End-node Fingerprinting for Malware Detection on HTTPS Data
ARES(2017)
摘要
One of the current challenges in network intrusion detection research is the malware communicating over HTTPS protocol. Usually the task is to detect infected end-nodes with this type of malware by monitoring network traffic. The challenge lies in a very limited number of weak features that can be extracted from the network traffic capture of encrypted HTTP communication. This paper suggests a novel fingerprinting method that addresses this problem by building a higher-level end-node representation on top of the weak features. Conducted large-scale experiments on real network data show superior performance of the proposed method over the state-of-the-art solution in terms of both a lower number of produced false alarms (precision) and a higher number of detected infections (recall).
更多查看译文
关键词
HTTPS data, Malware detection, Supervised learning
AI 理解论文
溯源树
样例
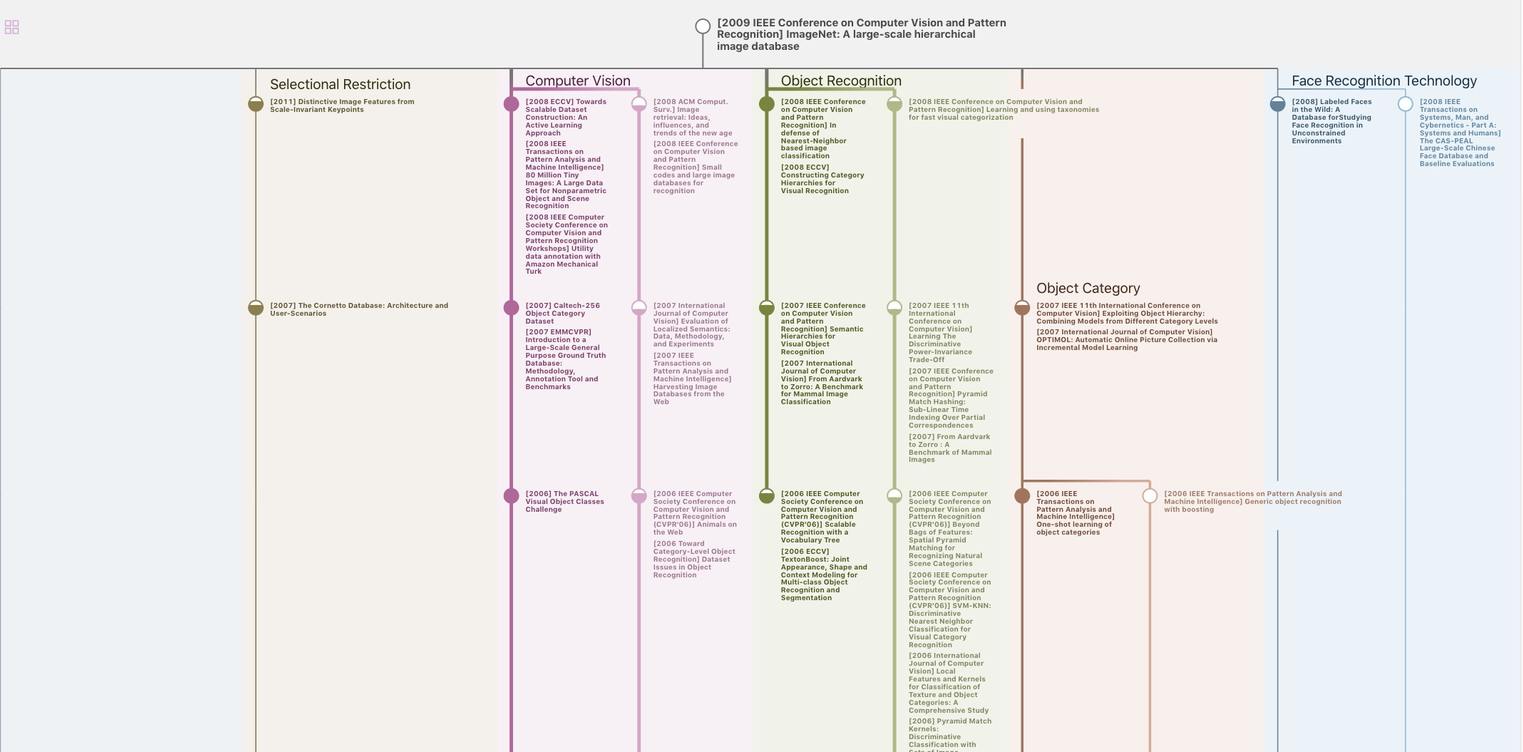
生成溯源树,研究论文发展脉络
Chat Paper
正在生成论文摘要