You are what apps you use: Transfer Learning for Personalized Content and Ad Recommendation
RecSys(2017)
摘要
Cold start is always a key challenge for building real-life recommendation systems. Thanks to the ever-growing multi-modal data in the mobile Internet age and the latest deep learning techniques, transfer-learning based cross-domain recommendation starts to play a crucial role in tackling the cold start problem and to provide \"warm-start\" recommendation for new users. At Cheetah Mobile, we apply transfer learning to build personalized recommendation systems for both advertisement and content scenarios, serving 600+ millions monthly active mobile users. In particular, we leveraged the app install & usage and many other mobile data, built a Unified User Profile (UUP) by using transfer learning and deep learning, and developed cross-domain personalized Ad and news recommendation. Our approaches enable us to solve the cold start problem with close to full coverage of our user base while yielding significant CTR increase and better user experience.
更多查看译文
关键词
User Profile, Personalized Recommendation, Transfer Learning
AI 理解论文
溯源树
样例
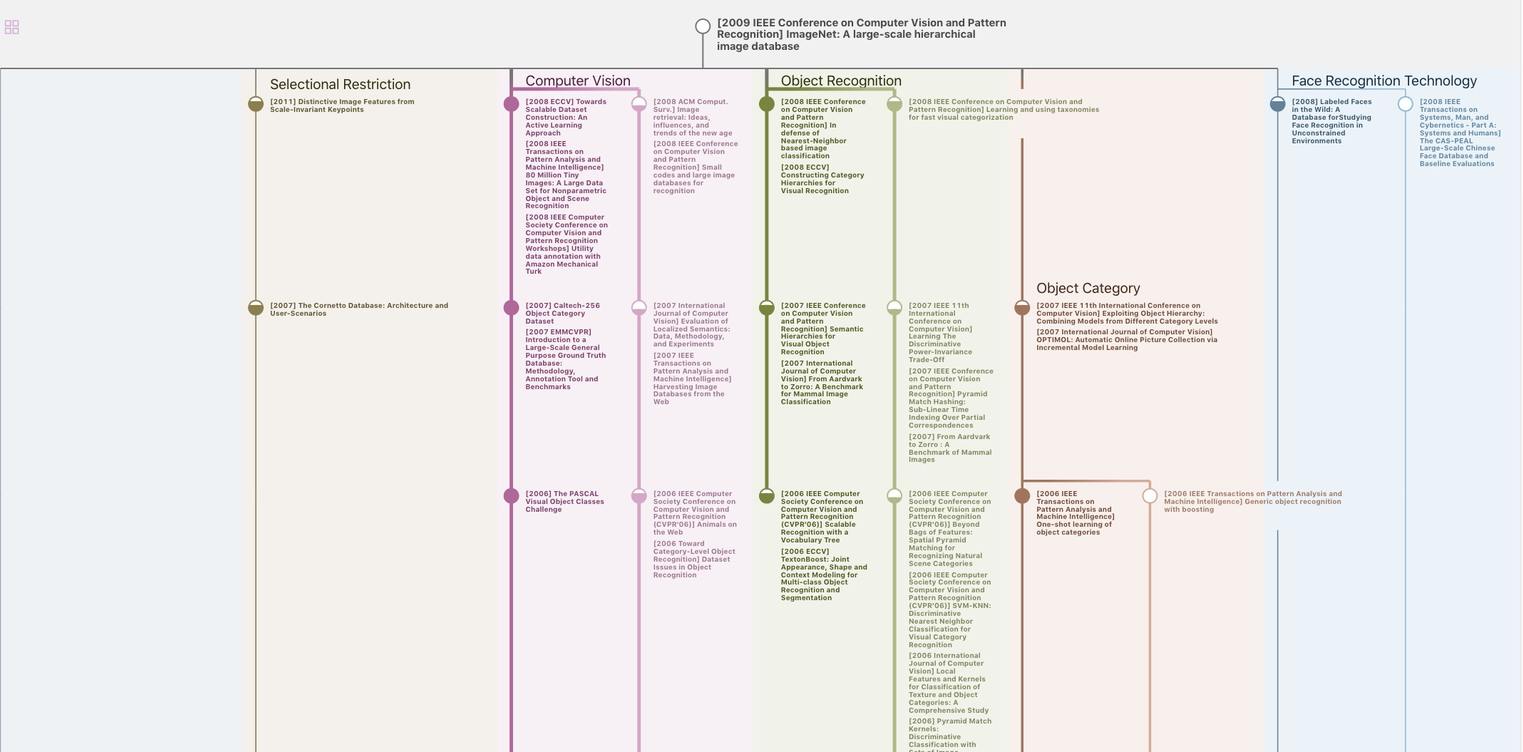
生成溯源树,研究论文发展脉络
Chat Paper
正在生成论文摘要