Inductive Semi-supervised Multi-Label Learning with Co-Training
KDD(2017)
摘要
In multi-label learning, each training example is associated with multiple class labels and the task is to learn a mapping from the feature space to the power set of label space. It is generally demanding and time-consuming to obtain labels for training examples, especially for multi-label learning task where a number of class labels need to be annotated for the instance. To circumvent this difficulty, semi-supervised multi-label learning aims to exploit the readily-available unlabeled data to help build multi-label predictive model. Nonetheless, most semi-supervised solutions to multi-label learning work under transductive setting, which only focus on making predictions on existing unlabeled data and cannot generalize to unseen instances. In this paper, a novel approach named COINS is proposed to learning from labeled and unlabeled data by adapting the well-known co-training strategy which naturally works under inductive setting. In each co-training round, a dichotomy over the feature space is learned by maximizing the diversity between the two classifiers induced on either dichotomized feature subset. After that, pairwise ranking predictions on unlabeled data are communicated between either classifier for model refinement. Extensive experiments on a number of benchmark data sets show that COINS performs favorably against state-of-the-art multi-label learning approaches.
更多查看译文
AI 理解论文
溯源树
样例
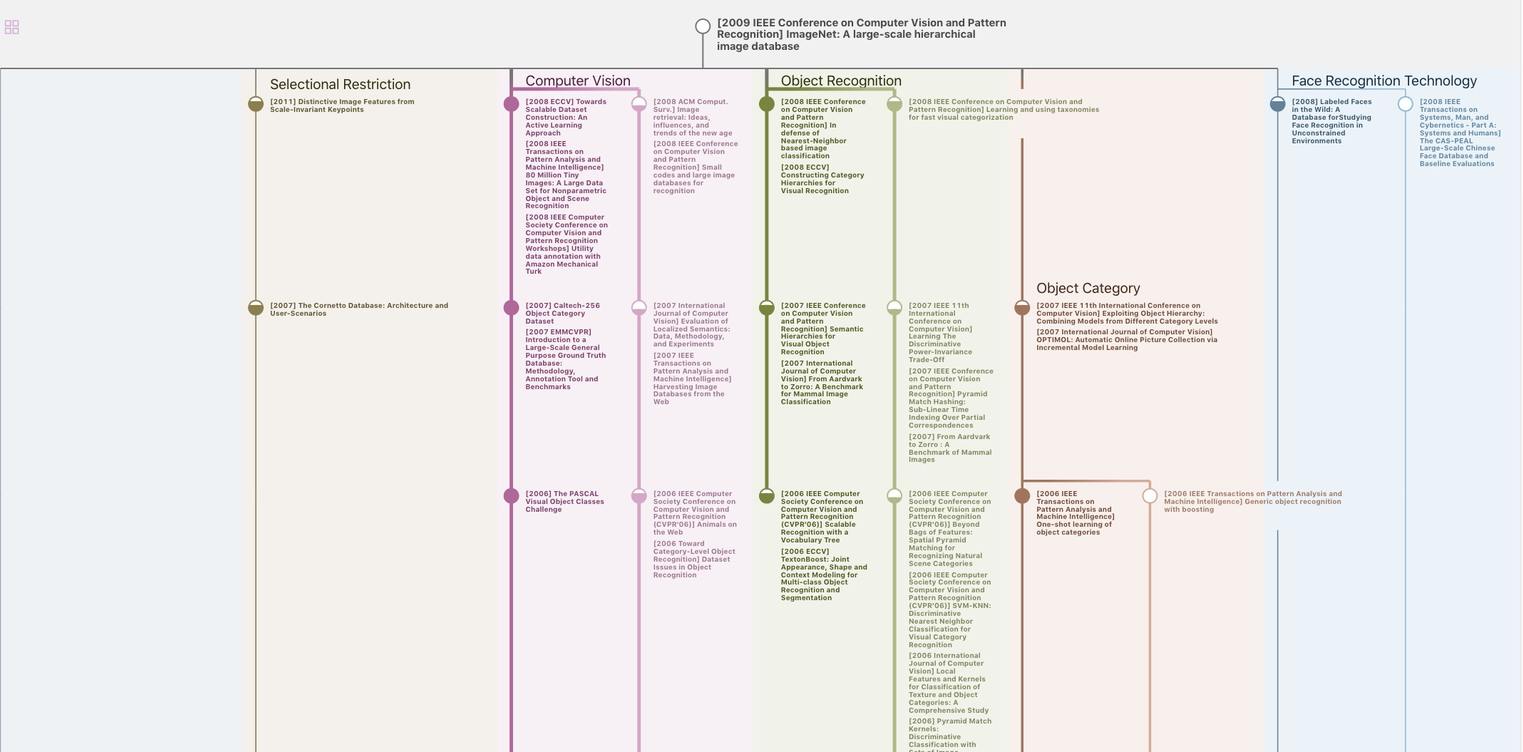
生成溯源树,研究论文发展脉络
Chat Paper
正在生成论文摘要