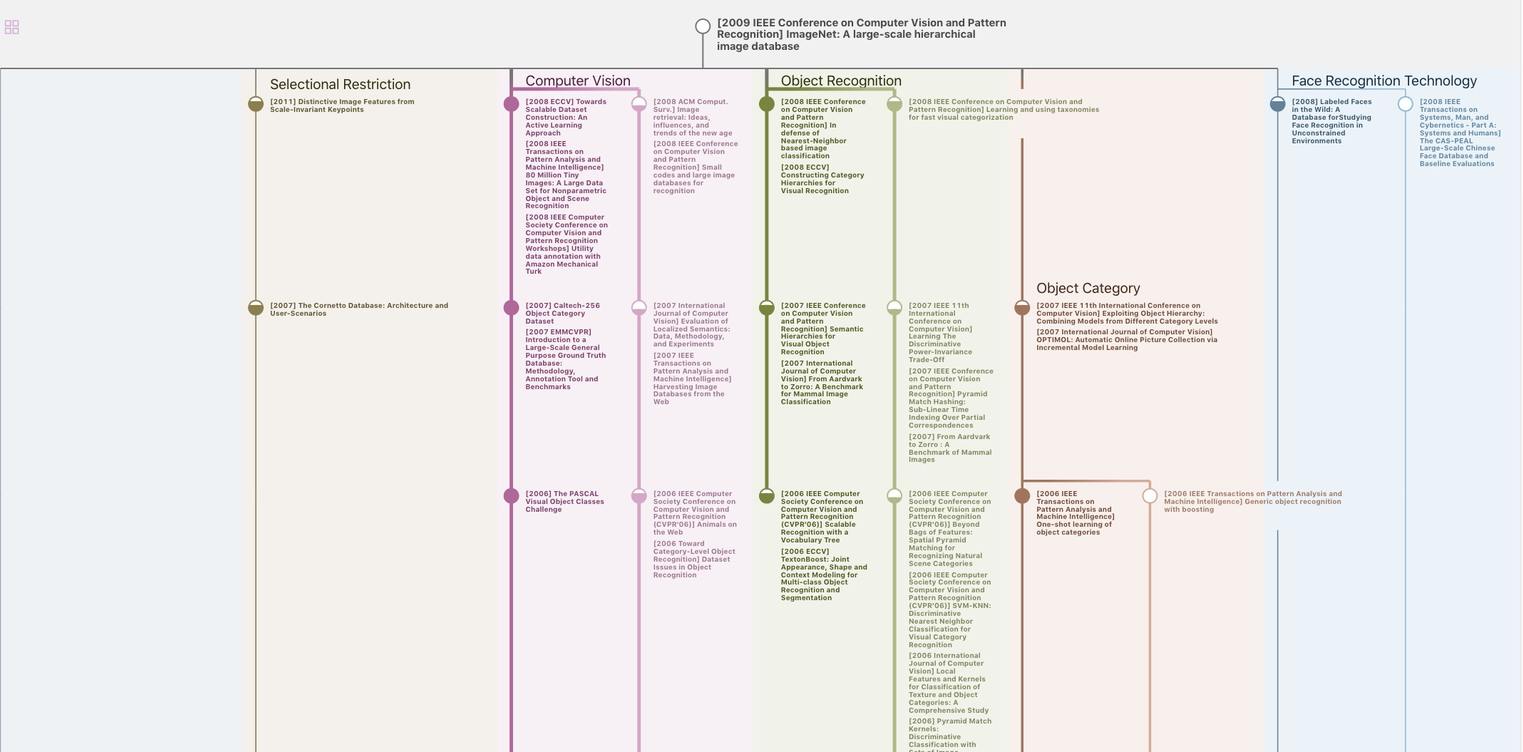
生成溯源树,研究论文发展脉络
Chat Paper
正在生成论文摘要